Bi-level hierarchical model with deep reinforcement learning-based extended horizon scheduling for integrated electricity-heat systems
ELECTRIC POWER SYSTEMS RESEARCH(2024)
摘要
The integration of heat storage tank (HST) in the integrated electricity-heat system (IEHS) has been widely adopted to facilitate the accommodation of wind power. However, existing studies generally put a simplified end-of-period heat capacity (EHC) constraints of the HST, which limits the utilization of potential heat supply in subsequent dispatch periods. To addresses the issue, this paper proposes an innovative scheduling scheme with an extended decision horizon for the IEHS, considering the optimal status of the HST at the end of each dispatch period. To implement this scheme, a bi-level hierarchical model is developed, which integrates a high-level planning model based on deep reinforcement learning with a low-level scheduling model based on mathematical programming. The high-level planning model aims to learn the optimal policy for determining a suitable EHC in accordance with the heat release needs of subsequent dispatch periods. Meanwhile, the low-level scheduling model incorporates the EHC as a constraint and formulates a mixed-integer quadratic programming subproblem, wherein the reward is computed and fed back to the high-level model to guide the policy learning. Experimental results conducted on the modified 6-bus test system verified the effectiveness and economic benefits of the proposed scheduling scheme, which significantly enhances the wind power accommodation capacity of the IEHS.
更多查看译文
关键词
Heat storage tank,Deep reinforcement learning,Integrated electricity -heat system,Wind power accommodation
AI 理解论文
溯源树
样例
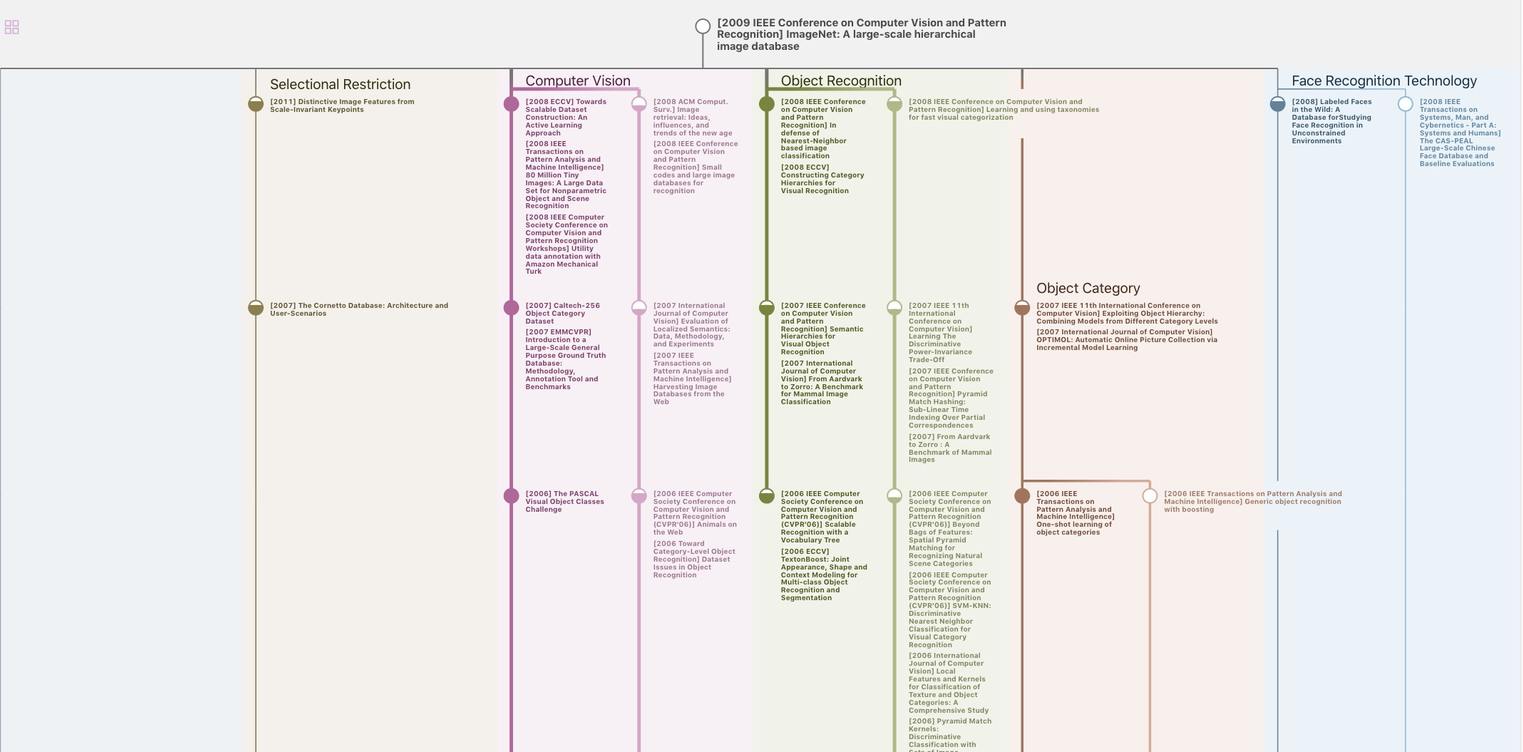
生成溯源树,研究论文发展脉络
Chat Paper
正在生成论文摘要