Grid-tuned ensemble models for 2D spectrogram-based autism classification
BIOMEDICAL SIGNAL PROCESSING AND CONTROL(2024)
摘要
Background: Autism spectrum disorder (ASD) is a prevalent collection of developmental disorders that affects around one in every 36 children worldwide. Since autistic children exhibit aberrant electroencephalogram (EEG) signals in comparison to typically developing (TD) children, many authors have recently proposed several machine learning (ML) and deep learning (DL) models to classify ASD patients using EEG signals. However, these models lack extensive and large-scale feature extraction and apply multiple benchmark datasets. Furthermore, most of the proposed ML models need feature engineering to extract key features for classification. Method: This study proposed a DL-based weighted ensemble model to avoid the need for feature engineering. The proposed model is based on finding the best suitable combination of weights using a grid search strategy, treating the highest weighted model as the most accurate, and giving more weightage. The strength of the suggested technique involves extracting features from EEG recordings by transforming raw EEG into multi-channel twodimensional time-frequency maps (2D spectrograms) using the short-time Fourier transform (STFT). Furthermore, it applies two widely-researched public EEG datasets [i.e., the King Abdulaziz University (KAU) and the University of Sheffield (TUOS) databases] for performance comparison and better generalizability. Additionally, the performance of the proposed model has been compared with other well-known DL models, and our proposed straightforward, easily implemented models surpass these models in terms of accuracy and training time. Results: The suggested model yields an accuracy of 94.71% for ASD classification using the KAU dataset and 96.22% accuracy for utilizing the TUOS dataset. Conclusions: Ensembled models can efficiently enhance accuracy, stability, and reproducibility. The demonstrated finding solidifies the applicability of the weighted ensemble model to classify ASD patients utilizing EEG data. The suggested model might be used as a supplemental tool to assist neurologists in diagnosing ASD.
更多查看译文
关键词
Autism spectrum disorder,Electroencephalogram,Deep learning,Ensemble learning,Spectrograms,short-time Fourier transform
AI 理解论文
溯源树
样例
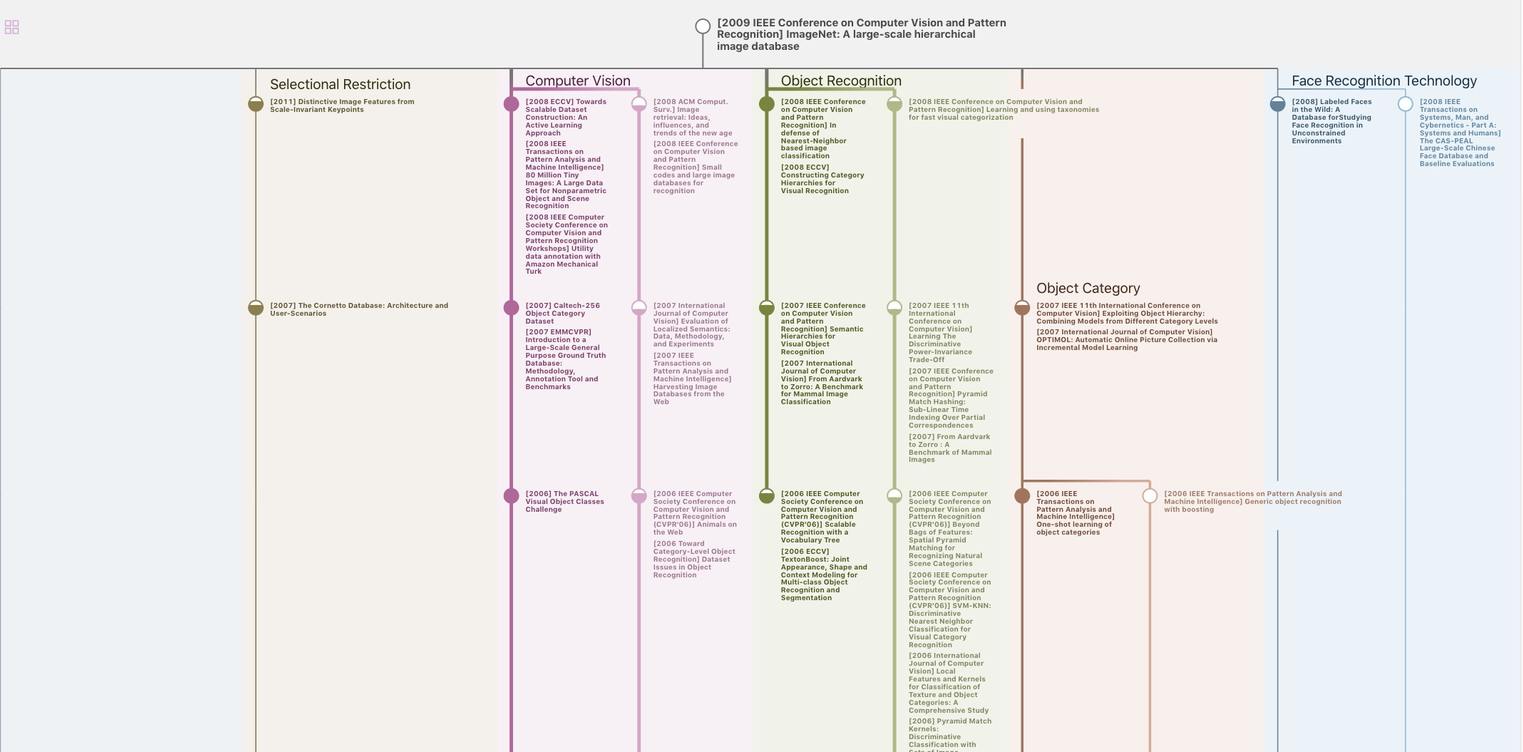
生成溯源树,研究论文发展脉络
Chat Paper
正在生成论文摘要