Ensemble learning for multi-channel sleep stage classification
Biomedical Signal Processing and Control(2024)
摘要
Sleep is a vital process for human well-being. Sleep scoring is performed by experts using polysomnograms, that record several body activities, such as electroencephalograms (EEG), electrooculograms (EOG), and electromyograms (EMG). This task is known to be exhausting, biased, time-consuming, and prone to errors. Current automatic sleep scoring approaches are mostly based on single-channel EEG and do not produce explainable results. Therefore, we propose a heterogeneous ensemble learning-based approach where we combine accuracy-based learning classifier systems with different algorithms to produce a robust, explainable, and enhanced classifier. The efficiency of our approach was evaluated using the Sleep-EDF benchmark dataset. The proposed models have reached an accuracy of 89.2% for the stacking model and 87.9% for the voting model, on a multi-class classification task based on the R&K guidelines.
更多查看译文
关键词
Sleep scoring,Ensemble learning,Learning classifier systems,Multi-signals
AI 理解论文
溯源树
样例
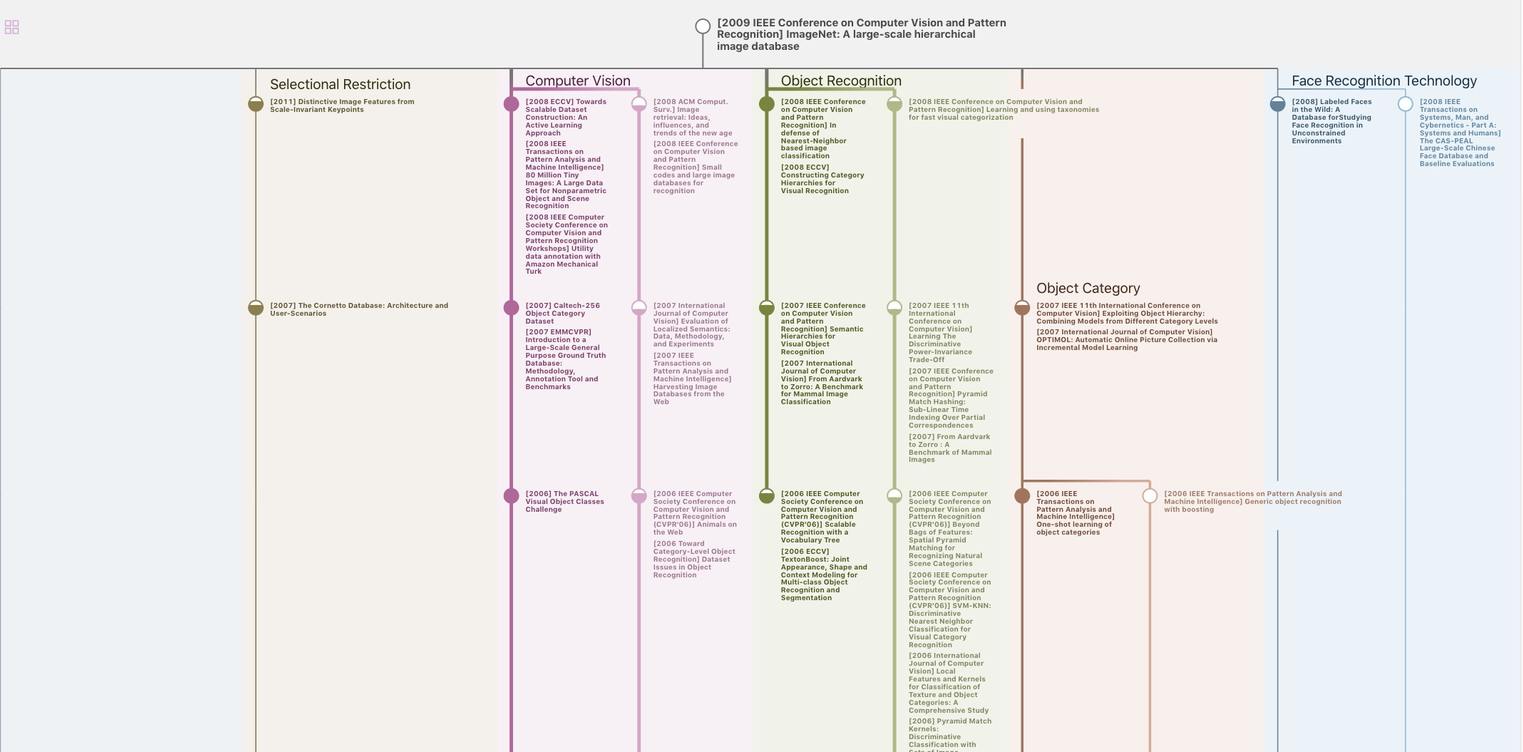
生成溯源树,研究论文发展脉络
Chat Paper
正在生成论文摘要