Enhancing the online estimation of finger kinematics from sEMG using LSTM with attention mechanisms
Biomedical Signal Processing and Control(2024)
摘要
Simultaneous and proportional estimation of human finger kinematics using muscle interface has gained significant attention for human-robot interaction. Most existing researches focused on proposing novel estimation methods to achieve high estimation accuracy and validated them offline. However, the gap between the online and offline estimation has not been fully considered which may lead to unpredictable deterioration or failure in practical application. This paper quantifies the gap between online and offline estimation performance and the underlying factors for such gap. The temporal variability of muscle activities represented by surface electromyography (sEMG) is found to be more significant in online estimation which challenges the estimation model in computation complexity and temporal feature extraction. To improve the online estimation accuracy, this paper proposes to combine an attention module with the long short-term memory (LSTM) network which enables not only the global but also the local key information of sEMG signals being focused. The finger kinematics of five primary degrees of freedom (DoFs) is estimated from the sEMG recordings during some grasping tasks. Compared with the sole-LSTM model (CC=0.85,RMSE=9.41), the LSTM with attention mechanism (CC=0.92,RMSE=7.96) demonstrates an improvement in online estimation accuracy, presenting an effective way of kinematics decoding for human-robot interaction.
更多查看译文
关键词
Simultaneous and proportional estimation of kinematics,Surface electromyography (sEMG),LSTM with attention mechanism,Human-robot interaction
AI 理解论文
溯源树
样例
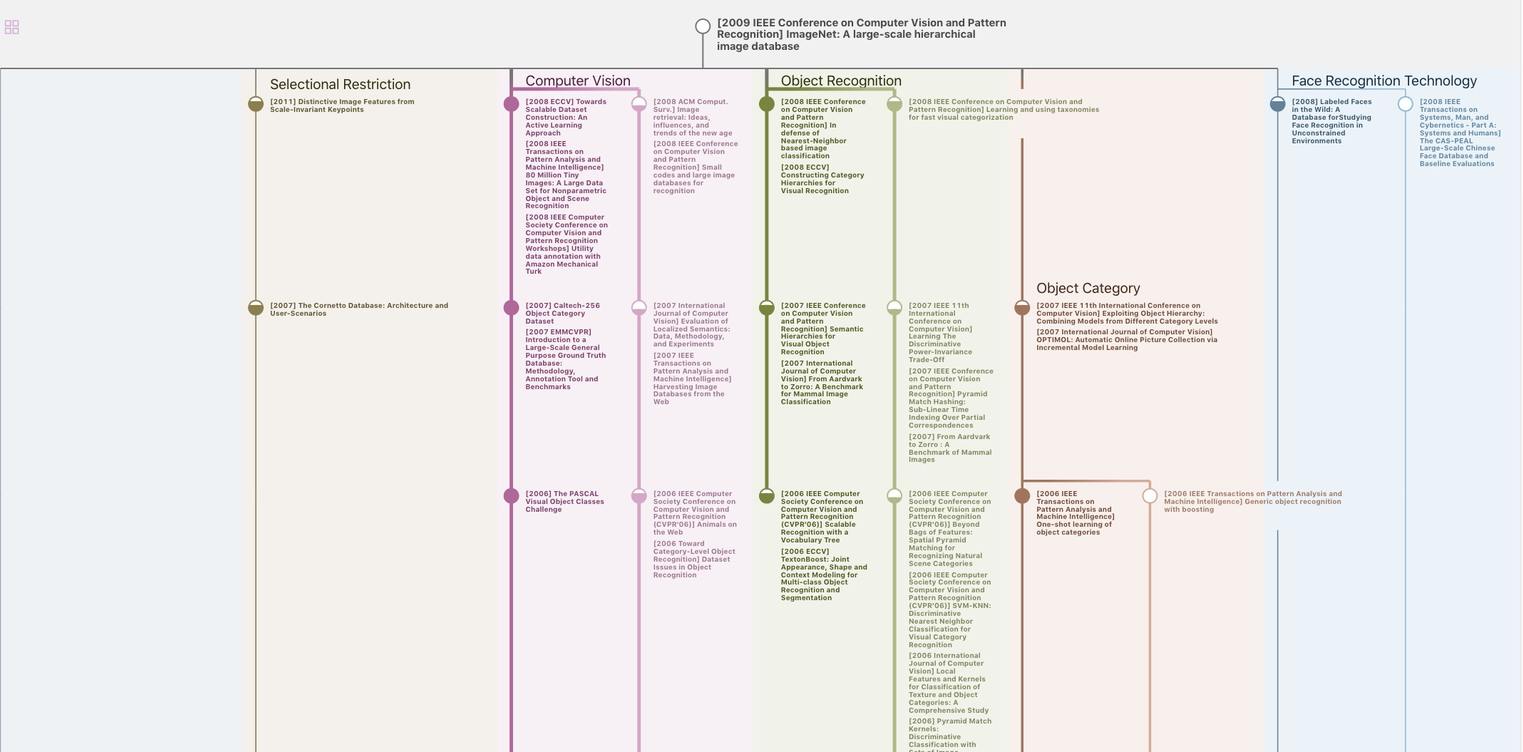
生成溯源树,研究论文发展脉络
Chat Paper
正在生成论文摘要