Shift-invariant rank-(L, L, 1, 1) BTD with 3D spatial pooling and orthonormalization: Application to multi-subject fMRI data
BIOMEDICAL SIGNAL PROCESSING AND CONTROL(2024)
摘要
The rank-(L, L, 1, 1) block term decomposition (BTD) model shows better separation performance for multisubject fMRI data by preserving the high-way structure of fMRI data than canonical polyadic decomposition (CPD). However, multi-subject fMRI data are noisy and have high spatiotemporal variability. To address these limitations, this paper proposes a novel 3D weighted spatial pooling preprocessing that compresses and smooths multi-subject fMRI data and assigns a higher weight to in-brain voxels but a lower weight to out-brain voxels. This strategy not only largely reduces the size of spatial images but also improves the robustness to noise. Furthermore, to address the high spatiotemporal variability, the rank-(L, L, 1, 1) BTD model of the reduced fMRI data is relaxed by incorporating temporal shift-invariance and spatial orthonormality constraints to extract pooled multi-subject shared spatial maps, shared time courses, subject-specific time delays and intensities. Finally, multi-subject intact shared spatial maps are obtained based on shift-invariant rank-(L, L, 1, 1) BTD of intact fMRI data. The simulated and experimental fMRI data experiments both verify that the proposed method achieves better separation performance and stronger robustness to noise than rank-(L, L, 1, 1) BTD with a spatial orthonormality constraint and a method combining independent component analysis and shift-invariant CPD. Moreover, the proposed method with 3D spatial pooling yields better separation performance than that with 2D spatial pooling, because 3D spatial pooling preserves refined voxels, thereby retaining more information of adjacent slices.
更多查看译文
关键词
Block term decomposition (BTD),Spatiotemporal variability,Weighted spatial pooling,Orthonormality,Shift-invariance,Tensor decomposition
AI 理解论文
溯源树
样例
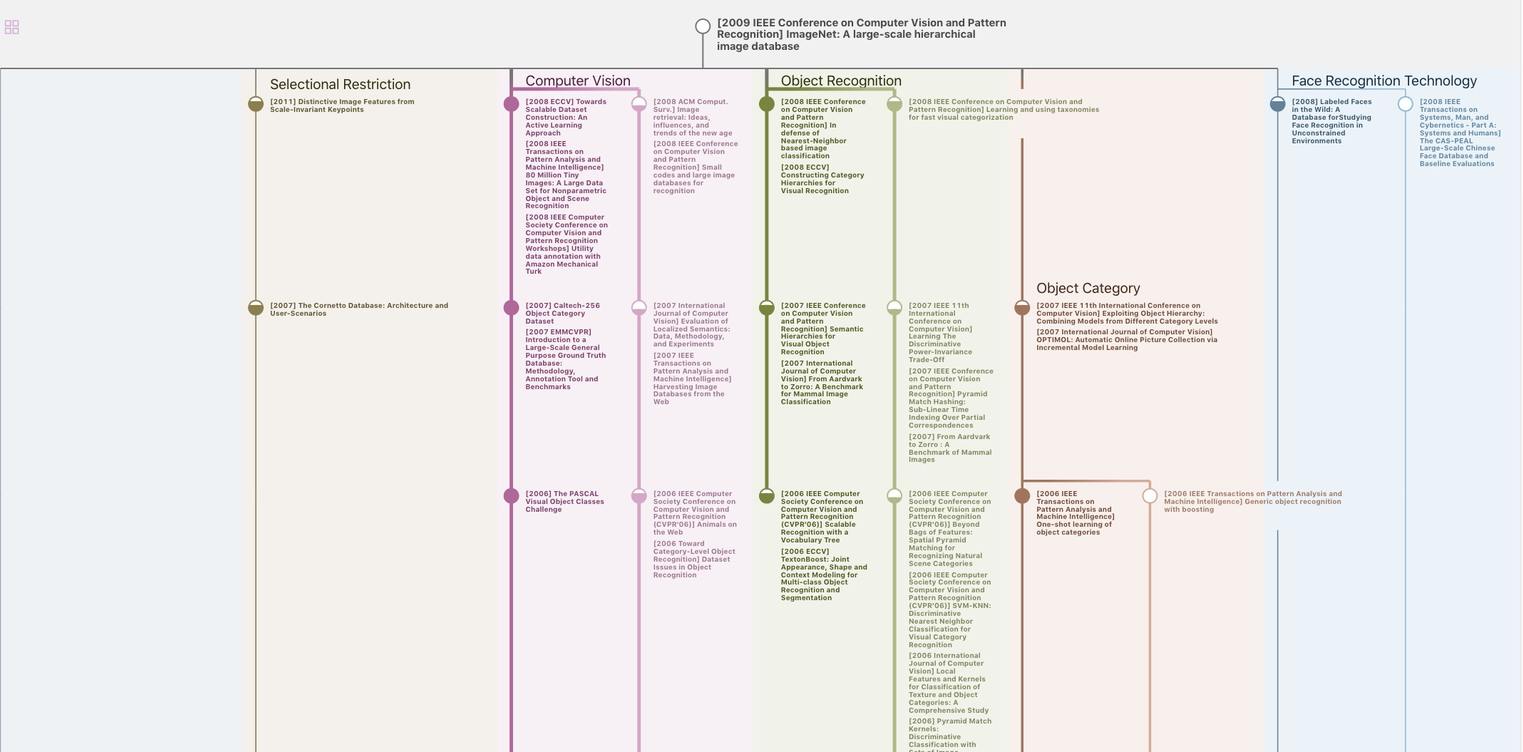
生成溯源树,研究论文发展脉络
Chat Paper
正在生成论文摘要