L2-BiTCN-CNN: Spatio-temporal features fusion-based multi-classification model for various internet applications identification
Computer Networks(2024)
摘要
Identifying various types of Internet applications is a key issue for network management. Traffic classification is one of the important technologies for identifying Internet applications traffic. However, some secure technologies such as protocol encryption, information hiding, and traffic obfuscation pose new challenges to traffic identification and classification, especially in feature extraction. In addition, multi-classification has always been a difficult problem in traffic classification research. This paper proposes a spatio-temporal features fusion-based multi-classification model, named as L2-BiTCN-CNN. Our approach combines bidirectional temporal convolutional network (TCN) and convolutional neural network (CNN) to create an enhanced model. The L2-BiTCN model utilizes a bidirectional approach to learn both forward and backward sequence features of traffic, with a focus on the hierarchical structure of network traffic known as “byte-packet-session flow”. To fully extract timing characteristics between adjacent bytes and packets, this model incorporates attention mechanisms for both “byte hierarchy” and “packet hierarchy”. Filters of various sizes are utilizes in the CNN stage to capture packet features of different ranges. The spatial features of the packets are then obtained through two convolution and average pooling processes. The results of the experiments provide evidence of the effectiveness and superiority of our approach. Our model achieved an impressive overall accuracy of 99.839% and 97.186% in two public available datasets, namely USTC-TFC2016 and ISCX VPN-nonVPN2016, respectively. It outperforms the state-of-the-art models in terms of classification performance, which suggests that the model has the potential to be a valuable tool in various applications that require high accuracy.
更多查看译文
关键词
Internet applications identification,Traffic classification,Temporal convolutional network,Convolutional neural network,Attention mechanism
AI 理解论文
溯源树
样例
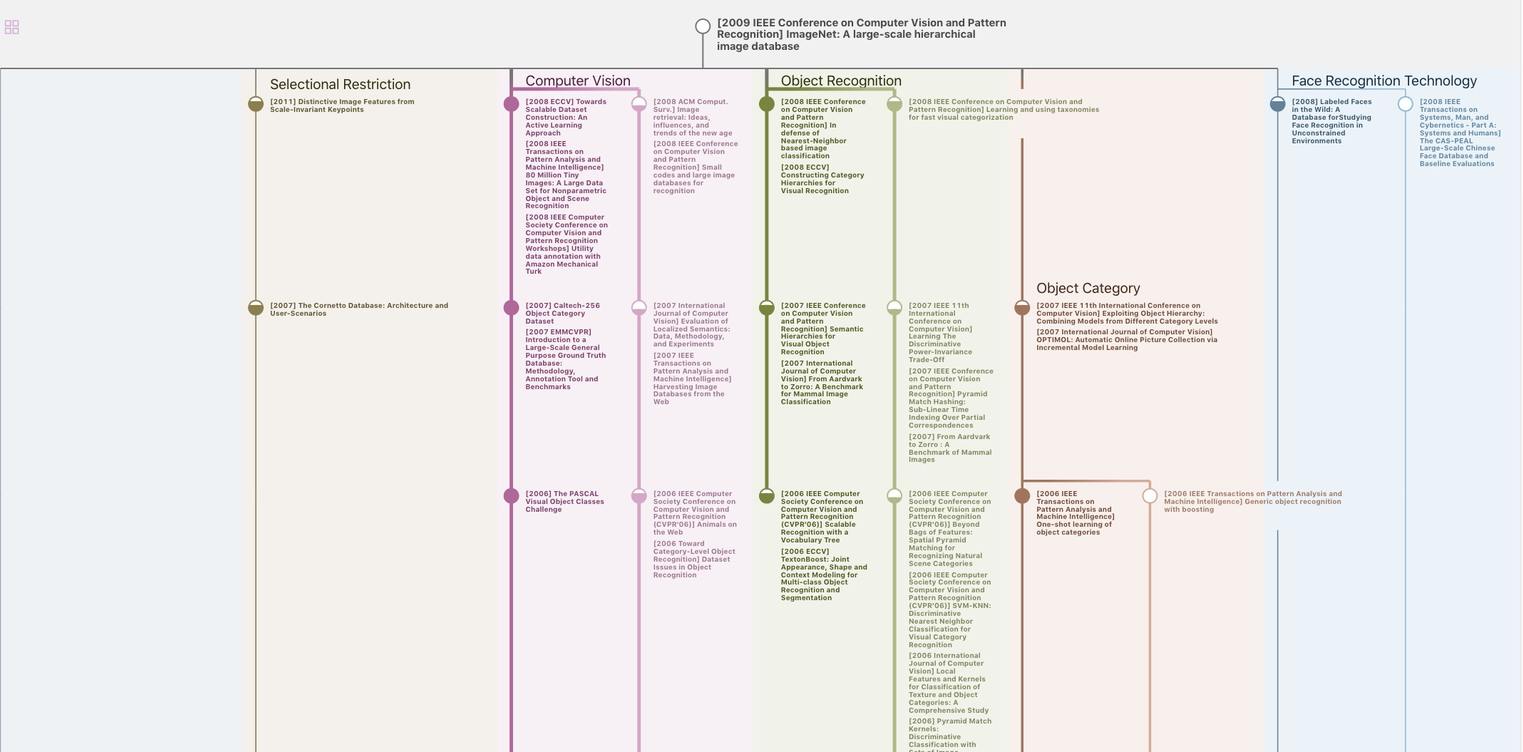
生成溯源树,研究论文发展脉络
Chat Paper
正在生成论文摘要