Cross-modal Semantic Interference Suppression for image-text matching
ENGINEERING APPLICATIONS OF ARTIFICIAL INTELLIGENCE(2024)
摘要
Image -text matching, which aims at precisely measuring the visual -semantic similarities between images and texts, is a fundamental research topic in multimedia analysis domain. Current methods have obtained an impressive performance by taking advantage of Transformer architecture. However, most of them only consider inter -modal relationships to mine the image -text semantic correspondences, which makes them hard to accurately measure the similarity when facing similar images and text due to the cross -modal semantic interferences. In this work, to tackle the issue mentioned above, we propose a Cross -Modal Semantic Interference Suppression (CMSIS) method, which incorporates intra-modal fine-grained semantics and unmatched segments to suppress the semantic influences caused by similar heterogeneous data points. The intra-modal fine-grained semantics are utilized to push similar images or text away in the learned latent embedding space for better matching results. To further suppress the cross -modal semantic interferences among similar data points, the unmatched segments that can provide explicit clues to distinguish similar images or text, is also adopted. Experimental results on two popular multimodal datasets have demonstrated that the proposed CMSIS outperforms a range of baselines.
更多查看译文
关键词
Image-text matching,Intra-modal learning,Semantic relation,Semantic interference,Negative similarty
AI 理解论文
溯源树
样例
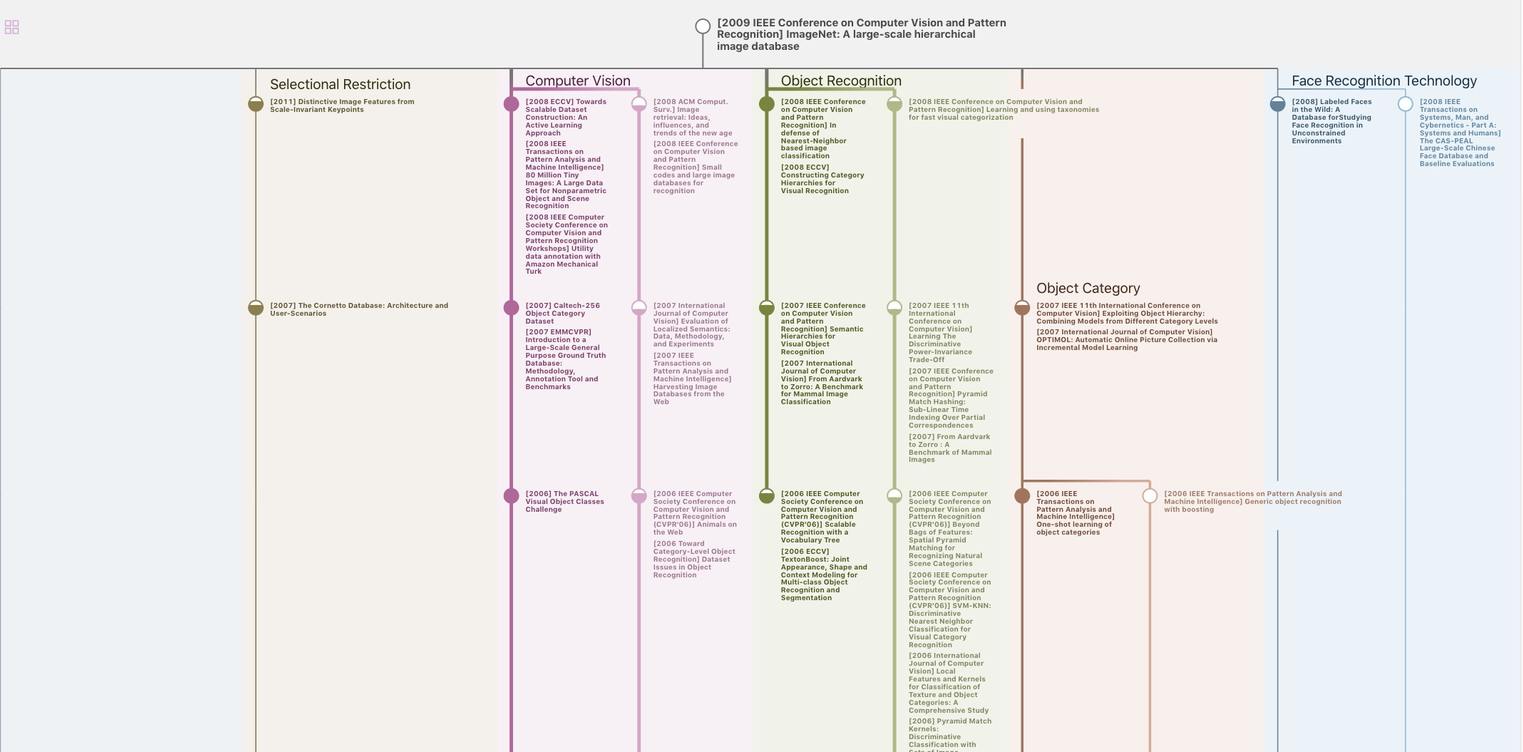
生成溯源树,研究论文发展脉络
Chat Paper
正在生成论文摘要