The Entropy Economy and the Kolmogorov Learning Cycle: Leveraging the intersection of Machine Learning and Algorithmic Information Theory to jointly optimize energy and learning
PHYSICA D-NONLINEAR PHENOMENA(2024)
摘要
We augment the Kolmogorov Structure Function with energy cost and drive the concept of "Additive AI" where Machine Learning Models are created by traversing the Kolmogorov Structure function from low model complexity to high while seeking models achieving the Kolmogorov Minimum Sufficient Statistic with least energy cost. In this way, the intersection of Algorithmic Information Theory (AIT) with Machine Learning (ML) can enable optimization of the "Entropy Economy," where the precious resource of entropy flow is managed to jointly optimize computation, energy, and learning. In this paper we lay out the Kolmogorov Learning Cycle as a framework for this joint optimization and demonstrate the energy efficient machine learning algorithm Least Energy Usage Network (LEAN) as an example of how restraining complexity can reduce learning energy cost while maintaining performance. We motivate further directions for optimizing how AI models can be optimally learned and discuss additional opportunities to optimize where and when AI and machine learning models can be created to maximize learning while minimizing energy (and subsequently carbon costs) through the intersection of AIT and ML.
更多查看译文
关键词
Kolmogorov complexity,Kolmogorov structure function,Machine learning,Entropy Economy,Algorithmic Information Theory
AI 理解论文
溯源树
样例
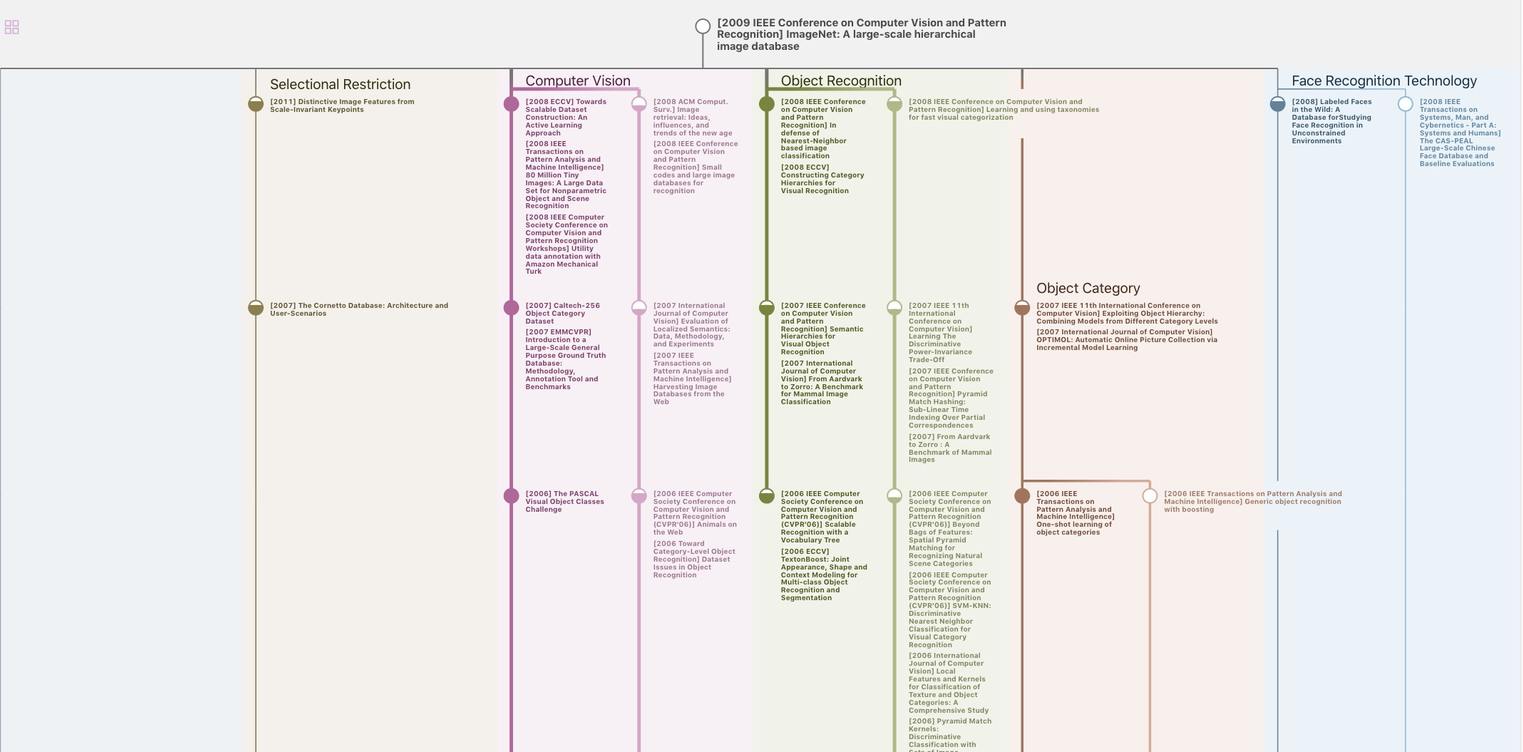
生成溯源树,研究论文发展脉络
Chat Paper
正在生成论文摘要