Electric vehicle charging navigation strategy in coupled smart grid and transportation network: A hierarchical reinforcement learning approach
INTERNATIONAL JOURNAL OF ELECTRICAL POWER & ENERGY SYSTEMS(2024)
摘要
Most of the existing electric vehicle (EV) charging navigation methods do not simultaneously take into account the electric vehicle charging destination optimization and path planning. Moreover, they are unable to provide online real-time decision-making under a variety of uncertain factors. To address these problems, this paper first establishes a bilevel stochastic optimization model for EV charging navigation considering various uncertainties, and then proposes an EV charging navigation method based on the hierarchical enhanced deep Q network (HEDQN) to solve the above stochastic optimization model in real-time. The proposed HEDQN contains two enhanced deep Q networks, which are utilized to optimize the charging destination and charging route path of EVs, respectively. Finally, the proposed method is simulated and validated in two urban transportation networks. The simulation results demonstrate that compared with the Dijkstra shortest path algorithm, single-layer deep reinforcement learning algorithm, and traditional hierarchical deep reinforcement learning algorithm, the proposed HEDQN algorithm can effectively reduce the total charging cost of electric vehicles and realize online realtime charging navigation of electric vehicles, that shows excellent generalization ability and scalability.
更多查看译文
关键词
Electric vehicle,Charging navigation,Hierarchical reinforcement learning,Destination optimization,Route planning
AI 理解论文
溯源树
样例
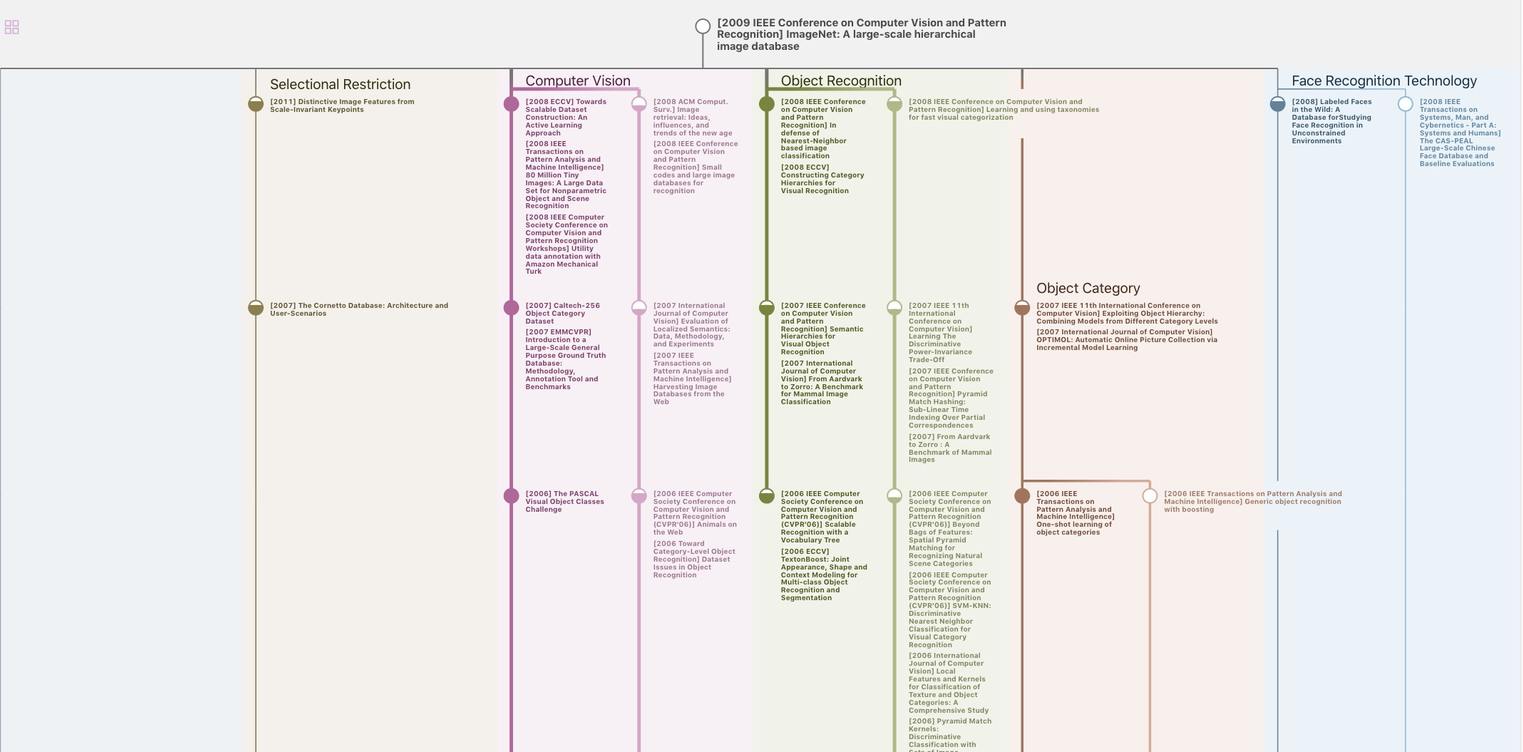
生成溯源树,研究论文发展脉络
Chat Paper
正在生成论文摘要