Model-informed deep learning-based compressive sensing for resampling and recovering 2D SHM vision data
AUTOMATION IN CONSTRUCTION(2024)
摘要
Long-term structural health monitoring (SHM) using vision -based methods may encounter challenges of data storage, low -sampling resolution, and data loss. Compressive sensing (CS) offers the possibility for alleviating these problems, by using less than half of the complete signal to recover the signal based on the sparsity. This paper proposed a model -informed deep learning -based CS method, named variable splitting scalable convolutional neural network (VSSNet). VSSNet includes a single block sampling convolution network, plus a hierarchical recovery network that contains one base layer (BL) and multiple enhancement layers (ELs). In each BL and EL, different data loss problems are solved using the variable splitting recovery network, by integrating optimization theories with deep learning -based CS methods. Then recoveries are refined by initial and deep recovery networks. Additionally, the greedy technique is used to select important sample bases, enabling resampling and recovery at different sampling rates using one model. The superiority of VSSNet was demonstrated through static and dynamic examples, showcasing its high accuracy and robustness in image recovery, structural motion estimation, and structural modal identification.
更多查看译文
关键词
Structural health monitoring,Video processing,Compressive sensing,Model-informed deep learning
AI 理解论文
溯源树
样例
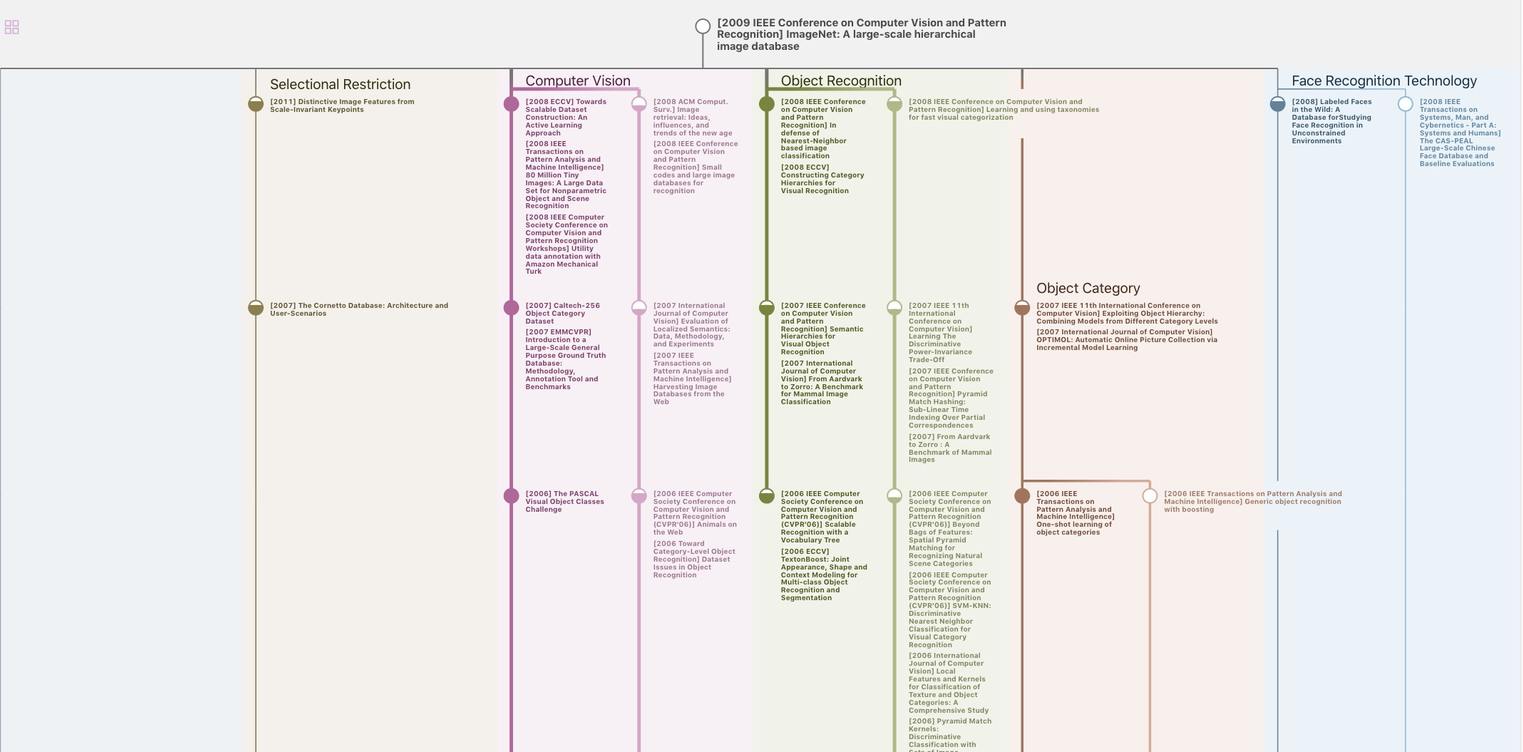
生成溯源树,研究论文发展脉络
Chat Paper
正在生成论文摘要