Short-term trajectory prediction for individual metro passengers based on multi-level periodicity mining from semantic trajectory
Engineering Applications of Artificial Intelligence(2024)
摘要
Short-term trajectory prediction for individual metro passengers is an essential component of many location-based applications, such as bottleneck identification, anomalous trajectory detection, and emergency evacuation. Accurate and interpretable trajectory prediction in short-term remains challenging since the trajectory of individual metro passengers is influenced by time-varying and periodic mobility patterns. Moreover, it is still difficult for deep learning-based prediction approaches to intuitively explain the individual periodic mobility patterns captured by the black-box model. In this study, a long short-term memory method based on multi-level periodicity mining (LSTM-MP) from semantic trajectory is developed to achieve accurate and interpretable short-term trajectory prediction for individual metro passengers. The newly defined semantic trajectories of metro passengers that combine the semantic information about the geometric and operational characteristics of the metro with the raw trajectory data are constructed to help obtain fine-grained periodic mobility patterns of individual passengers. Multi-level periodicity which includes long-range, weekly-interval, and daily-interval periodicities, is calculated with variable time granularity and its polymerization from the constructed semantic trajectories. Finally, the characteristics of multi-level periodicity are considered by developing a principal trajectory attention mechanism to select the most relevant trajectories for prediction in the LSTM-MP framework. The prediction performance of LSTM-MP is compared with 2-order Markov chain and Naïve-LSTM algorithms using the large real-world anonymous passenger WiFi probe dataset. Experimental results show that LSTM-MP can predict when and where an individual will be in the future time series with an average accuracy of 82.3%. This demonstrates the effectiveness of LSTM-MP in the short-term trajectory prediction for individual metro passengers. Potential applications of the short-term trajectory prediction for individual metro passengers are also identified.
更多查看译文
关键词
Intelligent transportation system,Short-term trajectory prediction,Multi-level periodicity mining,Semantic trajectory,WiFi probe data
AI 理解论文
溯源树
样例
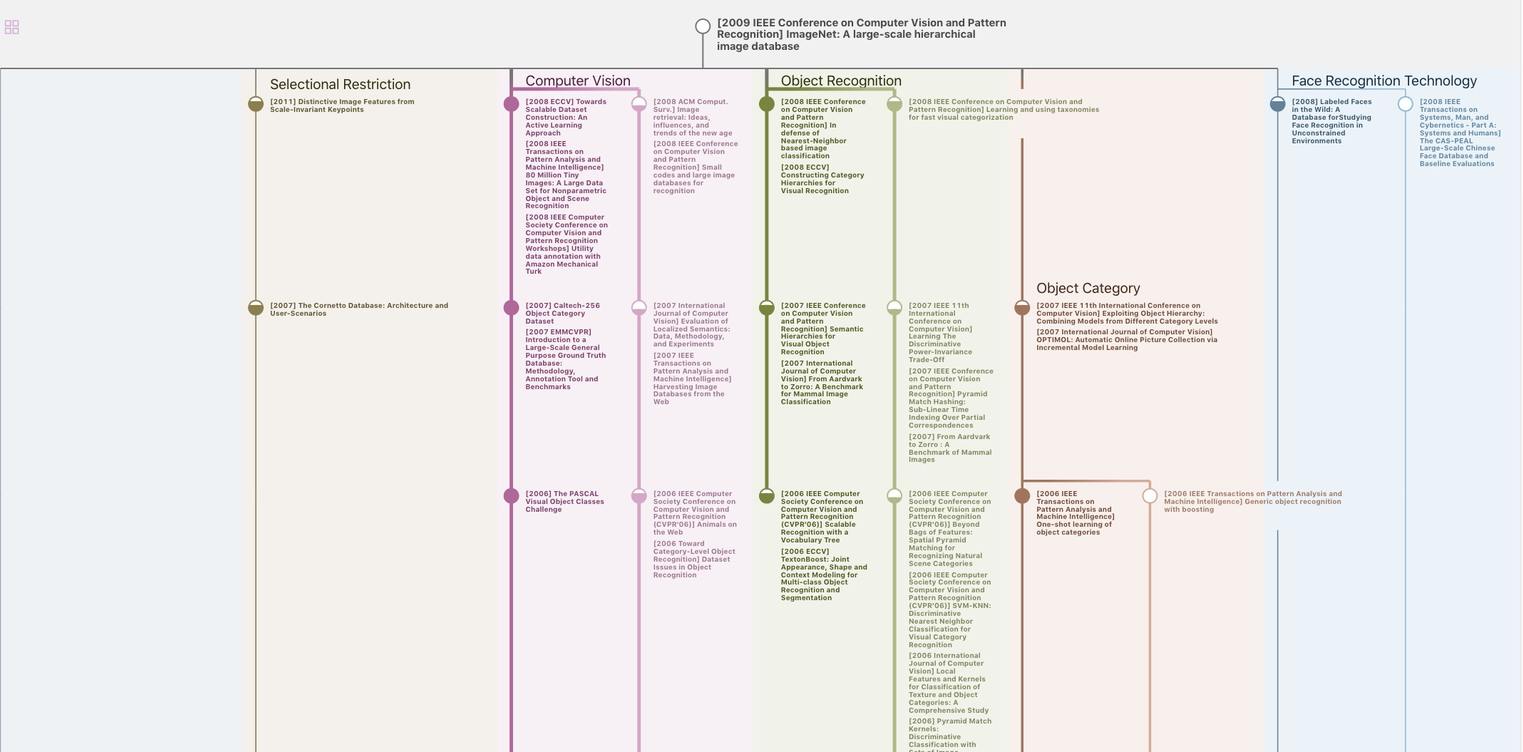
生成溯源树,研究论文发展脉络
Chat Paper
正在生成论文摘要