Collaborative and Discriminative Subspace Learning for unsupervised multi-view feature selection
Engineering Applications of Artificial Intelligence(2024)
摘要
By effectively exploiting the consistent information of multi-view data, multi-view feature selection seeks to select crucial features from multiple heterogeneous data views to improve the clustering and classification accuracy of multi-view data in pattern recognition, data mining, and machine learning. However, most unsupervised multi-view feature selection models investigate the consistent information based on the correlations between data, which may not be accurate enough to reveal data distribution because of the noisy features. Moreover, the consistent information could be insufficient to capture the full information of multi-view data because each individual view typically contains a portion of information of the data. To overcome these issues, we propose Collaborative and Discriminative Subspace Learning (CDSL) for unsupervised multi-view feature selection. Our model collaborates different views and encodes the full information of multi-view data into latent representations via robust collaborative subspace learning to exploit cross-view information of multi-view data, and discriminates the data features in different views via discriminative subspace learning to exploit the within-view information. Furthermore, it learns a collaborative graph for data by leveraging representations of data in both the collaborative subspace and the discriminative subspaces to investigate the underlying structure of multi-view data. Following that, an efficient algorithm is designed to solve the optimization problem. Extensive experiments on several benchmark datasets demonstrate the superiority of the proposed method over state-of-the-art approaches.
更多查看译文
关键词
Multi-view learning,Unsupervised Feature Selection,Subspace learning,Correlation learning
AI 理解论文
溯源树
样例
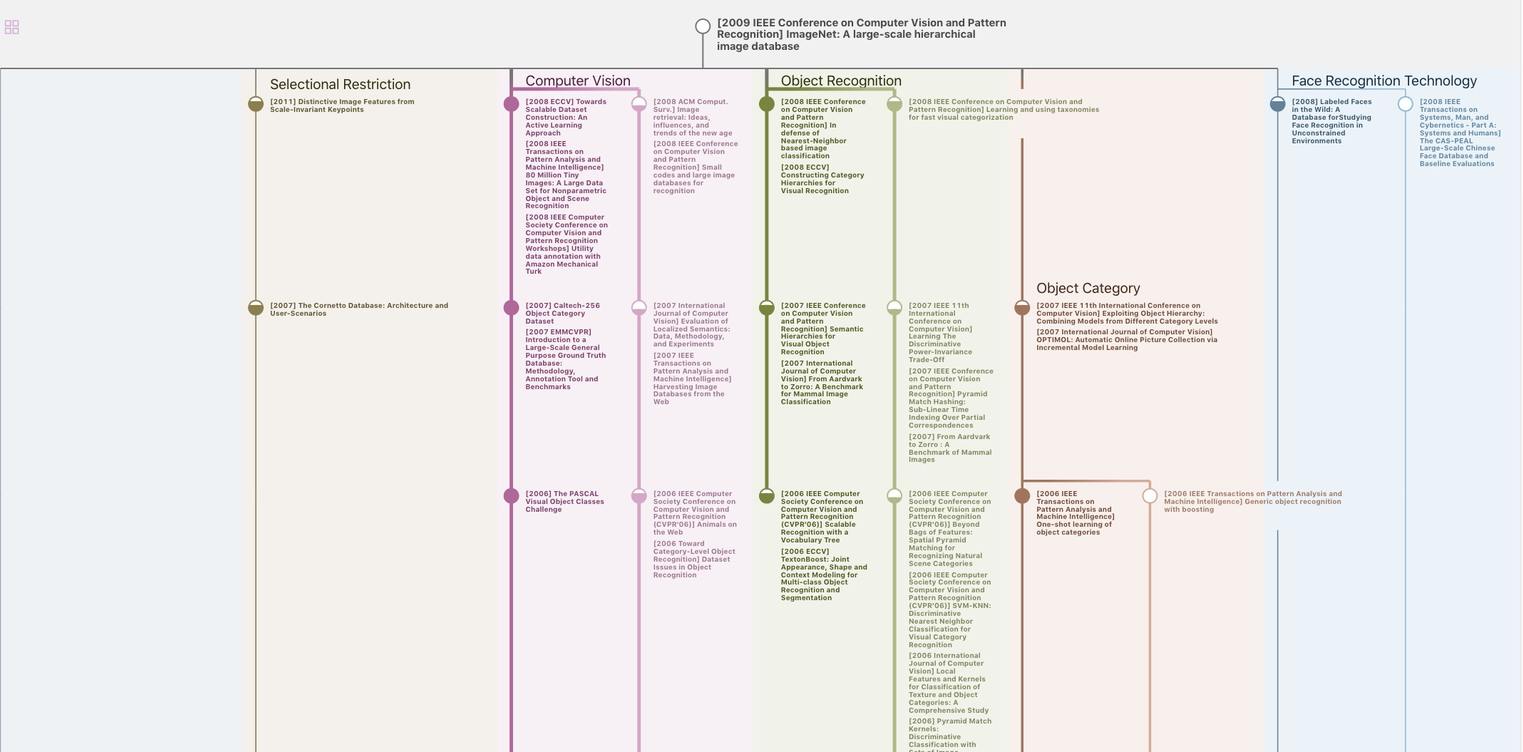
生成溯源树,研究论文发展脉络
Chat Paper
正在生成论文摘要