The use of machine learning techniques to investigate the properties of metakaolin-based geopolymer concrete
JOURNAL OF CLEANER PRODUCTION(2024)
摘要
The construction industry significantly contributes to global greenhouse gas emissions, highlighting the imperative for developing environmentally friendly construction materials. Geopolymers, particularly those utilizing metakaolin (MK), have emerged as a promising green alternative to conventional concrete. However, the acquisition of MK-based geopolymer concrete with optimal mechanical properties poses challenges due to numerous influential factors, disagreement over various findings, and the lack of a reliable predictive model. This study aimed to address this gap by employing a wide range of machine learning methods, namely gradient boosting machine, random forest, decision tree, artificial neural network, and support vector machine. Different optimization and regularization techniques were used to comprehensively understand the factors affecting the compressive strength of MK-based geopolymer concrete, including mixture design, chemical characteristics of the initial binder and activators, and different curing regimes. The results demonstrated the exceptional performance of the gradient boosting machine in predicting the compressive strength of MK-based geopolymer concrete, achieving a coefficient of determination of 0.983 and a mean absolute error of 1.615 MPa. Additionally, the study employed partial dependence plots, feature importance analysis, and SHapley Additive exPlanations (SHAP) to elucidate the proposed models. The coarse-to-fine aggregate ratio, H2O/Na2O molar ratio, extra water content, and sodium hydroxide concentration were identified as the most critical parameters affecting the compressive strength of MK-based geopolymer concrete. This research contributes to advancing the development of sustainable construction materials, streamlining experimental tasks, minimizing the need for labor and materials, improving time efficiency, and providing valuable insights for optimizing the design of MKbased geopolymer concrete.
更多查看译文
关键词
Geopolymer concrete,Metakaolin,Compressive strength,Machine learning,SHAP
AI 理解论文
溯源树
样例
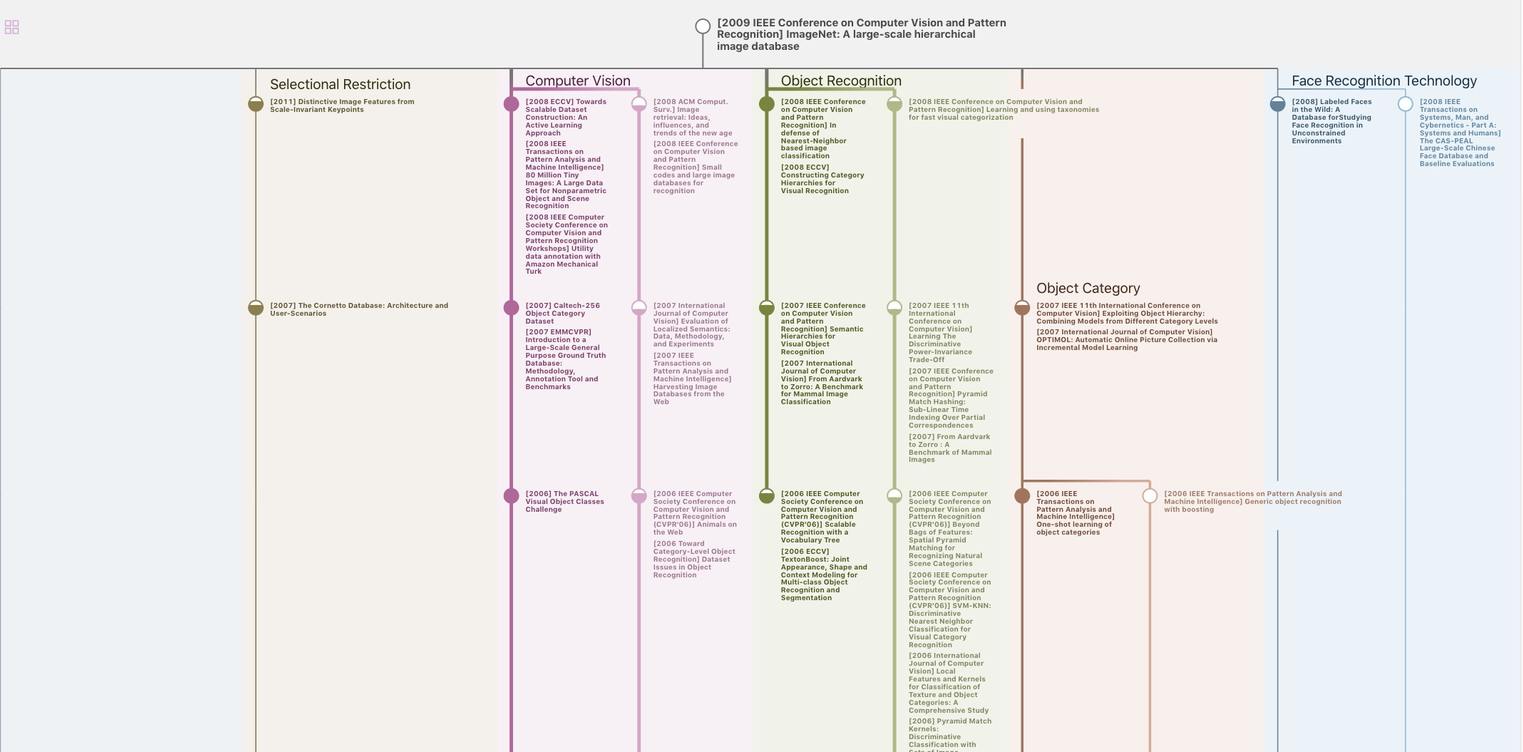
生成溯源树,研究论文发展脉络
Chat Paper
正在生成论文摘要