Scheduling optimization of electric ready mixed concrete vehicles using an improved model-based reinforcement learning
Automation in Construction(2024)
摘要
The decarbonization of the construction industry has emerged as a pressing global priority, and the electric machine is the driving force towards achieving this goal. This study focuses on developing an operation scheduling optimization methodology for electric ready-mixed concrete vehicles (ERVs), which is an innovative and environmental solution for the construction industry. Firstly, we propose a systematic problem definition for the ERV operation process that takes into account the unique properties of electric vehicles and RMC delivery tasks. Subsequently, the entire process is formulated as a Markov decision problem (MDP), which facilitates efficient sequential decision-making. Finally, we develop an improved model-based reinforcement learning method, named parallel-masked-decaying Monte Carlo Tree Search (PMD-MCTS), to solve the MDP efficiently. The entire system is validated in a real case study, and the performance of PMD-MCTS is compared with state-of-the-art benchmarks. The results have shown that the proposed MDP formulation is well-suited for addressing RMC delivery tasks. The PMD-MCTS algorithm and one of its ablation algorithms (PM-MCTS) have outperformed the other benchmarks in terms of either minimizing costs or delay, and PMD-MCTS consumes 32% less computation time than PM-MCTS.
更多查看译文
关键词
Electric vehicle, ready-mixed concrete delivery,Scheduling optimization,Model-based reinforcement learning,Monte Carlo tree search
AI 理解论文
溯源树
样例
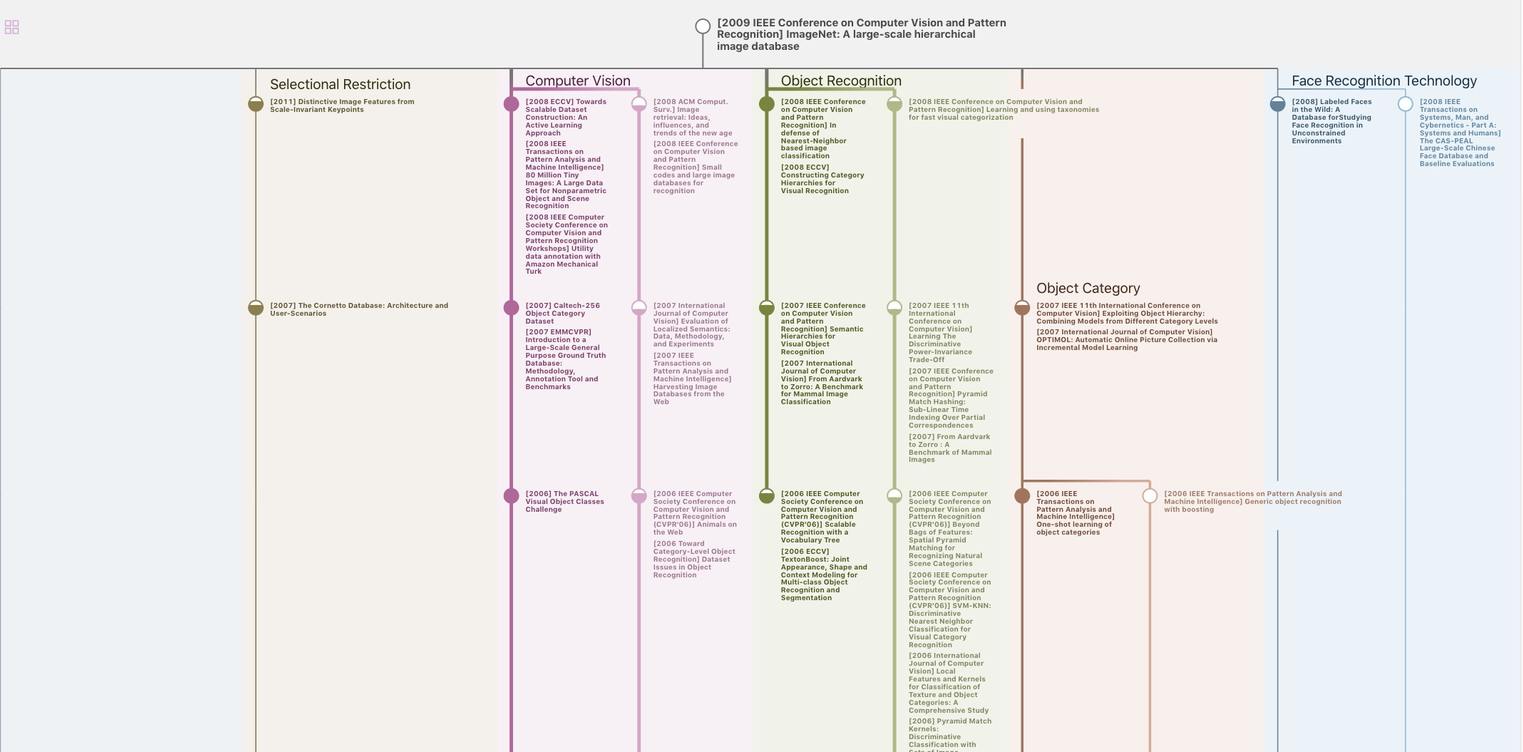
生成溯源树,研究论文发展脉络
Chat Paper
正在生成论文摘要