Fisher ratio-based multi-domain frame-level feature aggregation for short utterance speaker verification
ENGINEERING APPLICATIONS OF ARTIFICIAL INTELLIGENCE(2024)
摘要
As the durations of the short utterances are small, it is difficult to learn sufficient information to distinguish the person, thus, short utterance speaker recognition is highly challenging. In this paper, we propose a multi-domain frame-level feature joint learning method to aggregate the discriminative information from multiple dimensions and domain, which is different domains of the speech, time-domain, frequency-domain, and spectral-domain, represent distinct physical characteristics and provide different dimension information, the time domain captures information about the temporal aspect of the physical signal, the frequency domain represents the signal strength in different frequency ranges, and the spectral domain reflects the overall information of the speech, then, based on the extracted multi-domain frame-level features, using the Multi-Fisher criterion aggregates feature parameters categorically and match the corresponding Multi-Fisher ratio weights to the feature parameters as a way to achieve effective feature aggregation and to preserve more effective information, termed FirmDomain. Extensive experiments are carried out on short-duration text-independent speaker verification datasets derived from the VoxCeleb, SITW, and NIST SRE corpora, which contain speech samples of varying lengths and scenarios. The results demonstrate that the proposed method outperforms the state-of-the-art deep learning architectures by at least 13%, respectively, in the test set. The results of the ablation experiments demonstrate that our proposed methods can significantly outperform previous approaches.
更多查看译文
关键词
Multi -domain feature,Joint learning,Feature enhancement,Discriminative embedding,Speaker verification,Fisher-ratio
AI 理解论文
溯源树
样例
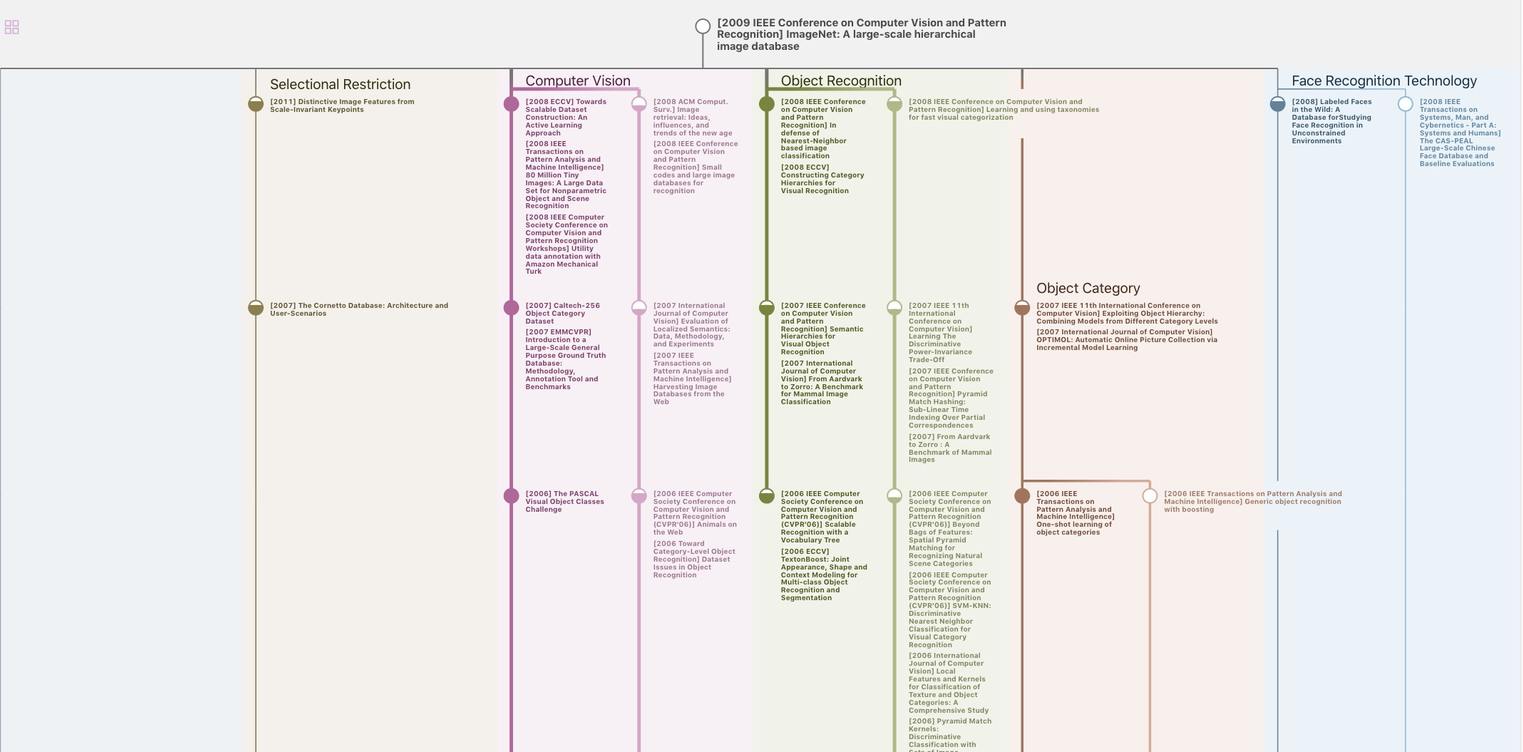
生成溯源树,研究论文发展脉络
Chat Paper
正在生成论文摘要