Cooperative Markov Decision Process model for human-machine co-adaptation in robot-assisted rehabilitation
KNOWLEDGE-BASED SYSTEMS(2024)
摘要
Human-machine interaction is a critical component in robotic rehabilitation systems. A mutual learning strategy involving both machine- and human -oriented learning has shown improvements in learning efficiency and receptiveness. Despite these advancements, a theoretical framework that encompasses high-level human responses during robot -assisted rehabilitation is still needed. This paper introduces a novel human-machine interface that uses a Co -adaptive Markov Decision Process (CaMDP) model based on cooperative multi -agent reinforcement learning. The CaMDP model effectively measures user adaptation to machines, treating the entire rehabilitation process as a collaborative learning experience. It quantifies learning rates at a higher system abstraction level. Policy Iteration in Reinforcement Learning is employed for the cooperative adjustment of Policy Improvement between the human and machine. Simulation studies demonstrate that the proposed new Policy Improvement approach has great potential to address non-stationarity issues and significantly reduce the switching frequency of patients - from 16.6% to 2% in a sample of 120,000 cases. The CaMDP model provides valuable insights into rehabilitation effect prediction and risk avoidance through dual -agent simulation, thereby enhancing the overall performance of the rehabilitation process.
更多查看译文
关键词
Reinforcement learning,Cooperative Markov Decision Processes,Multi-agent reinforcement learning,Model-based reinforcement learning,Rehabilitation engineering
AI 理解论文
溯源树
样例
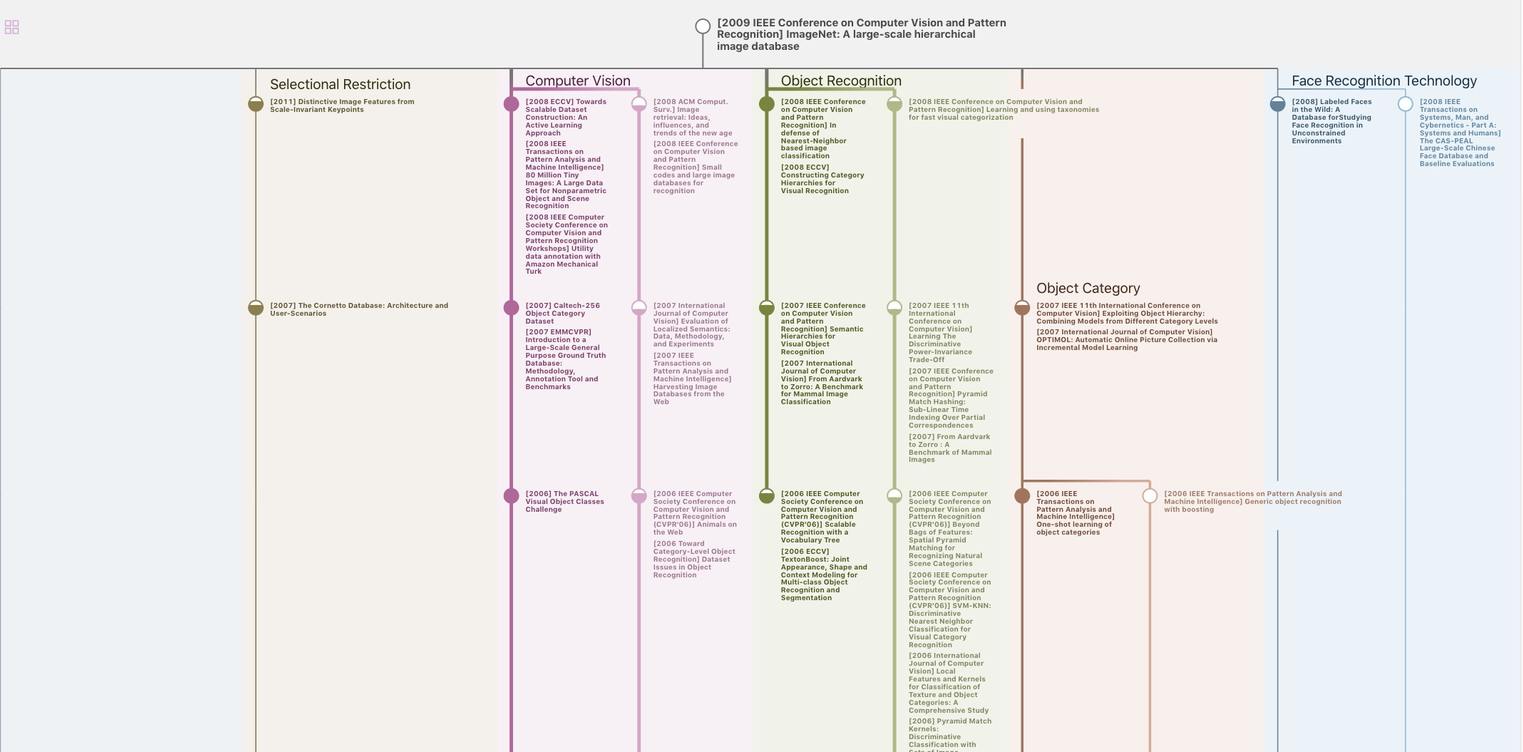
生成溯源树,研究论文发展脉络
Chat Paper
正在生成论文摘要