Linearized maximum rank correlation estimation when covariates are functional
JOURNAL OF MULTIVARIATE ANALYSIS(2024)
摘要
This paper extends the linearized maximum rank correlation (LMRC) estimation proposed by Shen et al. (2023) to the setting where the covariate is a function. However, this extension is nontrivial due to the difficulty of inverting the covariance operator, which may raise the ill -posed inverse problem, for which we integrate the functional principal component analysis to the LMRC procedure. The proposed estimator is robust to outliers in response and computationally efficient. We establish the rate of convergence of the proposed estimator, which is minimax optimal under certain smoothness assumptions. Furthermore, we extend the proposed estimation procedure to handle discretely observed functional covariates, including both sparse and dense sampling designs, and establish the corresponding rate of convergence. Simulation studies demonstrate that the proposed estimators outperform the other existing methods for some examples. Finally, we apply our method to a real data to illustrate its usefulness.
更多查看译文
关键词
Functional principal component analysis,General functional single-index model,Linearized maximum rank correlation,Rate of convergence
AI 理解论文
溯源树
样例
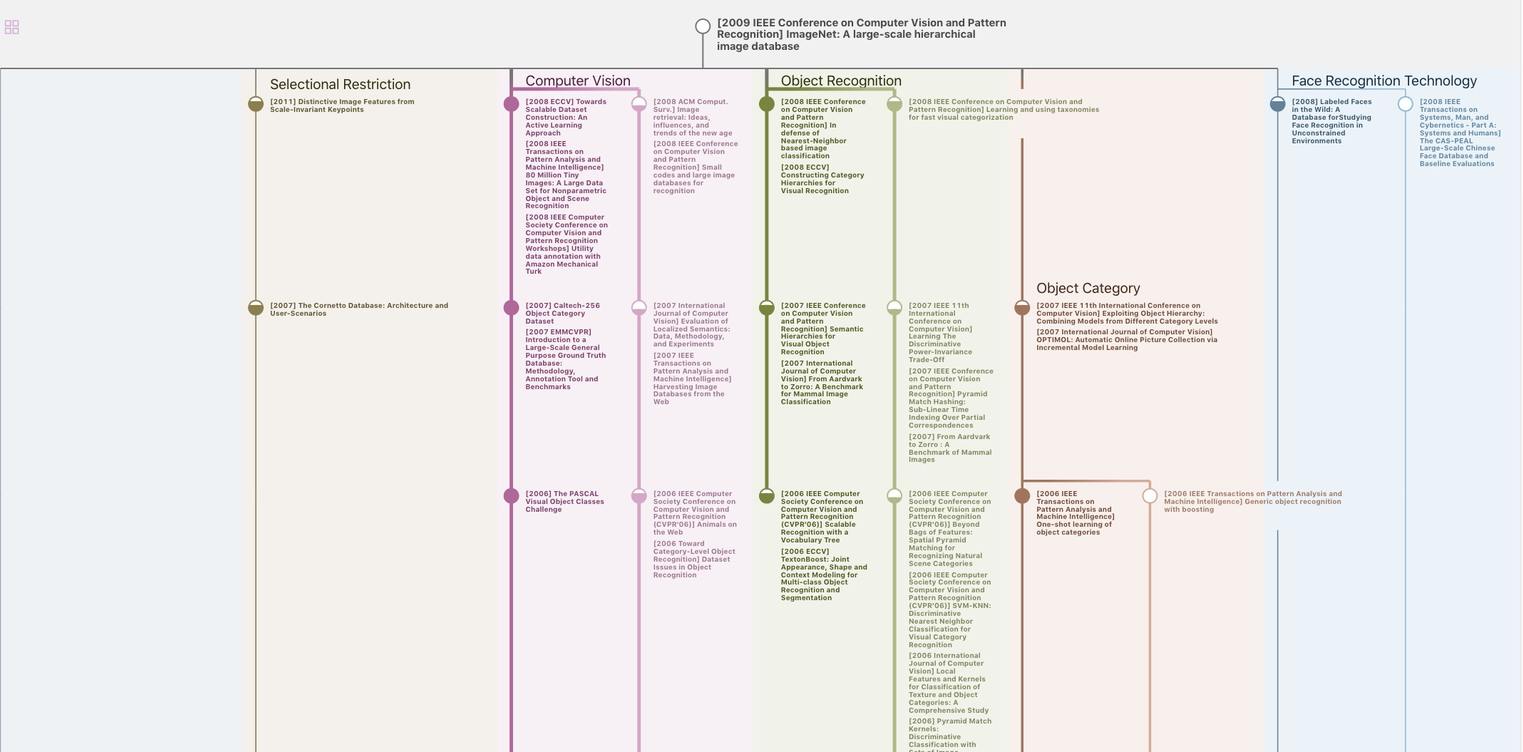
生成溯源树,研究论文发展脉络
Chat Paper
正在生成论文摘要