The multi-task transfer learning for multiple data streams with uncertain data
Information Sciences(2024)
摘要
In existing research on data streams, most problems are processed and studied based on single data streams. However, there exist multiple data streams and the reaching data may contain noise information which is considered uncertain in representation. In this paper, we propose the multi-task transfer learning for multiple data streams with uncertain data (MTUMDS), which can make use of the similarity of multiple data streams to carry out multi-task learning that can improve the classification ability of the data stream model. At the same time, transfer learning is applied for each single data stream, which transfers knowledge from the known classifiers of the previous time windows to the current target window classifier. This can settle the situation that the concept drift causes model fitness reduction. Then, in view of the noise and collection error hidden in the real data, boundary constraints are generated for each sample to build the SVM classifier to solve the uncertainty of the data. A large number of experiments in multiple data streams show that our approach has better performance and robustness than previous studies.
更多查看译文
关键词
Multiple data streams,Transfer learning,Multi-task learning,Uncertain data
AI 理解论文
溯源树
样例
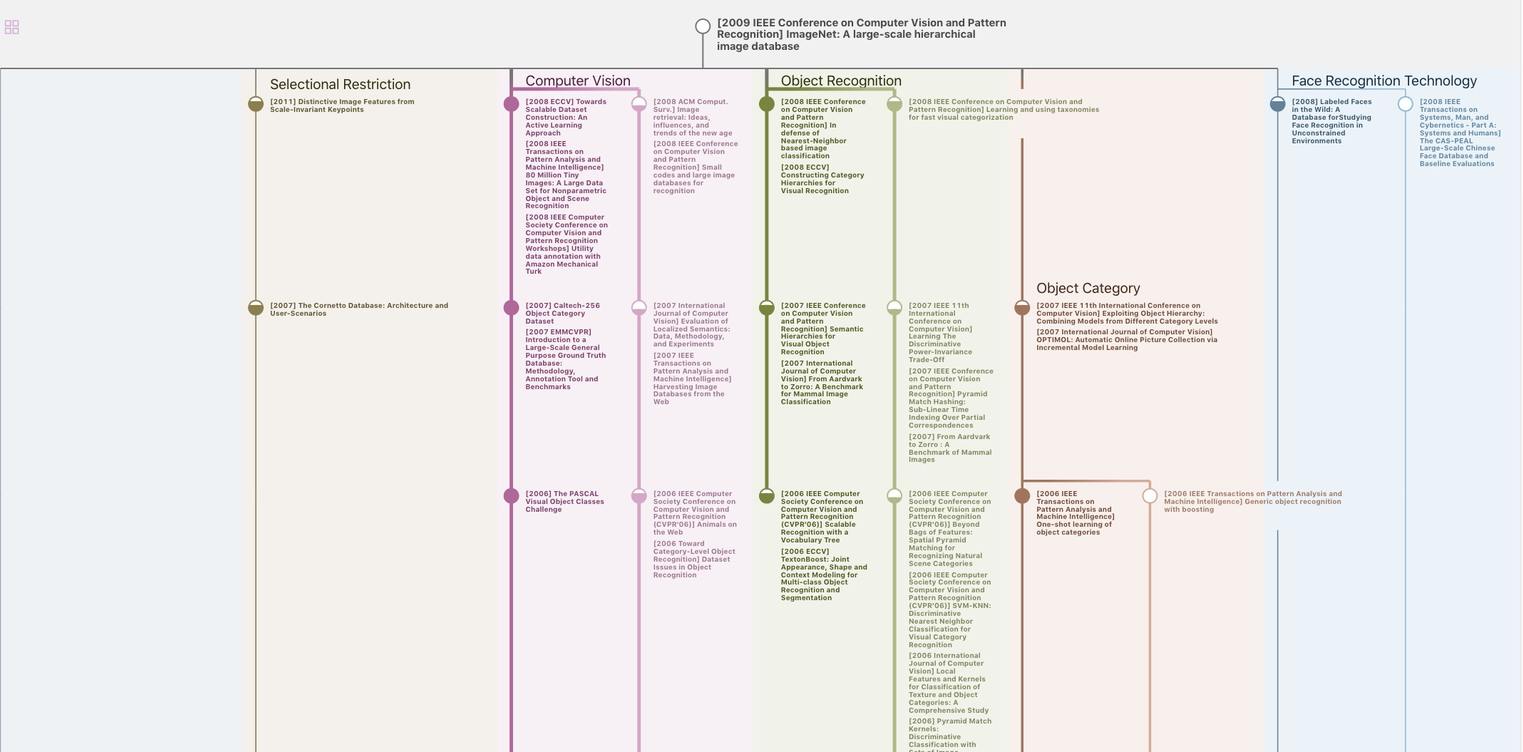
生成溯源树,研究论文发展脉络
Chat Paper
正在生成论文摘要