Multi-modal heart failure risk estimation based on short ECG and sampled long-term HRV
INFORMATION FUSION(2024)
摘要
Cardiovascular diseases, including Heart Failure (HF), remain a leading global cause of mortality, often evading early detection. In this context, accessible and effective risk assessment is indispensable. Traditional approaches rely on resource -intensive diagnostic tests, typically administered after the onset of symptoms. The widespread availability of electrocardiogram (ECG) technology and the power of Machine Learning are emerging as viable alternatives within smart healthcare. In this paper, we propose several multi -modal approaches that combine 30-s ECG recordings and approximate long-term Heart Rate Variability (HRV) data to estimate the risk of HF hospitalization. We introduce two survival models: an XGBoost model with Accelerated Failure Time (AFT) incorporating comprehensive ECG features and a ResNet model that learns from the raw ECG. We extend these with our novel long-term HRVs extracted from the combination of ultra -short-term beat -to -beat measurements taken over the day. To capture their temporal dynamics, we propose a survival model comprising ResNet and Transformer architectures (TFM-ResNet). Our experiments demonstrate high model performance for HF risk assessment with a concordance index of 0.8537 compared to 14 survival models and competitive discrimination power on various external ECG datasets. After transferability tests with Apple Watch data, our approach implemented in the myHeartScore App offers cost-effective and highly accessible HF risk assessment, contributing to its prevention and management.
更多查看译文
关键词
Survival analysis,Heart failure,Electrocardiogram,Heart Rate Variability (HRV),Multi-modal learning
AI 理解论文
溯源树
样例
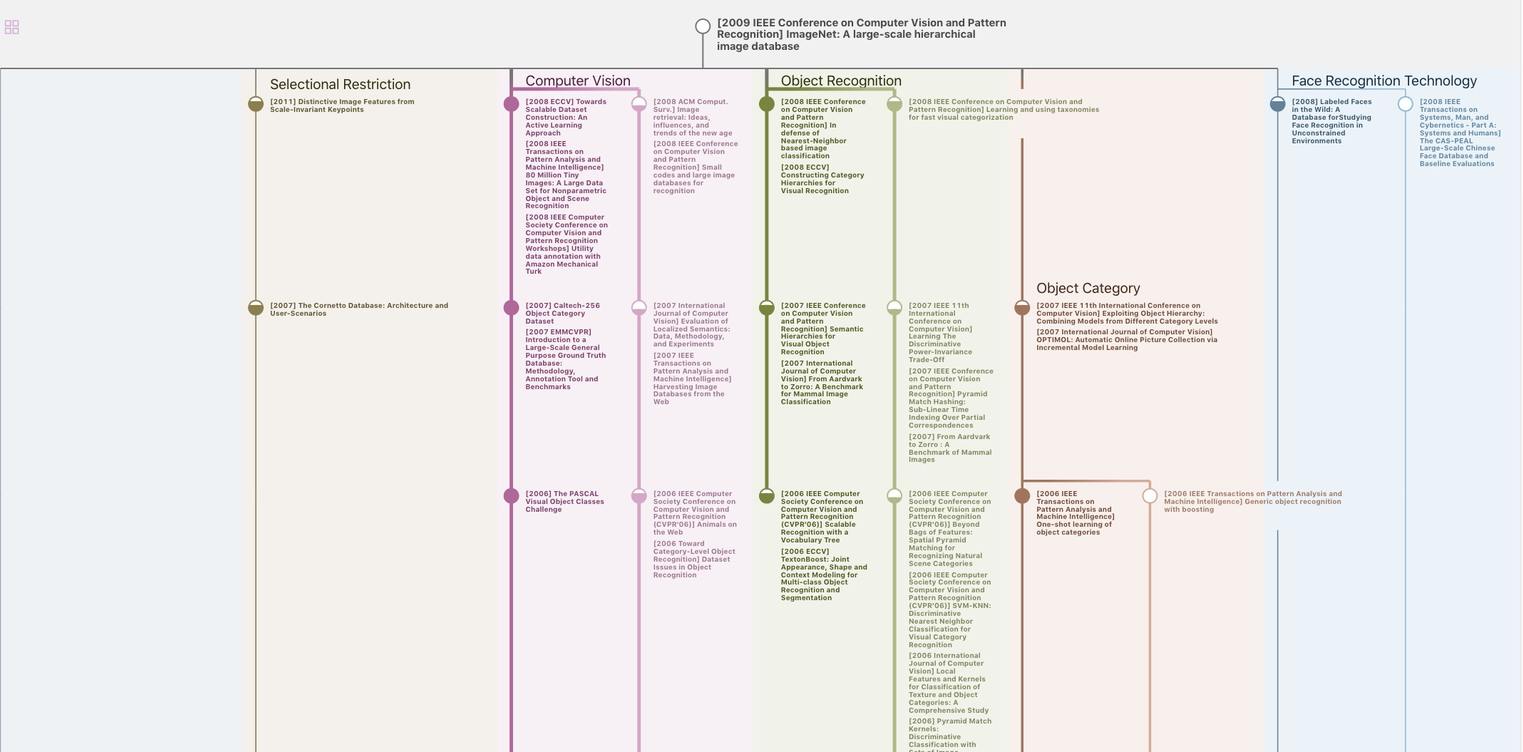
生成溯源树,研究论文发展脉络
Chat Paper
正在生成论文摘要