Machine learning-assisted FTIR spectra to predict freeze-drying curve of food
LWT-FOOD SCIENCE AND TECHNOLOGY(2024)
摘要
With the demand for improving freeze-drying (FD) process efficiency and protecting product characteristics, intelligent and robust analysis of process parameters for drawing effective FD curves has become the development direction of modern FD process. In this study, a prompt and applicable prediction model of FD parameters was designed by FTIR associated with chemometrics. By using spectral preprocessing and principal component analysis, 34 feature wavenumbers were extracted as input variables for modeling to quantify FD parameters. Among the 18 parameter prediction models, artificial neural network was adopted as the optimum model for the temperature and time of pre-freezing and desorption stages (R2 = 0.91, 0.83, 0.92, 0.84, RMSE = 0.12, 0.13, 0.08, 0.10), and random forest was confirmed as the best model for the parameters of sublimation stage (R2 = 0.88, 0.77, RMSE = 0.13, 0.16). According to the model prediction, random samples were selected for verification that the experimental results were close to 96% agreement with the model output.
更多查看译文
关键词
Mid-infrared spectroscopy,Freeze drying curve,Principal component analysis,Machine learning,Quantification
AI 理解论文
溯源树
样例
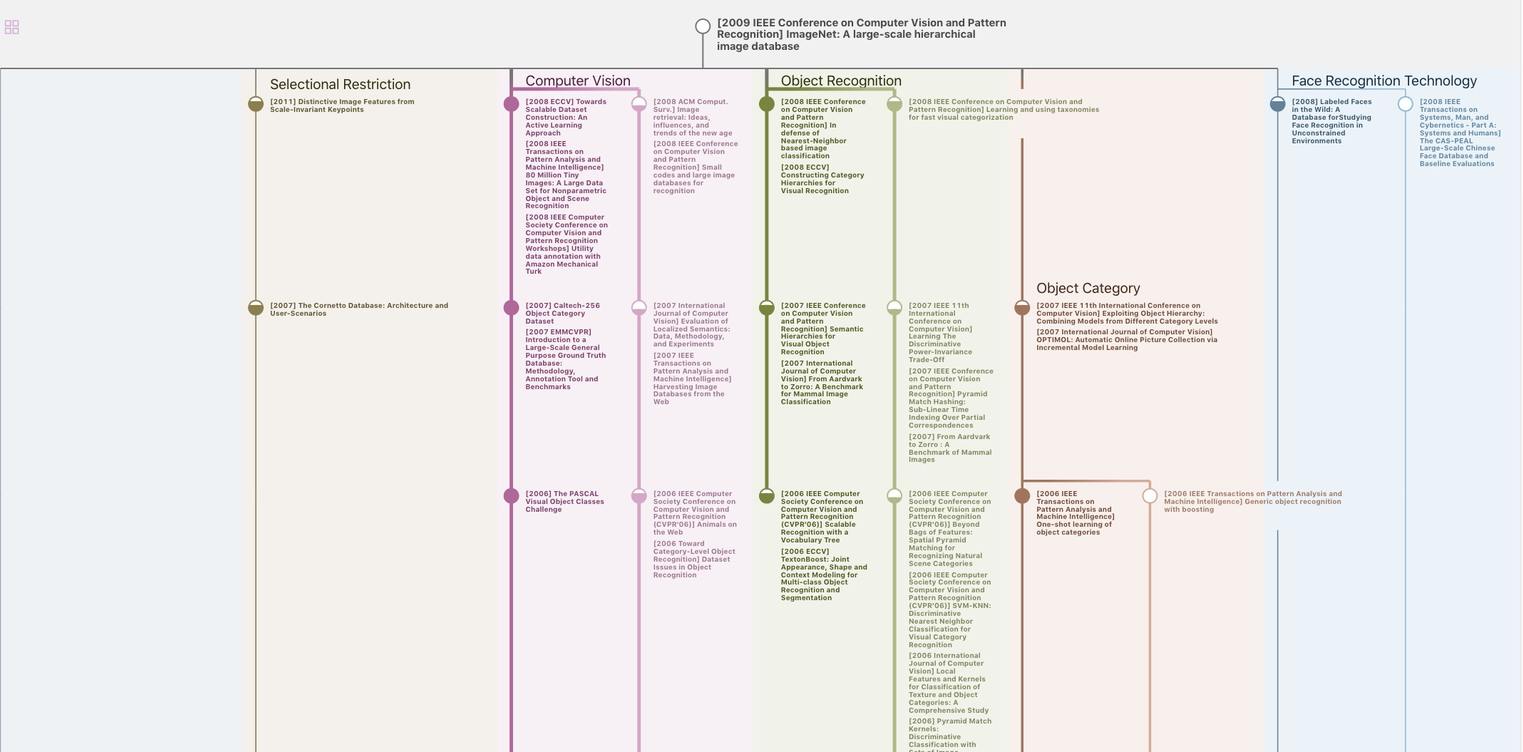
生成溯源树,研究论文发展脉络
Chat Paper
正在生成论文摘要