Enhancing parcel singulation efficiency through transformer-based position attention and state space augmentation
EXPERT SYSTEMS WITH APPLICATIONS(2024)
摘要
Parcel singulation has emerged as a critical bottleneck in the swiftly advancing logistics processes. In the pursuit of a balance between cost-effectiveness and singulation efficiency, an automated parcel singulator utilizing a sparse actuator array is widely acknowledged as the optimal solution to address this challenge. However, its successful operation necessitates the implementation of a sophisticated control policy. In this work, we tackle the problem of parcel singulation by formulating it as a Markov Decision Process (MDP) within a variable state space dimension. Traditional Deep Reinforcement Learning struggles with variable state dimensions and task -specific priority learning, necessitating adaptable state representations and the implementation of advanced learning algorithms. In this study, we introduce a novel DRL algorithm, designated as Transformer -based Position Attention and State Space Augmentation Soft Actor-Critic (TPASSA-SAC). This algorithm incorporates Transformer -based attention mechanisms, specifically tailored to prioritize processing of parcels based on their spatial positions. Moreover, the unique aspect of TPASSA-SAC lies in its capability to enhance Q -value estimations by employing State Space Augmentation. This approach not only refines the decision -making process but also contributes to a more robust and accurate learning paradigm. Furthermore, we have developed a simulation environment that is grounded in real -world data distributions specific to parcel singulation. Our experimental findings conclusively establish the enhanced performance of our proposed TPASSA-SAC, distinguishing it from existing DRL-based models and conventional singulation techniques. TPASSA-SAC demonstrates superior efficacy, evidenced by the highest parcel pass rates observed (ranging from 99.62% to 99.96%) and unparalleled throughput efficiency, processing in excess of 5036 parcels per hour across a variety of scenarios.
更多查看译文
关键词
Parcel singulation,Deep reinforcement learning,Non-stationary environment,State space augmentation,Soft actor-critic
AI 理解论文
溯源树
样例
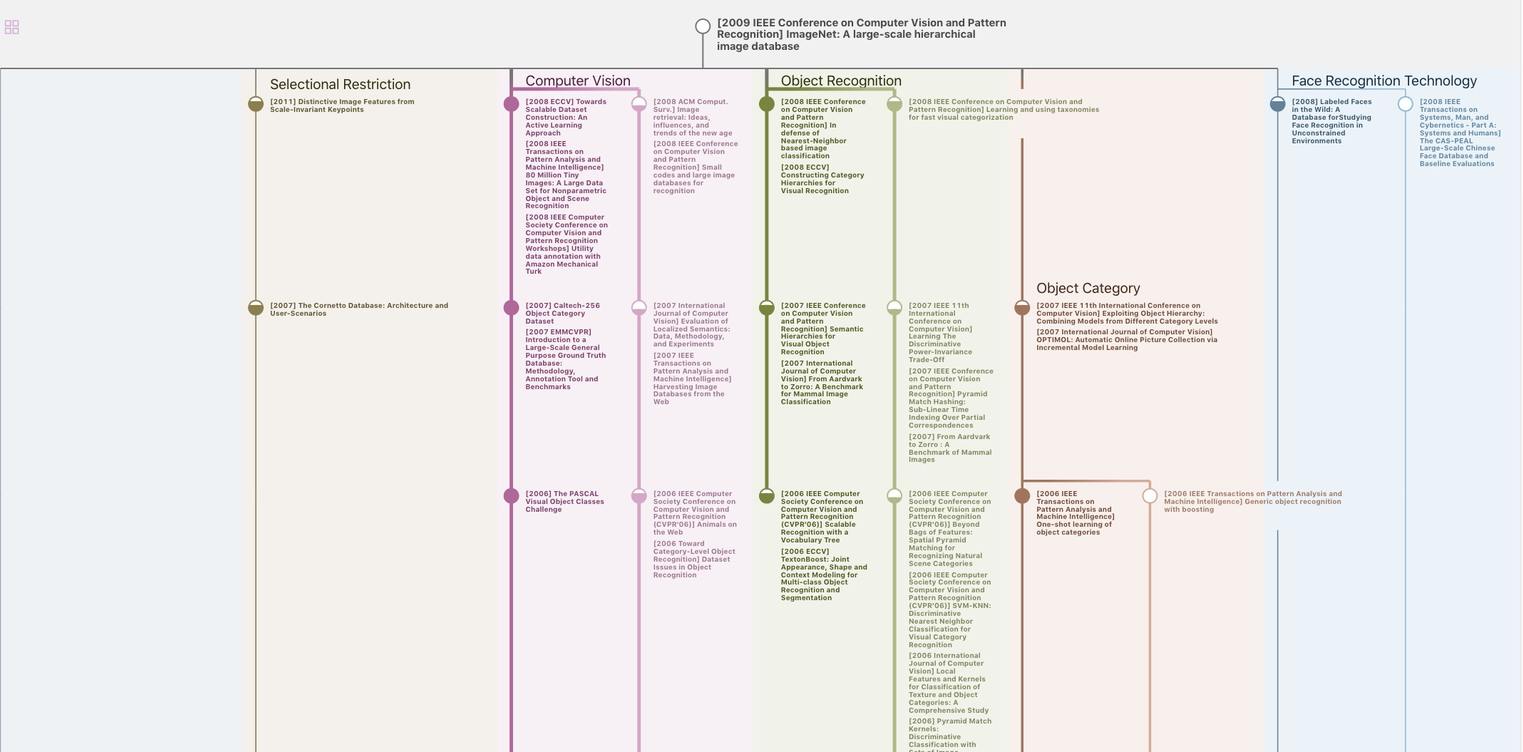
生成溯源树,研究论文发展脉络
Chat Paper
正在生成论文摘要