A novel data augmentation framework for remaining useful life estimation with dense convolutional regression network
JOURNAL OF MANUFACTURING SYSTEMS(2024)
摘要
Deep learning-based methods play an increasingly significant role in prognostic and health management, enabling accurate and rapid estimation of the remaining useful life (RUL) without relying on prior knowledge. In general, sufficient labeled samples are always needed to ensure the successful application of these methods, but the labeled samples are often difficult to obtain in practical engineering scenarios. Thus, a novel data augmentation framework for RUL estimation is proposed in this paper to fully utilize the information contained in the limited labeled data. Firstly, a weighted barycenter averaging technique based on dynamic time warping distance is adopted to generate virtual monitoring data with similar degradation characteristics. Next, the original and generated data are integrated into a modified dense convolutional regression network (DCRN), which improves the flow of information in the network and reduces the possibility of gradient disappearance through tight connections among different layers. Finally, fully connected networks (FCN) are employed to learn the deep and shallow feature representations extracted by DCRN for RUL estimation. Furthermore, the proposed framework is validated on a turbofan engine dataset. Experimental results show it has superior performance when compared with state-of-art algorithms
更多查看译文
关键词
Remaining useful life estimation,Data augmentation,Dense convolutional regression network,Prognostic and health management
AI 理解论文
溯源树
样例
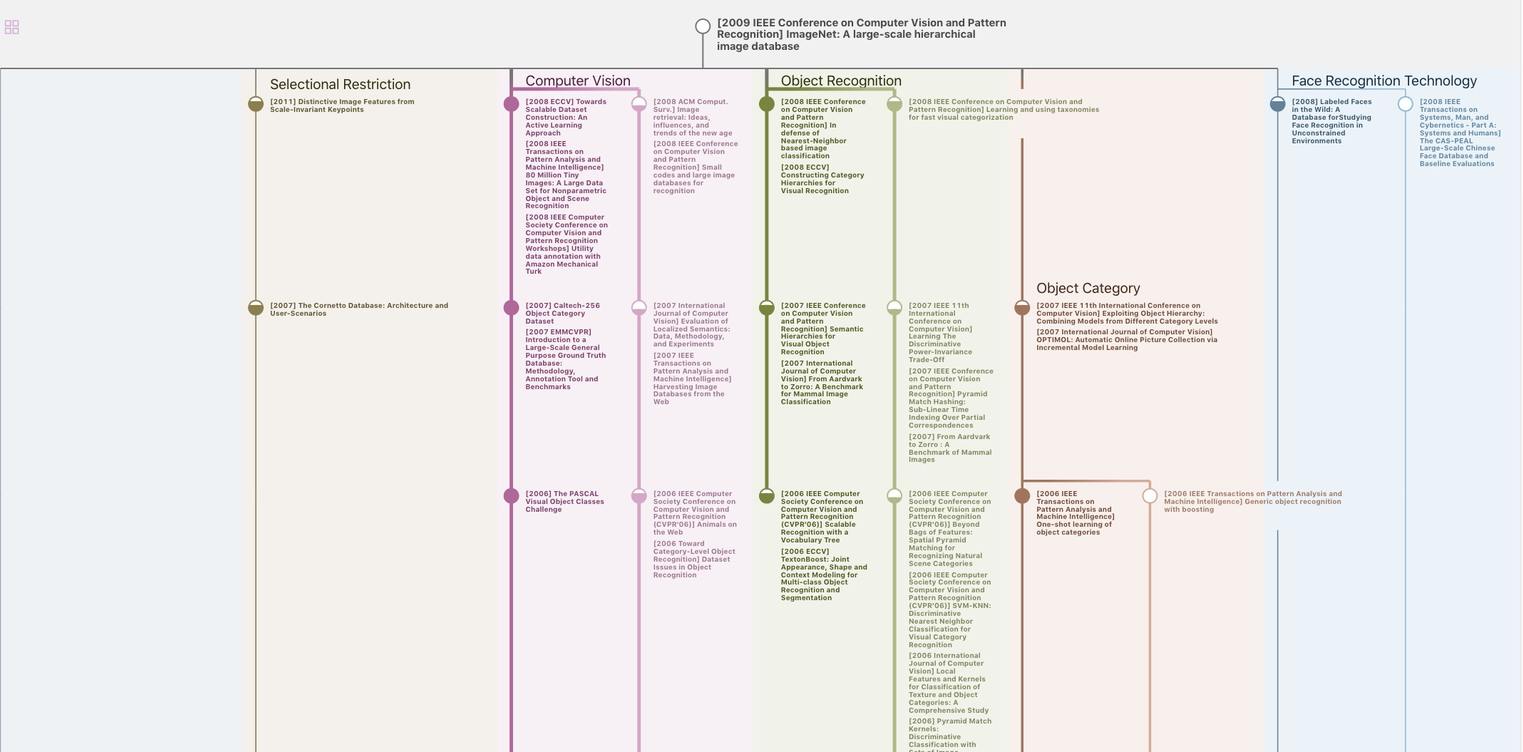
生成溯源树,研究论文发展脉络
Chat Paper
正在生成论文摘要