Machine learning and molecular design algorithm assisted discovery of gas separation membranes exceeding the CO2/CH4 and CO2/N2 upper bounds
CHEMICAL ENGINEERING SCIENCE(2024)
摘要
Designing polymers with desirable properties for gas separation membranes in a large chemical space is challenging. To address this issue, a framework combining machine learning (ML) and Bayesian-based molecular design algorithm is proposed to assist the discovery of polymers for gas separation membranes. In this study, explainable ML models for predicting gas permeability in polymers are developed and used to investigate the relationship between chemical structure and gas permeability. The Bayesian-based molecular design algorithm is further used to explore new polymers with properties above the current CO2/CH4 and CO2/N2 upper bounds. Hundreds of promising polymers with excellent CO2/CH4 and CO2/N2 separation performance are identified. Molecular dynamics (MD) simulations are used to calculate the gas permeabilities of the polymer candidates, providing additional support for the ML-predicted gas separation properties of polymer candidates. The polymer design framework presented in this work advances the discovery of polymers for gas separation membranes.
更多查看译文
关键词
Machine learning,Gas separation,Membrane,Polymer,Robeson upper bound
AI 理解论文
溯源树
样例
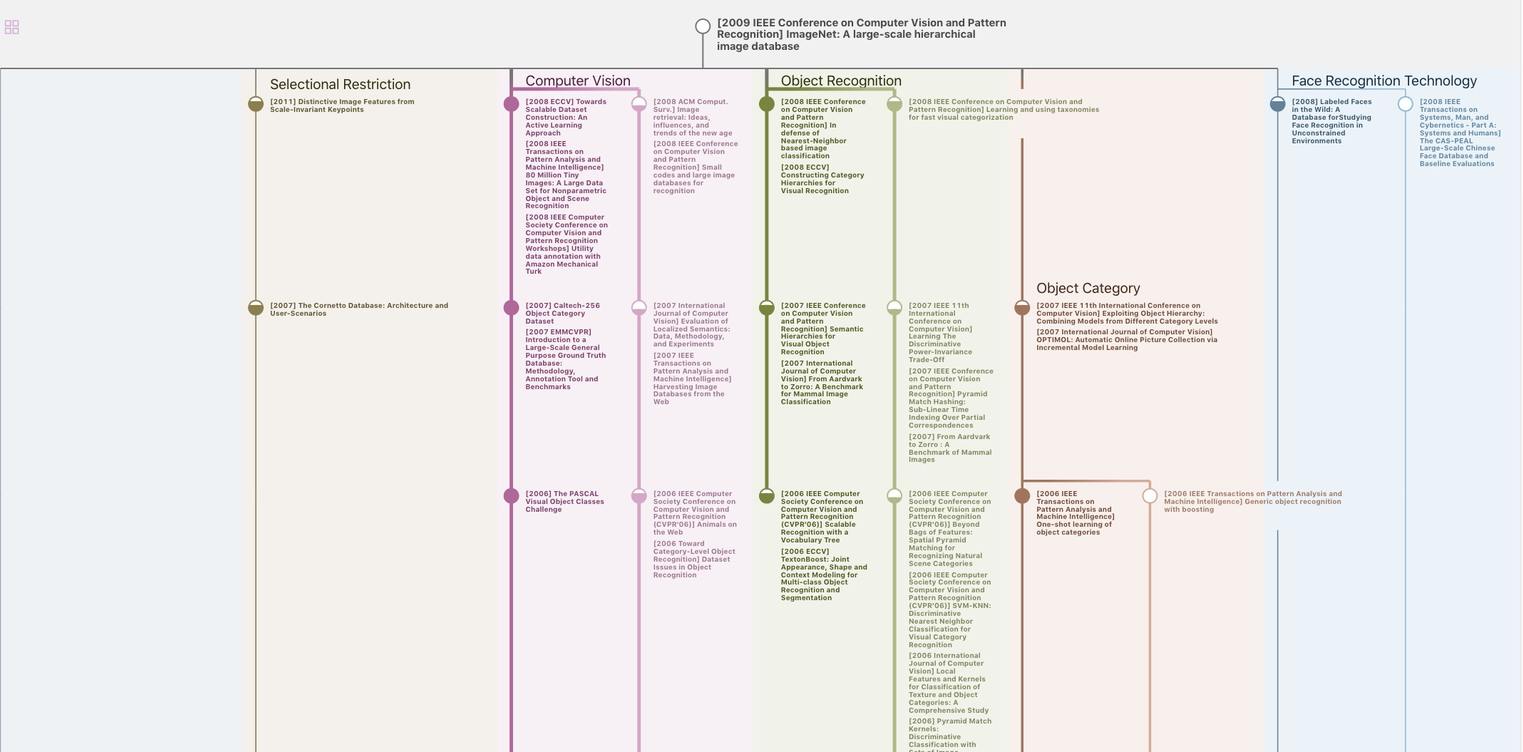
生成溯源树,研究论文发展脉络
Chat Paper
正在生成论文摘要