Reconstructing unsaturated infiltration behavior with sparse data via physics-informed deep learning
COMPUTERS AND GEOTECHNICS(2024)
摘要
In this paper, we propose a novel framework, physics -informed deep learning (PIDL), which combines a set of data- and physics -driven modeling methods along with an uncertainty assessment technique, to solve the illposed inverse problems in unsaturated infiltration and make plausible moisture field predictions. Specifically, PIDL integrates three methods: physics -informed neural network (PINN), multi -fidelity PINN (MF-PINN), and Monte Carlo dropout (MC -dropout). Firstly, we accurately predict the unsaturated infiltration behaviors using a PINN model, based on the Richards equation (RE) and a specific set of sparse and noisy observation data. Besides, in the presence of undetermined parameters within the soil-water characteristic curve, it is plausible to simultaneously ascertain those parameters. Subsequently, in cases where the available high-fidelity (HF) observation data are excessively sparse, the MF-PINN method can serve as an alternative to the PINN method for accurately predicting infiltration behavior by assimilating a certain quantity of easily accessible lowfidelity (LF) data. Finally, we apply the MC -dropout to investigate the uncertainty of the PINN and MF-PINN predicted results, and provide the corresponding credible intervals. We demonstrate the PIDL's efficacy with three unsaturated infiltration models and an on -site drainage case. This study offers a fresh perspective on addressing the inverse problems of unsaturated infiltration.
更多查看译文
关键词
Data-centric geotechnics,Unsaturated infiltration,Uncertainty model,Multi-fidelity,Physics-informed deep learning
AI 理解论文
溯源树
样例
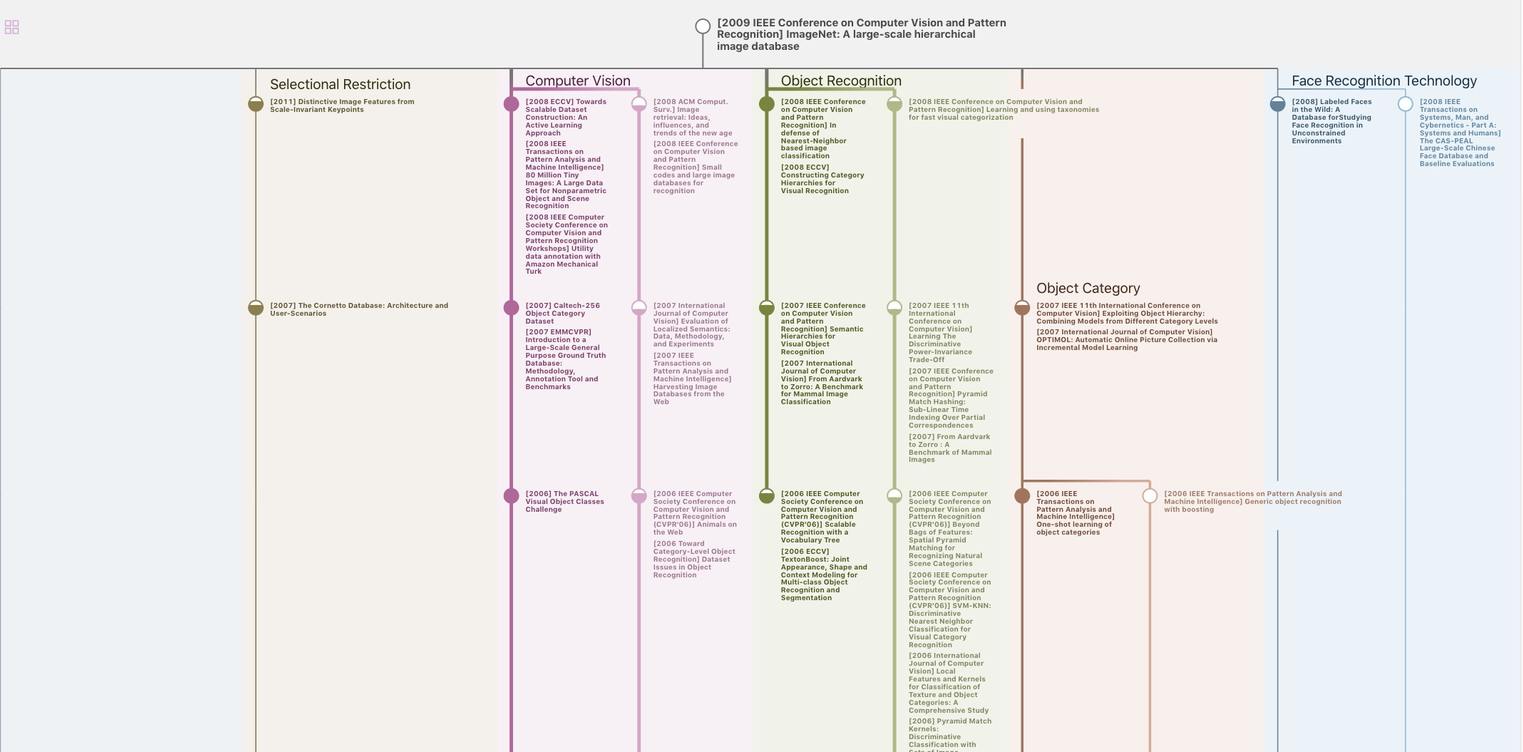
生成溯源树,研究论文发展脉络
Chat Paper
正在生成论文摘要