Transformer-based semantic segmentation and CNN network for detection of histopathological lung cancer
BIOMEDICAL SIGNAL PROCESSING AND CONTROL(2024)
摘要
The lungs are a very important organ in a human. Any abnormality in the lungs ultimately affects the whole body. Pulmonary nodules-initiated lung cancer that is very small in size initially. Lung cancer spreads in the whole body if it's not detected/treated at the start; therefore, detection of lung cancer at the earliest is mandatory for the survival of patients. In medical imaging, machine/deep learning methods are very useful for accurate and efficient detection of diseases. Therefore, two models are proposed for the segmentation and classification based on transformer/deep/machine learning. The segmentation model named TransSegNet is proposed in which input images are converted into [44] patches and embedded into four transformer blocks. The model is trained from scratch by using 10 epochs, 4 batch-size, and an Adam optimizer as selected hyperparameters. The model is evaluated on the ACDC-LungHP dataset that provides 99.62 % detection accuracy having 0.0067 loss, Mean IOU of 49.81, validation accuracy of 99.02 %, validation-loss of 0.0219, and validation mean IOU of 49.52. The minimum and maximum improvement in the results of the proposed segmentation model is +04.62 and +11.62 respectively as compared to existing methods. The second classification model named MinClassNet is proposed which contains seven layers including input, conv, ReLU, batch normalization, softmax, fully connected, and output. The model is also trained from scratch based on selected hyperparameters after extensive experimentation such as Adam optimizer, 8 batch size, and 10 epochs. The model performance is tested using a publically available histopathological lung cancer dataset which provided 98.39 % accuracy in classifying the healthy/ abnormal cells. The minimum and maximum improvement in the results of the proposed classification model are +1.29 and +20.06 respectively as compared to existing methods.
更多查看译文
关键词
Lung cancer,Artificial intelligence,Segmentation,Classification,Histopathology,Colon cancer,Nodules,Tumors
AI 理解论文
溯源树
样例
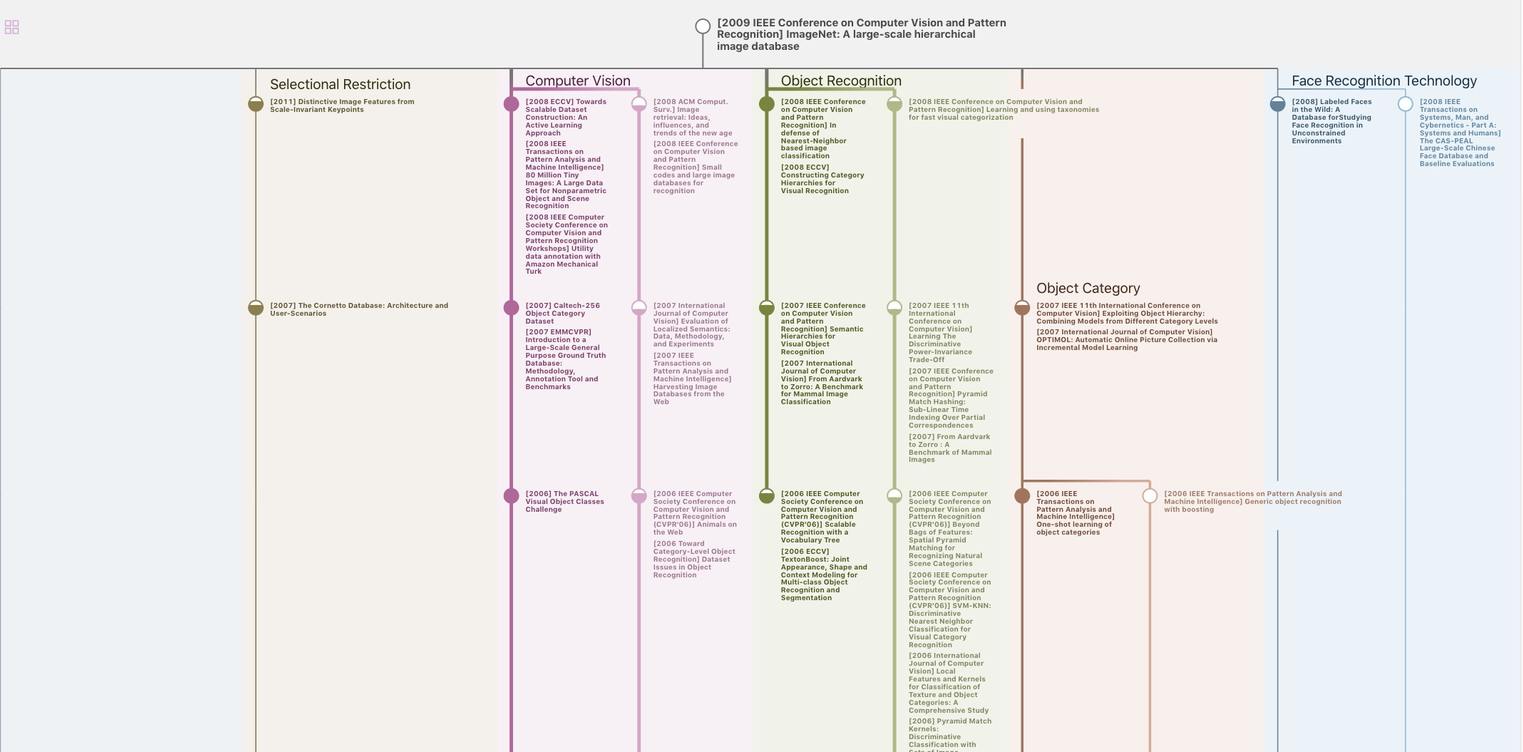
生成溯源树,研究论文发展脉络
Chat Paper
正在生成论文摘要