Remote sensing-based land use land cover classification for the Heuningnes Catchment, Cape Agulhas, South Africa
PHYSICS AND CHEMISTRY OF THE EARTH(2024)
摘要
The primary objective of this study was to evaluate the effectiveness of Sentinel 2 and machine-learning technique for classifying seasonal land use land cover (LULC) changes on an annual basis, in the Heuningnes Catchment in Cape Agulhas, South Africa. The study focused on July 2017, October 2017, March 2018, and July 2018, representing both dry and wet seasons within the Catchment. The study also assessed the rainfall and temperature variations and how they link with these short-term changes in LULC. The classification results revealed a consistent increase in the extent of bare rock and soil cover from October 2017 to July 2018. The wet seasons of July 2017 and July 2018 exhibited the highest percentage of vegetation cover. The overall accuracy of the SVM classification ranged between 55 % and 75 %, with the wet seasons demonstrating higher overall accuracies of 75 %. The performance of SVM was evaluated using kappa statistics, which indicated a moderate to substantial level of agreement ranging from 0.43 to 0.69. By employing machine-learning techniques and satellite data, this study contributes to a better understanding of LULC patterns and their implications for water security, sustainable development goals, and community livelihoods in the Heuningnes Catchment of Cape Agulhas, South Africa.
更多查看译文
关键词
Land management,Machine-learning techniques,Sentinel-2
AI 理解论文
溯源树
样例
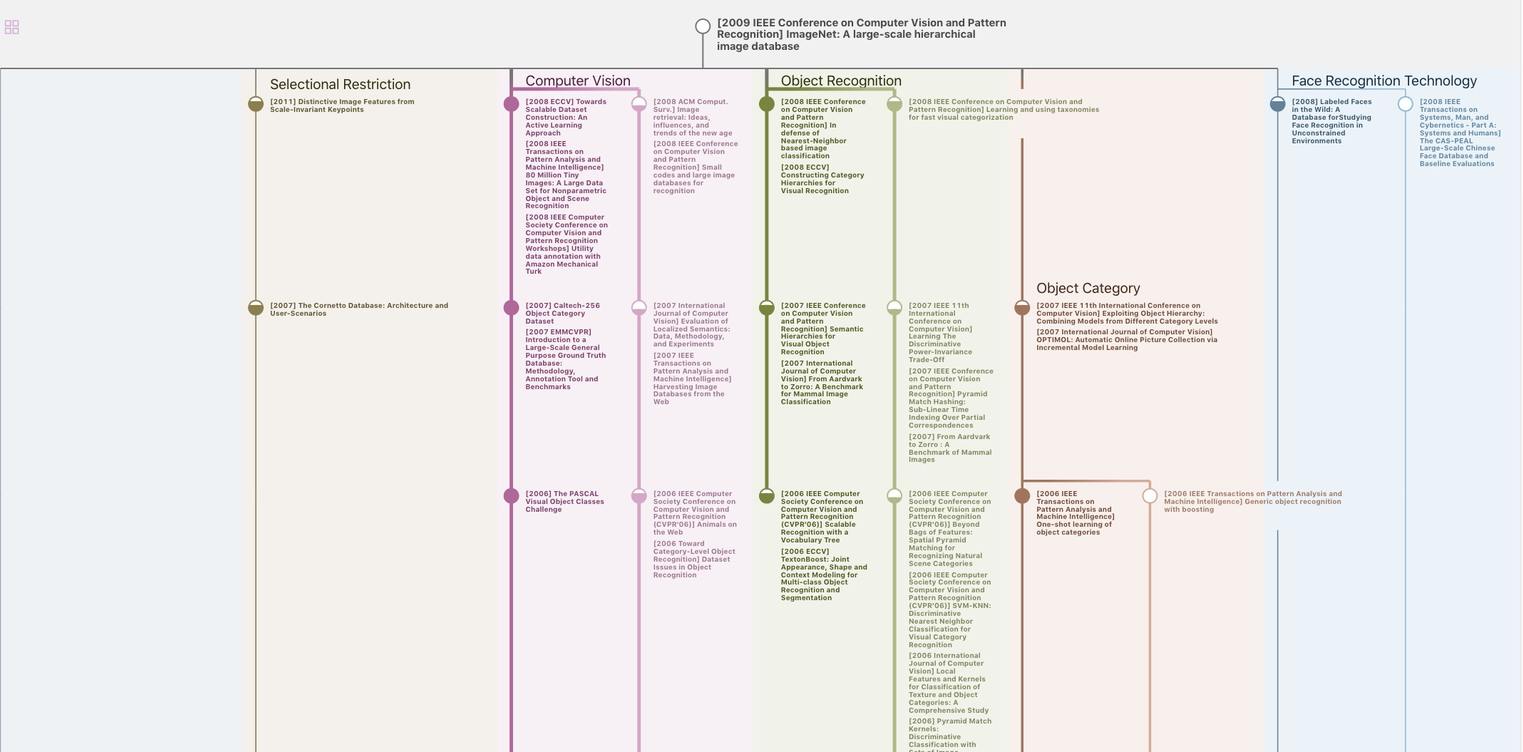
生成溯源树,研究论文发展脉络
Chat Paper
正在生成论文摘要