Deep neural networks can differentiate thyroid pathologies on infrared hyperspectral images
COMPUTER METHODS AND PROGRAMS IN BIOMEDICINE(2024)
摘要
Background and objective: The thyroid is a gland responsible for producing important body hormones. Several pathologies can affect this gland, such as thyroiditis, hypothyroidism, and thyroid cancer. The visual histological analysis of thyroid specimens is a valuable process that enables pathologists to detect diseases with high efficiency, providing the patient with a better prognosis. Existing computer vision systems developed to aid in the analysis of histological samples have limitations in distinguishing pathologies with similar characteristics or samples containing multiple diseases. To overcome this challenge, hyperspectral images are being studied to represent biological samples based on their molecular interaction with light. Methods: In this study, we address the acquisition of infrared absorbance spectra from each voxel of histological specimens. This data is then used for the development of a multiclass fully-connected neural network model that discriminates spectral patterns, enabling the classification of voxels as healthy, cancerous, or goiter. Results: Through experiments using the k -fold cross -validation protocol, we obtained an average accuracy of 93.66 %, a sensitivity of 93.47 %, and a specificity of 96.93 %. Our results demonstrate the feasibility of using infrared hyperspectral imaging to characterize healthy tissue and thyroid pathologies using absorbance measurements. The proposed deep learning model has the potential to improve diagnostic efficiency and enhance patient outcomes.
更多查看译文
关键词
Computer-aided diagnosis,Deep learning,Hyperspectral imaging,Computational pathology,Thyroid cancer
AI 理解论文
溯源树
样例
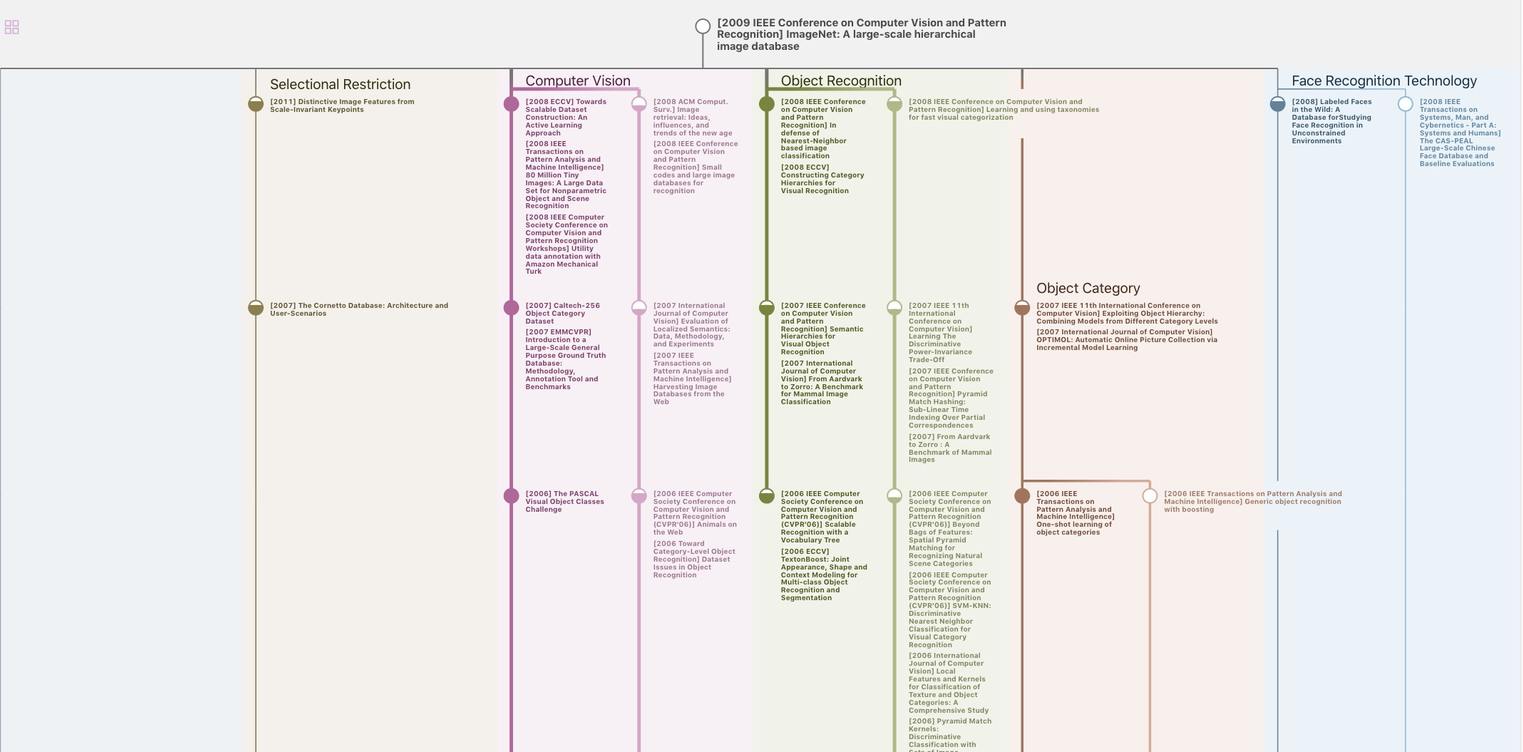
生成溯源树,研究论文发展脉络
Chat Paper
正在生成论文摘要