A dynamic feature selection-based data-driven quality prediction method for soft sensing in the diesel engine assembly system
Advanced Engineering Informatics(2024)
摘要
Establishing an accurate quality prediction model is an essential prerequisite for quality management in diesel engine manufacturing factories. However, the large production scale and non-linear relationship between features and the diesel engine power significantly aggravate the complexity of establishing a power prediction model. The identification of key features can not only improve the prediction performance but also facilitate the control of product quality. The mainstream feature selection methods estimate features on a whole static sampling space, which ignores dynamic correlation among candidate features, selected features, and the label variable. This paper focuses on the relevance between the candidate features and diesel engine quality, the complementary and redundancy between the candidate features and selected features, proposing a conditional mutual information-based dynamic feature selection method for the prediction of diesel engine quality. Then, a high-accuracy AdaBoost model is proposed with support vector regression-partial swarm optimization (SVR-PSO) as weak learners, showing a satisfactory non-linear fitting ability and a convenient parameter optimization process. Finally, several cases are performed to evaluate the effectiveness of the proposed methods. The proposed feature selection method shows a better performance than common feature selection methods on UCI datasets. Results on diesel engine power prediction declare that the proposed AdaBoost-SVR-PSO model is an effective quality prediction method compared with adaptive boosting, multilayer perception, and gradient boosting decision tree.
更多查看译文
关键词
Dynamic feature selection,AdaBoost-SVR-PSO,Diesel engine quality,Intelligent manufacturing
AI 理解论文
溯源树
样例
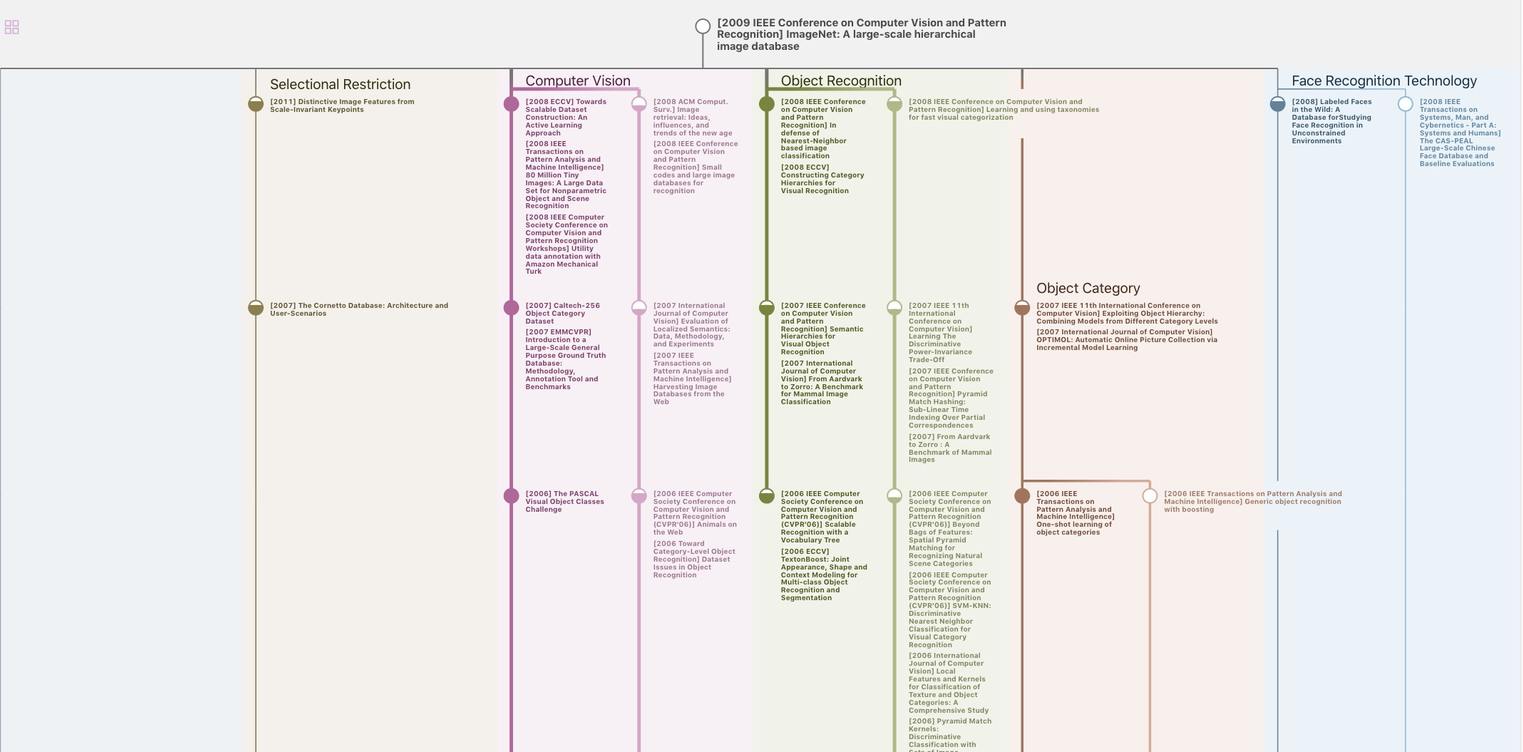
生成溯源树,研究论文发展脉络
Chat Paper
正在生成论文摘要