Remote sensing scene classification using multi-domain sematic high-order network
IMAGE AND VISION COMPUTING(2024)
摘要
Recently, convolutional neural networks (CNNs), which obtain powerful deep features in an end-to-end manner, have achieved powerful performance in remote sensing scene classification. However, the average or maximum pooling operations defined in the spatial domain and coarser-resolution features with high levels cannot extract reliable features and clear boundaries for small-scale targets in remote sensing scene imagery. This paper attempts to address these problems and proposes a multi-domain sematic high-order network for scene classification, named MSHNet. First, wavelet-spatial and detachable pooling blocks defined in the wavelet and spatial domains are inserted at the end of the convolutional block to learn the features in a more structural fusion manner. Second, multi-scale and multi-resolution semantic embedding modules are proposed to take full advantage of the complementary information and effectively maintain the spatial structures of learned deep features. Third, we employ a factorized bilinear coding approach to obtain compact and discriminative secondorder features. MSHNet is thoroughly evaluated on two publicly available benchmarks, i.e., AID (Aerial Image Dataset) and NWPU-RESISC45 (Northwestern Polytechnical University-Remote Sensing Image Scene Classification 45). The extensive results illustrate that our MSHNet is competitive with other related multi-scale deep neural networks.
更多查看译文
关键词
Remote sensing,Scene classification,Convolutional neural networks,Deep semantic feature,Second-order
AI 理解论文
溯源树
样例
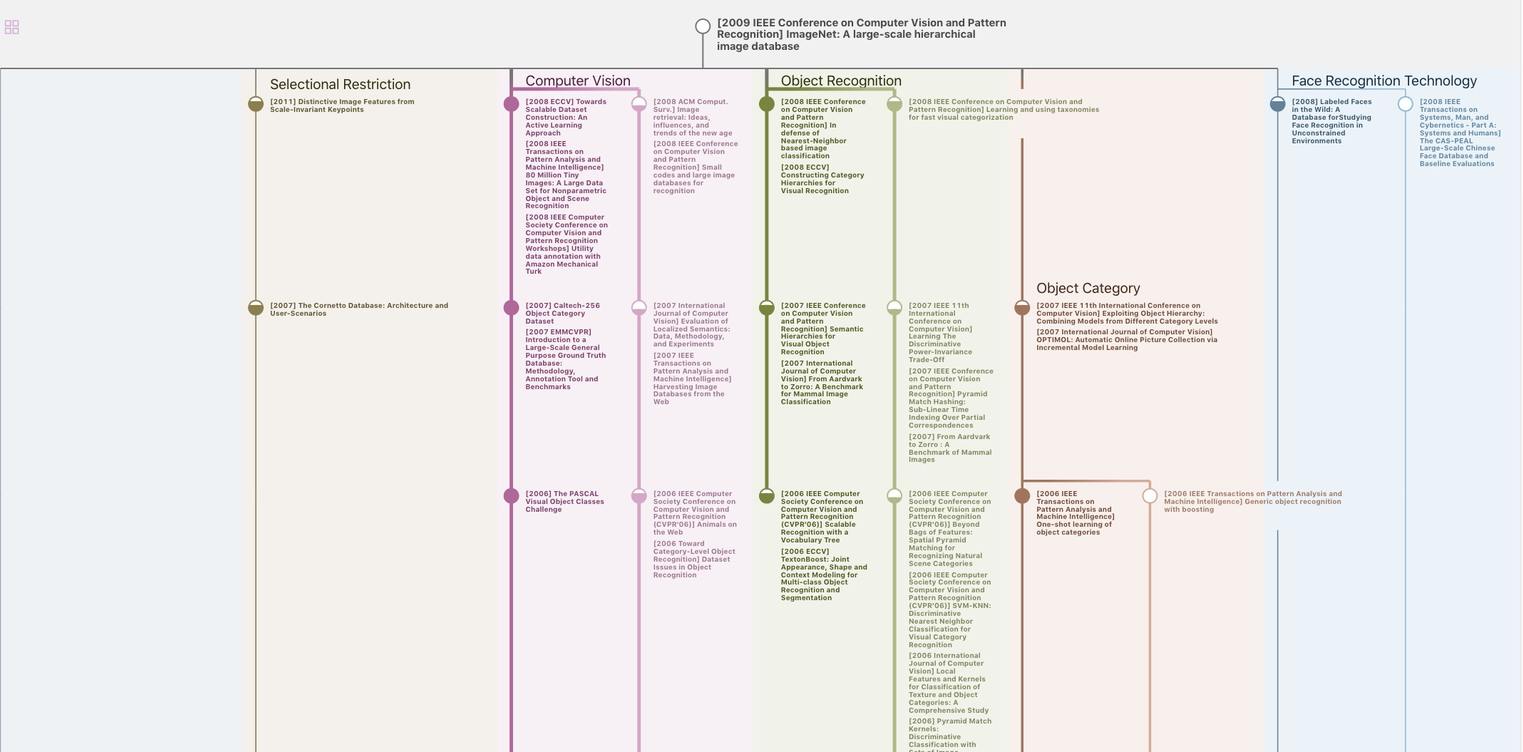
生成溯源树,研究论文发展脉络
Chat Paper
正在生成论文摘要