AMPS: Predicting popularity of short-form videos using multi-modal attention mechanisms in social media marketing environments
JOURNAL OF RETAILING AND CONSUMER SERVICES(2024)
摘要
Emerging as a dominant content format amid the shift from television to mobile, short -form videos wield immense potential across diverse domains. However, the scarcity of datasets and established metrics for their popularity evaluation poses a challenge in accurately reflecting their real -world distribution. In response, our work introduces a dataset and pioneers a cumulative distribution function -based standard tailored specifically for short -form videos. Our model, AMPS (Attention -based Multi -modal Popularity prediction model of Short -form videos) is designed to effectively forecast the popularity of these videos. Considering YouTube Shorts, typically confined to under one minute, our research capitalizes on complete video frames for a holistic prediction of popularity. AMPS harnesses BiLSTM with Self -Attention and Co -Attention mechanisms, enabling a deeper understanding of intra-modal and inter -modal relationships across various modalities. Leveraging full video frame representation, our model significantly enhances prediction accuracy. Comprehensive evaluations against baseline models and machine learning algorithms consistently showcase AMPS' superiority in metrics like GMean, average F1 -score, and Accuracy. Furthermore, when compared with other open social media datasets, our dataset coupled with AMPS consistently outperforms, affirming its robustness and reliability. Additionally, ablation studies underscore the effectiveness of AMPS' architecture and highlight the significance of each modality in predicting popularity.
更多查看译文
关键词
Social media,Popularity prediction,Multi-modal,Deep learning,Short-form content,Attention mechanism
AI 理解论文
溯源树
样例
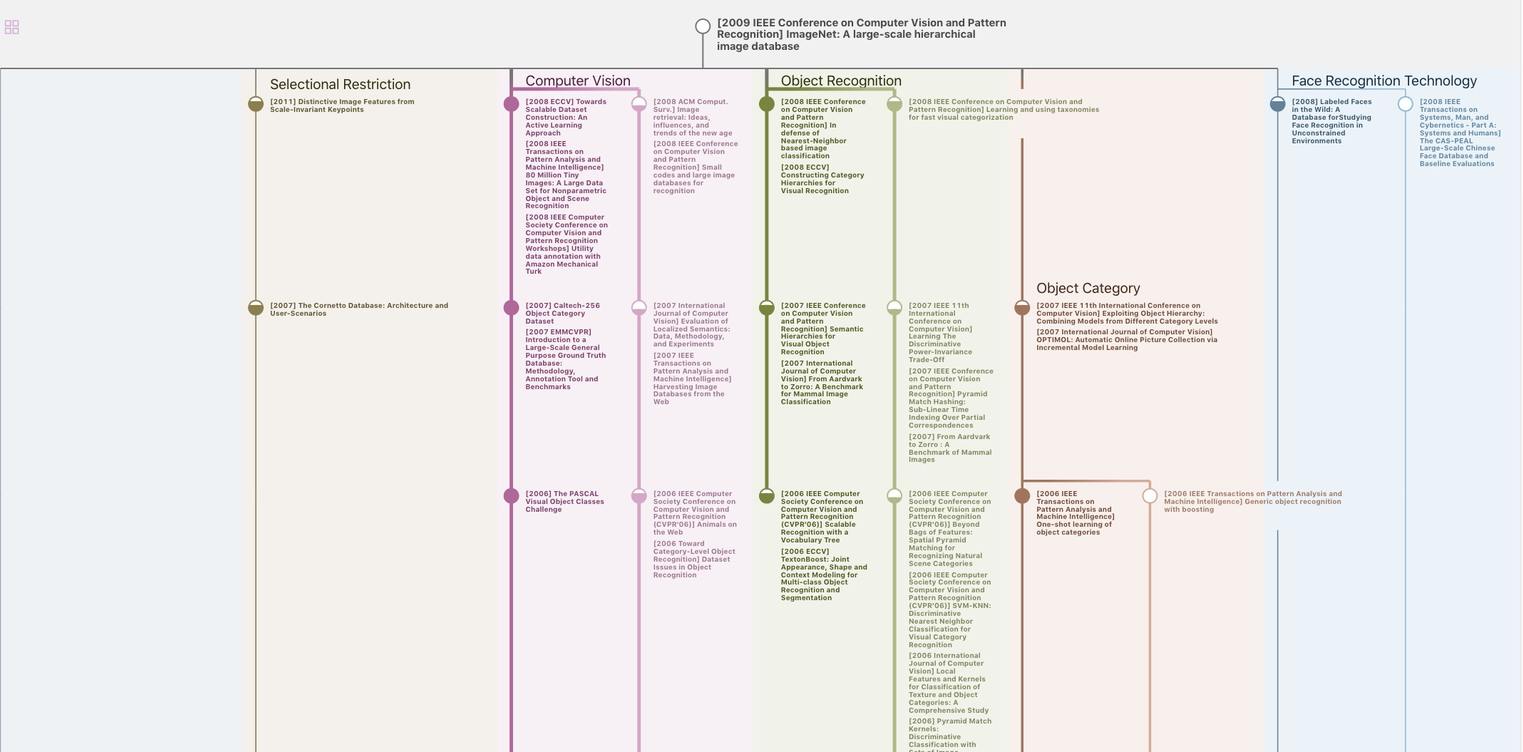
生成溯源树,研究论文发展脉络
Chat Paper
正在生成论文摘要