Deep parametric Retinex decomposition model for low-light image enhancement
COMPUTER VISION AND IMAGE UNDERSTANDING(2024)
摘要
Images captured under low light conditions often suffer from various degradations. The Retinex models are highly effective in enhancing low -light images. The analytical optimization models are interpretable but inflexible to various scenes. The data -driven learning models are flexible to various scenes but less interpretable. To reconcile the advantages of both, we propose a parametric Retinex model with pixel -wise varying parameters. Then we unroll its iterative algorithm into an unfolding network so that the parameters can be learned. We call it Deep Parametric REtinex Decomposition (DPRED). Based on the Retinex decomposition, we present a novel network for low -light image enhancement, also called DPRED. The whole network comprises three modules: parametric Retinex decomposition, enhancement and refinement. The first two modules operate on the V channel in the HSV space, avoiding color deviation. The refinement module aims to remove noise in the enhanced RGB image. Extensive experiments demonstrate the proposed method is effective in low -light image enhancement and it significantly outperforms recent baselines.
更多查看译文
关键词
Low-light image enhancement,Parametric Retinex decomposition,Deep network,Denoising
AI 理解论文
溯源树
样例
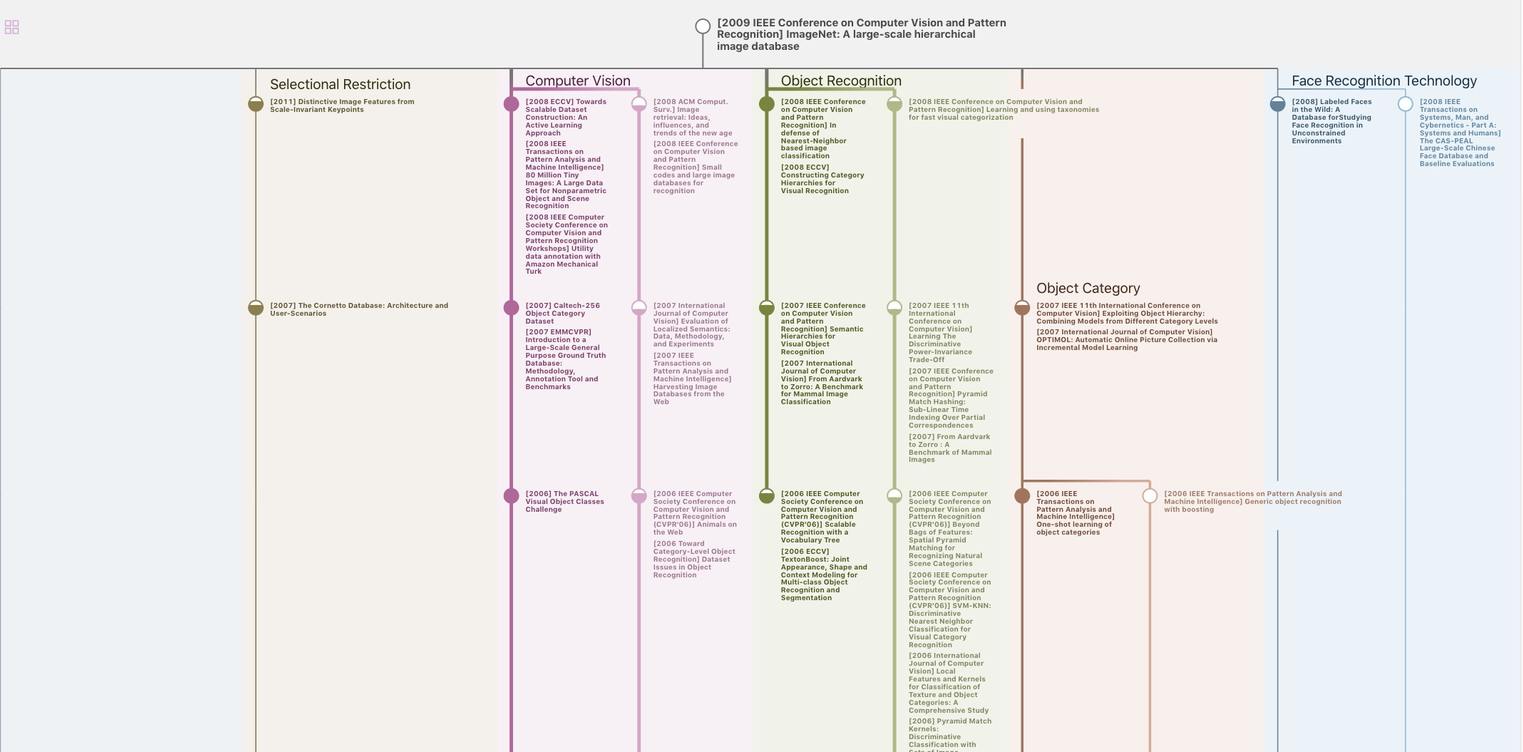
生成溯源树,研究论文发展脉络
Chat Paper
正在生成论文摘要