DMFF: Deep multimodel feature fusion for building occupancy detection
BUILDING AND ENVIRONMENT(2024)
摘要
The 2022 Global Status Report for Buildings and Construction (Buildings-GSR) indicates that construction activities have returned to pre -pandemic levels in most major economies, alongside more building energy consumption. To achieve the Net Zero emissions target by 2050, particularly in the post -pandemic era, accurate occupancy information is important to enhance building energy efficiency and improve occupancy comfort. While remarkable progress has been made in existing studies, they struggle to make full use of multi -sensor data to achieve high accuracy. Furthermore, there is a high expectation of the multimodel multi -temporary fusion by Transformer. In this study, we present a Transformer -based multimodal, multi -temporal feature fusion method (DMFF) for occupancy detection. To transfer domain knowledge from artificial intelligence into the building area, DMFF includes a pretrain-finetune pipeline and leverages pre -trained visual and sound models. Multiple pre -trained Transformer encoders are employed to extract features of different modalities. Then, we propose a self -attention mechanism for modality fusion to learn relationships among various sensors. Our DMFF method demonstrates superior performance on a real dataset, outperforming machine and deep learning methods (e.g., Convolutional Neural Networks, Random Forest, and Multilayer Perceptrons). Applied to a room setting, DMFF shows promising potential for building energy savings. The code and demo are accessible at https://github.com/kailaisun/multimodel_occupancy.
更多查看译文
关键词
Building occupancy detection,Audio-image pair,Transformer,Multimodel fusion
AI 理解论文
溯源树
样例
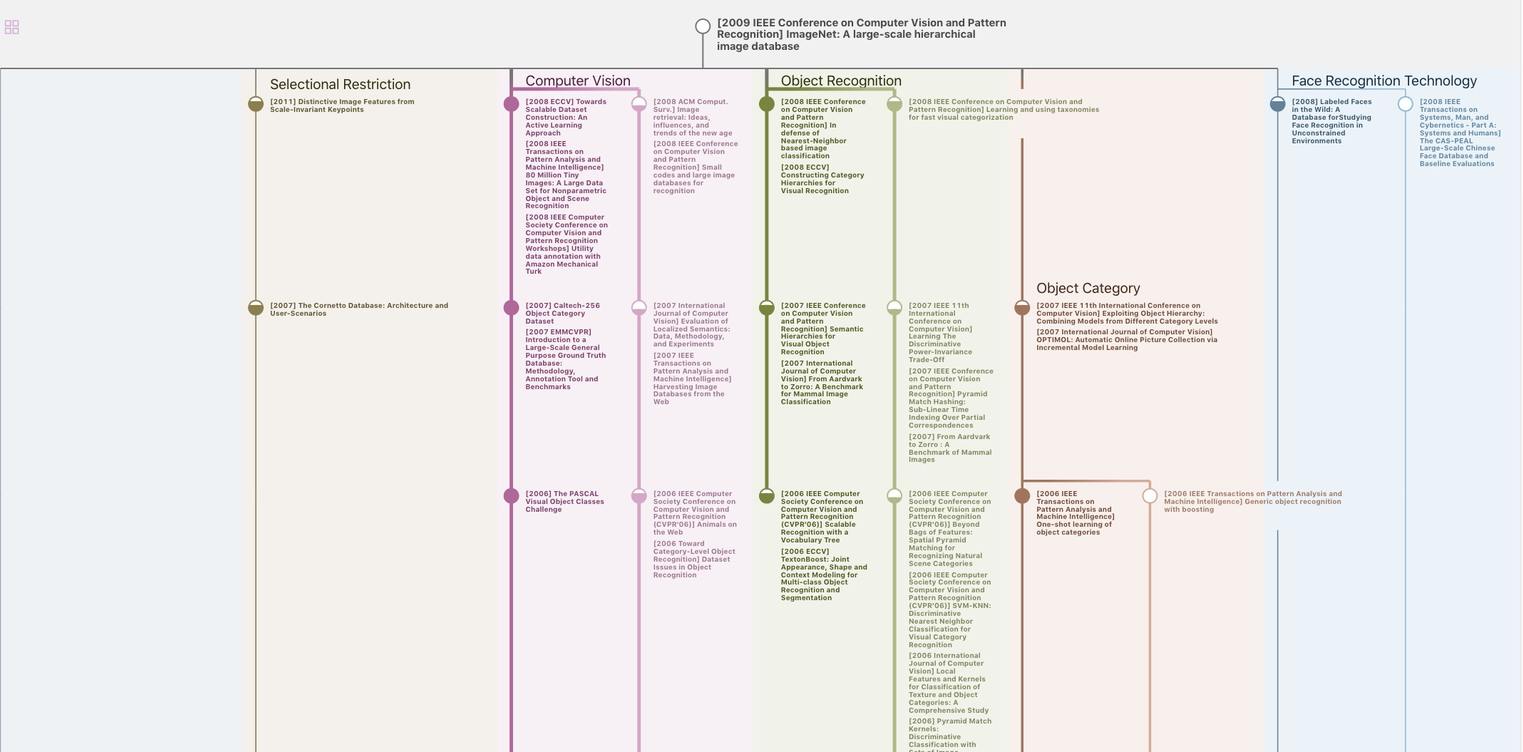
生成溯源树,研究论文发展脉络
Chat Paper
正在生成论文摘要