Maximum output discrepancy computation for convolutional neural network compression
INFORMATION SCIENCES(2024)
摘要
Network compression methods minimize the number of network parameters and computation costs while maintaining desired network performance. However, the safety assurance of many compression methods is based on a large amount of experimental data, whereas unforeseen incidents beyond the experiment data may result in unsafe consequences. In this work, we developed a discrepancy computation method for two convolutional neural networks by giving a concrete value to characterize the maximum output difference between the two networks after compression. Using Imagestar-based reachability analysis, we propose a novel method to merge the two networks to compute the difference. We illustrate reachability computation for each layer in the merged network, such as the convolution, max pooling, fully connected, and ReLU layers. We apply our method to a numerical example to prove its correctness. Furthermore, we implement our developed methods on the VGG16 model with the Quantization Aware Training (QAT) compression method; the results show that our approach can efficiently compute the accurate maximum output discrepancy between the original neural network and the compressed neural network.
更多查看译文
关键词
Reachability analysis,Convolutional neural network,Discrepancy computation,Neural network compression
AI 理解论文
溯源树
样例
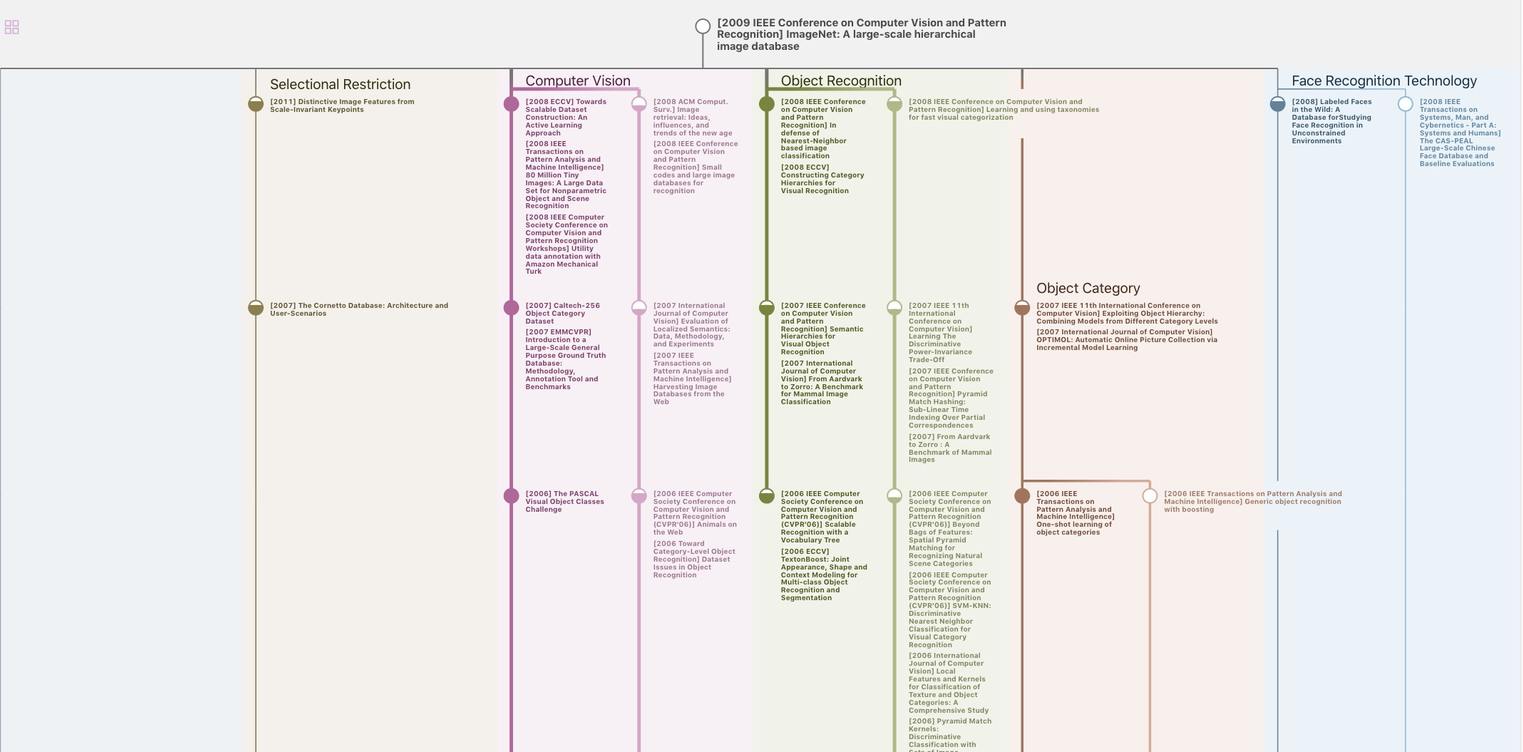
生成溯源树,研究论文发展脉络
Chat Paper
正在生成论文摘要