Machine learning controller for data rate management in science DMZ networks
COMPUTER NETWORKS(2024)
摘要
This article presents a Machine Learning Controller (MLC) supported by a P4 switch for improving rate control in non -dedicated Science Demilitarized Zone (Science DMZ) cyberinfrastructures. The proposed scheme utilizes passive data plane measurements such as Round Trip Time (RTT), throughput, queuing delay, and active flow count to regulate campus network output and achieve a desired Data Transfer Node (DTN) target rate. We evaluated our solution through a testbed using a bare -metal data plane switch, legacy router, and emulated hosts. Results show that including a rate controller based on data plane programmable devices on a nondedicated Science DMZ cyberinfrastructure can effectively improve the completion time of scientific big data flows, while having a low impact on the campus network traffic and bottleneck link utilization. Specifically, the proposed controller achieved an average improvement of 21.72% in Flow Completion Time (FCT) compared to a trivial fixed-rate solution when the DTN uses BBR2 as a Congestion Control Algorithm (CCA). The results highlight the potential of machine learning techniques in conjunction with data plane measurements for optimizing the performance of non -dedicated networks.
更多查看译文
关键词
Science DMZ,Machine learning control,Rate control,Programmable data planes,P4 language,Passive measurement
AI 理解论文
溯源树
样例
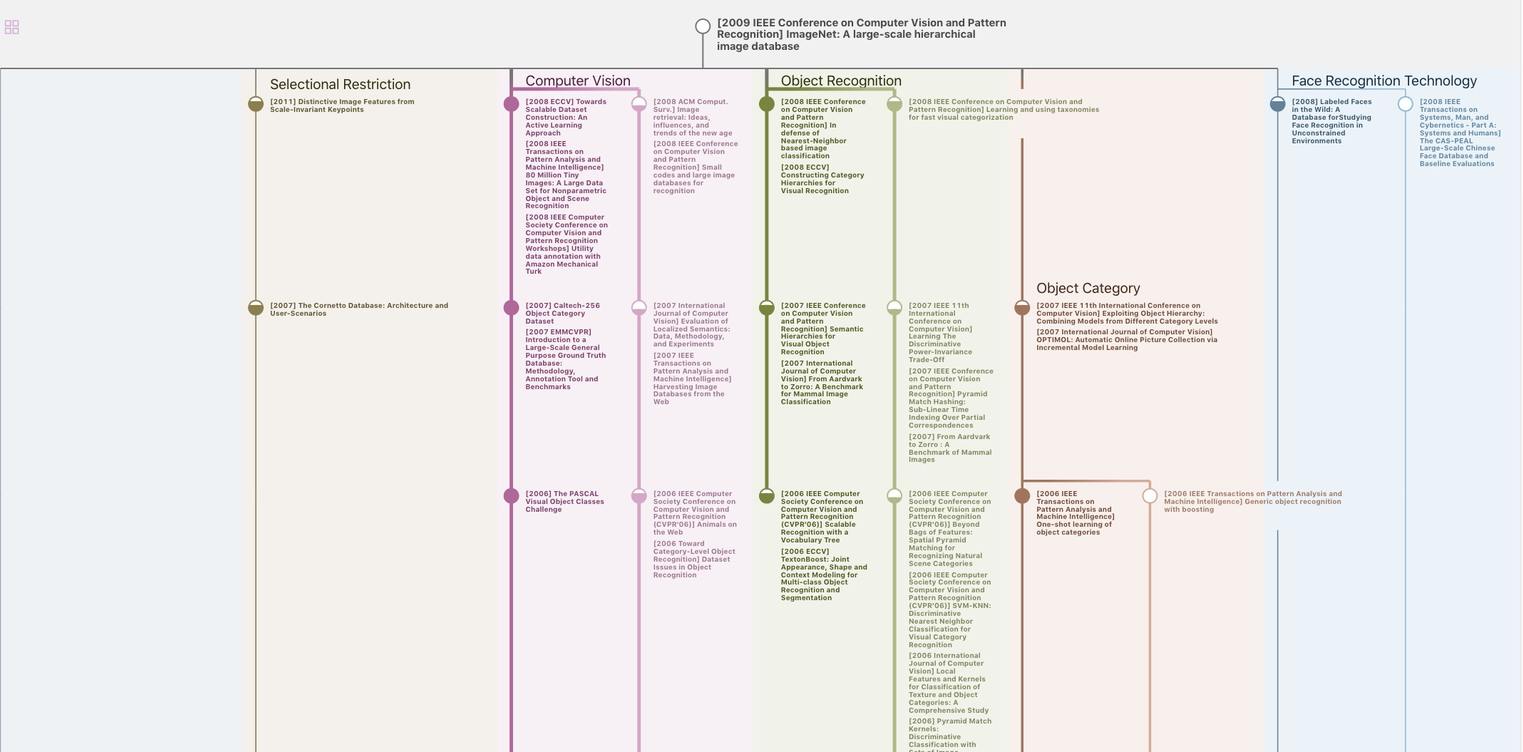
生成溯源树,研究论文发展脉络
Chat Paper
正在生成论文摘要