Second-order difference scatterplot-based transition network with Riemann similarity measure for epilepsy classification
BIOMEDICAL SIGNAL PROCESSING AND CONTROL(2024)
摘要
Epilepsy is a neurological condition affecting approximately 70 million individuals globally. It is marked by recurring seizures resulting from irregular brain electrical activity. Nonetheless, due to the significant variability and complexity of EEG signals, achieving dependable seizure classification remains a formidable endeavor. This paper presents a novel approach to classify epilepsy by examining epileptic EEG using a transition network founded on second-order difference scatterplot and employing a Riemann similarity measure. The approach employs a Riemann similarity measure to discern distinctions among diverse categories of time series data. This study's contributions encompass the introduction of an innovative second-order difference scatterplot, harnessing the attributes of second-order difference to enhance sensitivity to abrupt signals within local regions, its application in mapping organized patterns within time-series data, and the projection of a transition network's adjacency matrix onto a Riemannian manifold, whereby its kinetic resemblance is gauged through Riemannian distance comparison. Notably, this method attains highly commendable classification outcomes in both simulated and empirical datasets, particularly excelling in the classification of preictal and interictal phases.
更多查看译文
关键词
Nonlinear time series analysis,Second -order difference scatterplot,Ordinal partition transition network,Riemann similarity measure,Epilepsy classification
AI 理解论文
溯源树
样例
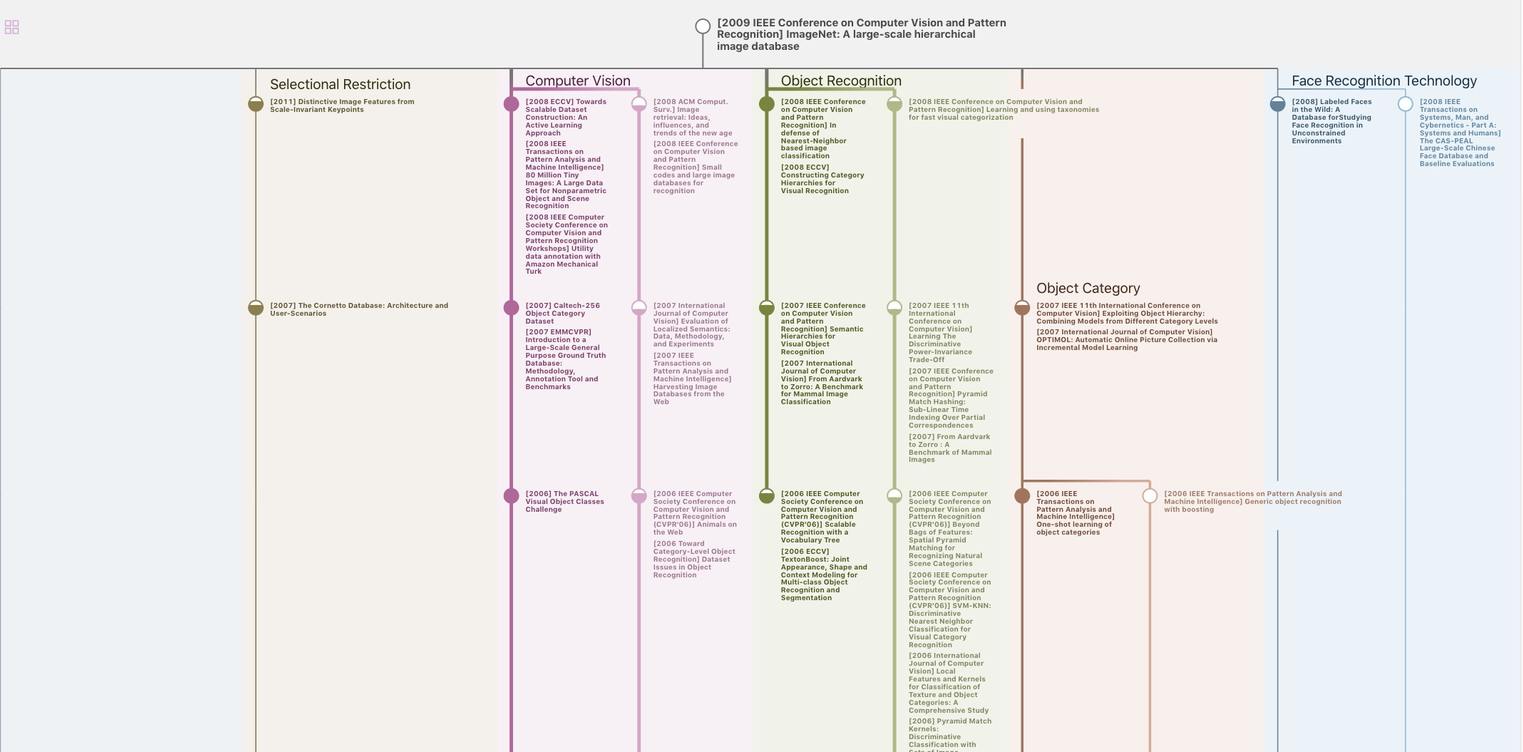
生成溯源树,研究论文发展脉络
Chat Paper
正在生成论文摘要