Efficient extraction of corn rows in diverse scenarios: A grid-based selection method for intelligent classification
COMPUTERS AND ELECTRONICS IN AGRICULTURE(2024)
摘要
In various complex field environments, machine learning -based crop row detection faces challenges like rigidity and low adaptability. To address this issue, we integrated deep learning into agricultural analysis and established a diverse dataset of corn fields across various scenarios. By employing an end -to -end CNN model and predicting row and column anchors, we created a grid -like understanding of images, significantly streamlining the crop row detection process without the need for pixel -level segmentation. This innovative approach offers a novel method for comprehending the spatial structure of crop rows. Furthermore, we extended the concept of agricultural machinery movement core areas to our data annotation strategy, eliminating the need for pre -selecting ROI regions during crop row extraction. Experimental results demonstrate that our Row and Column Anchor Selection Classification (RCASC) method surpasses conventional approaches in terms of versatility, achieving an F1 score of 92.6 %. It can autonomously extract agricultural machinery movement areas, with video stream processing frame rates exceeding 100FPS and an average image processing time of approximately 10 ms. This method not only meets the real-time requirements for corn crop row recognition but also operates effectively in various special scenarios, offering a feasible solution for further advancing agricultural automation and precision.
更多查看译文
关键词
Visual navigation,Deep Learning,Maize crop row detection,Early corn crops,Image classification
AI 理解论文
溯源树
样例
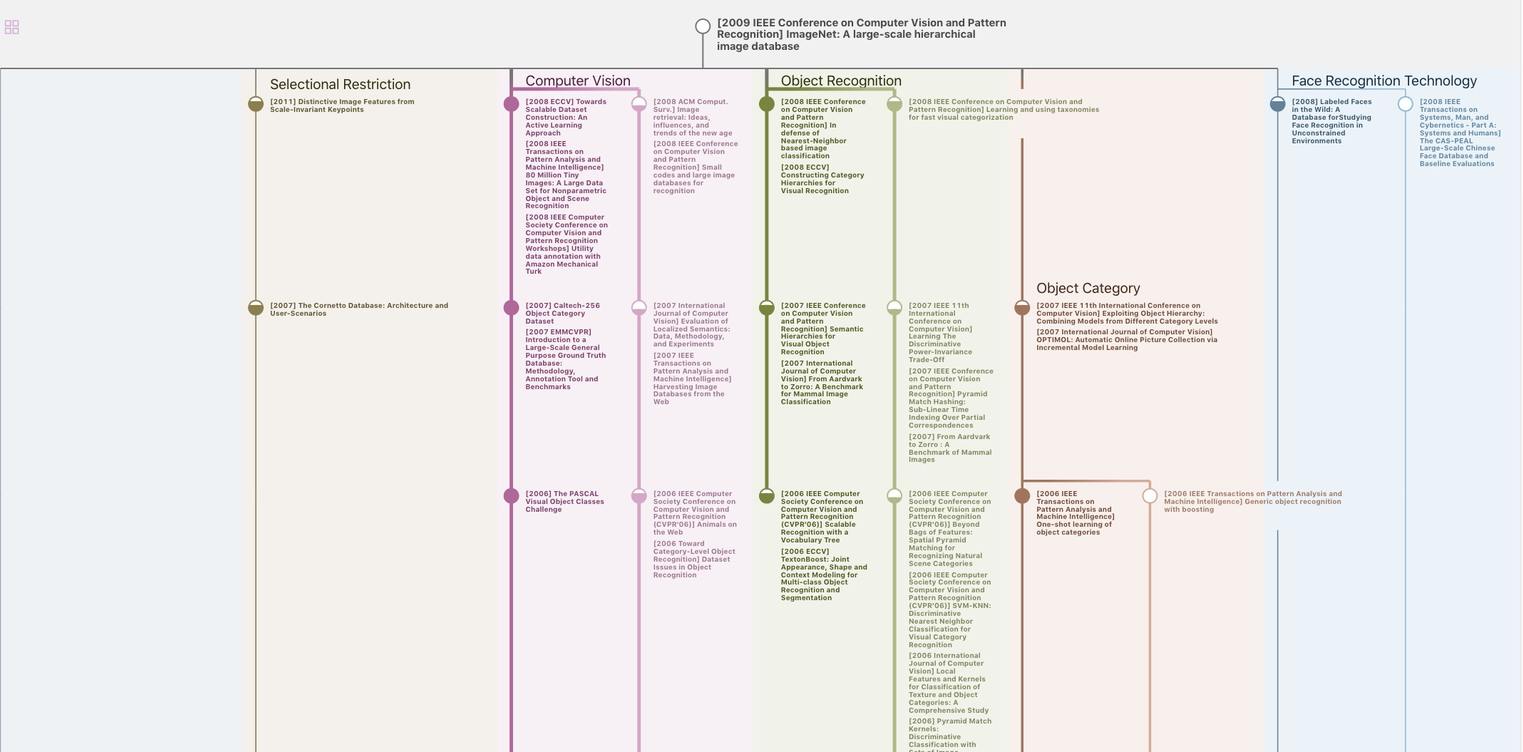
生成溯源树,研究论文发展脉络
Chat Paper
正在生成论文摘要