A deep multi-scale neural networks for crime hotspot mapping prediction
COMPUTERS ENVIRONMENT AND URBAN SYSTEMS(2024)
摘要
Prediction of high-risk areas for urban crime is of great significance for maintaining public safety and sustainable development. However, existing approaches are deficient in spatiotemporal sensitivity and perceptivity, which make it difficult to extract the spatiotemporal dependency from uneven and sparsely distributed data. To address this problem, the novel multi-scale neural network models, namely ST-HGNet and ST-HGNet(a) with attention, were proposed. It is dedicated to further exploring spatiotemporal patterns and improving hotspot location prediction accuracy for sparse types of crimes. First, multi-scale conception and attention mechanisms were introduced to address the receptive field range fixed problem. It enhanced representation of captured information by exposing spatial "scale" dimension and assigning weight relationships. Then, novel multi-scale hierarchical gating architecture was designed that has two forms of whether to add attention or not, to enhance the sensitivity of features and the perception of sparse features by filtering the valid information at different scales. Ultimately, the periodic temporal components were used to capture different time-trend dependencies. The proposed model adopted well-known Chicago assault crime dataset as a case study. Compared with five common benchmark models, the results show that the ST-HGNet model outperformed other baseline models and achieved higher prediction accuracy at multiple level spatial resolution. In particular, ST-HGNet(a) with self-attention achieved the greatest improvement at 1000 m, with a mean hit rate of more than 84%.
更多查看译文
关键词
Crime prediction,Multi-scale,Hotspot mapping,Spatiotemporal dependencies
AI 理解论文
溯源树
样例
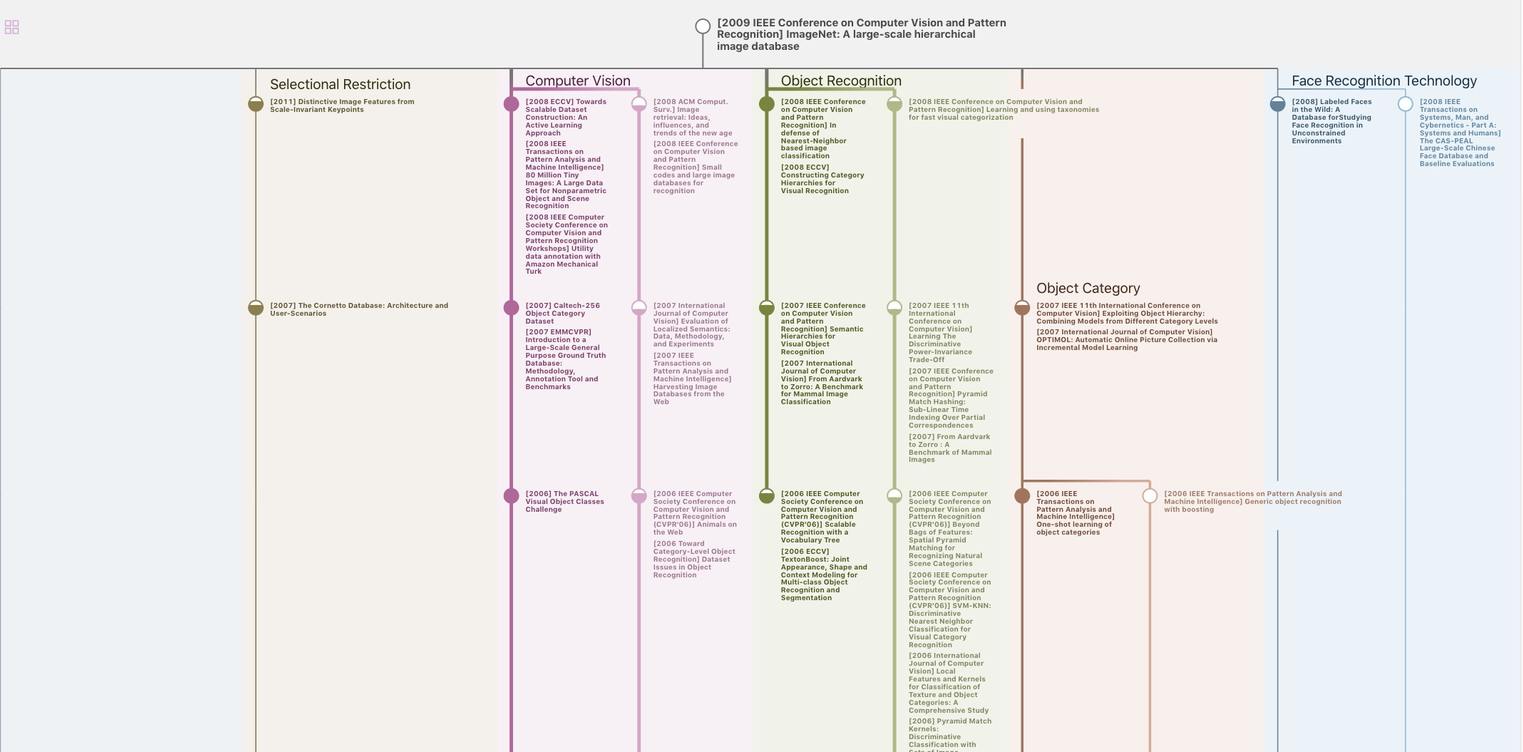
生成溯源树,研究论文发展脉络
Chat Paper
正在生成论文摘要