Statistical Inference for Generalized Power-Law Process in repairable systems
JOURNAL OF COMPUTATIONAL AND APPLIED MATHEMATICS(2024)
摘要
Repairable systems are often used to model the reliability of restored components after a failure is observed. Among various reliability growth models, the power law process (PLP) or Weibull process has been widely used in industrial problems and applications. In this article, we propose a new class of model called the generalized PLP (GPLP), based on change points. These can be treated as known or unknown parameters, or interpreted as failure times. Herein, we consider the impact of all or some fixes on the failure intensity function. In this context, unlike the usual PLP, the GPLP is not restricted to the assumption of minimal repair (MR). Other situations, such as perfect, efficient, and harmful repair, can be considered. We present some special cases of the GPLP, such as the main models used to analyze repairable systems under the assumption of imperfect repair. The estimators of the proposed model parameters were obtained using the maximum likelihood method. We evaluated the performance of the parameter estimators through Monte Carlo (MC) simulations. The proposed approach is fully illustrated using two real failure time datasets.
更多查看译文
关键词
Generalized Power Law Process,Non-Homogeneous Poisson Process,Repairable systems,Point of change,Imperfect repair
AI 理解论文
溯源树
样例
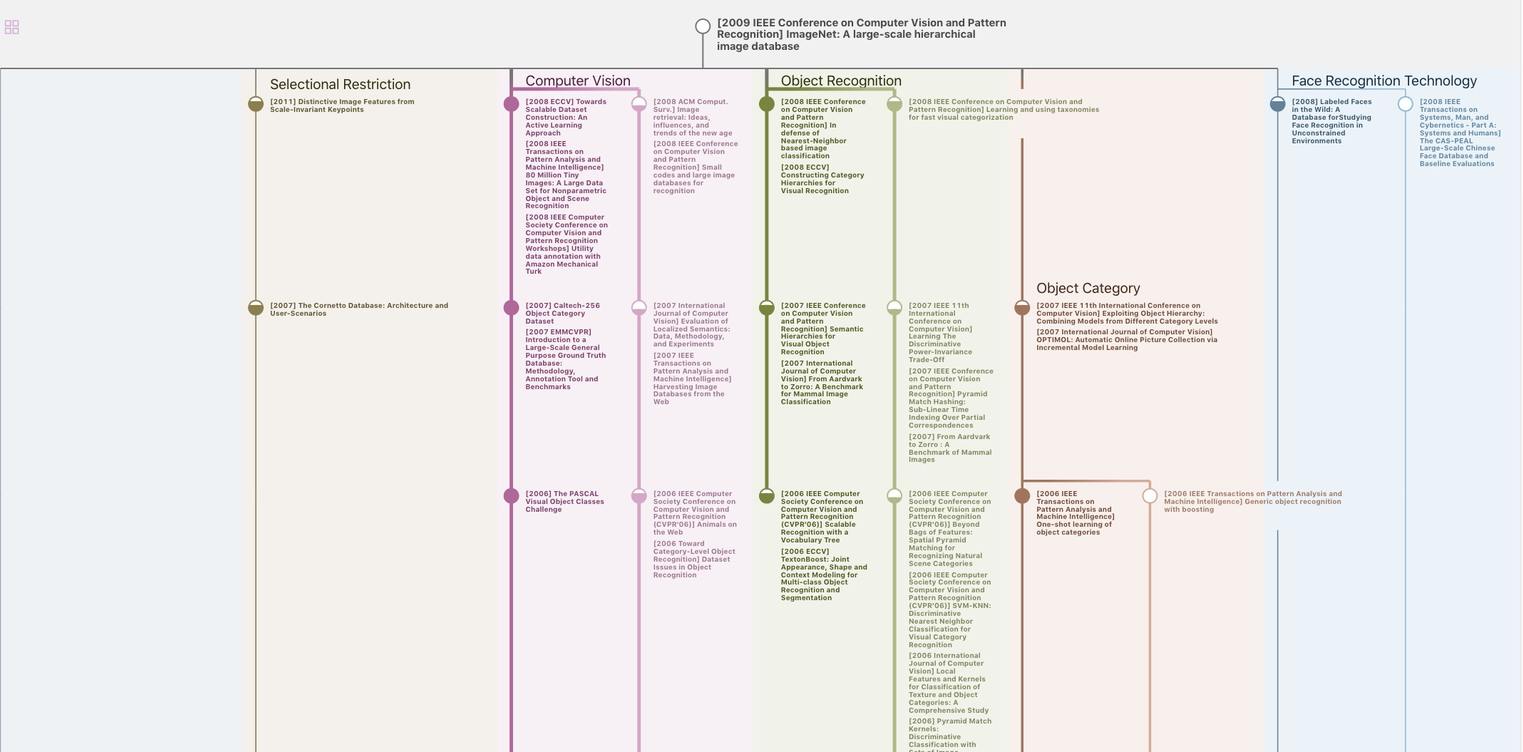
生成溯源树,研究论文发展脉络
Chat Paper
正在生成论文摘要