Multivariate reduced rank regression by signal subspace matching
SIGNAL PROCESSING(2024)
摘要
We present a tuning -free and computationally simple solution for multivariate reduced rank regression, based on the recently introduced signal subspace matching (SSM) metric. Unlike the existing solutions, which solve simultaneously for the rank and the value of the coefficient matrix, our solution decouples the two tasks. First, the rank of the coefficient matrix is determined using the SSM metric, and then the coefficient matrix is determined by ordinary least squares. We prove the consistency of the solution for the high signal -to -noiseratio limit, and also for the large -sample limit, conditioned on the noise being white. Experimental results, demonstrating the performance of the SSM solution, are included.
更多查看译文
关键词
Reduced-rank regression,Low-rank approximation,Rank determination,Soft projection,Signal subspace matching
AI 理解论文
溯源树
样例
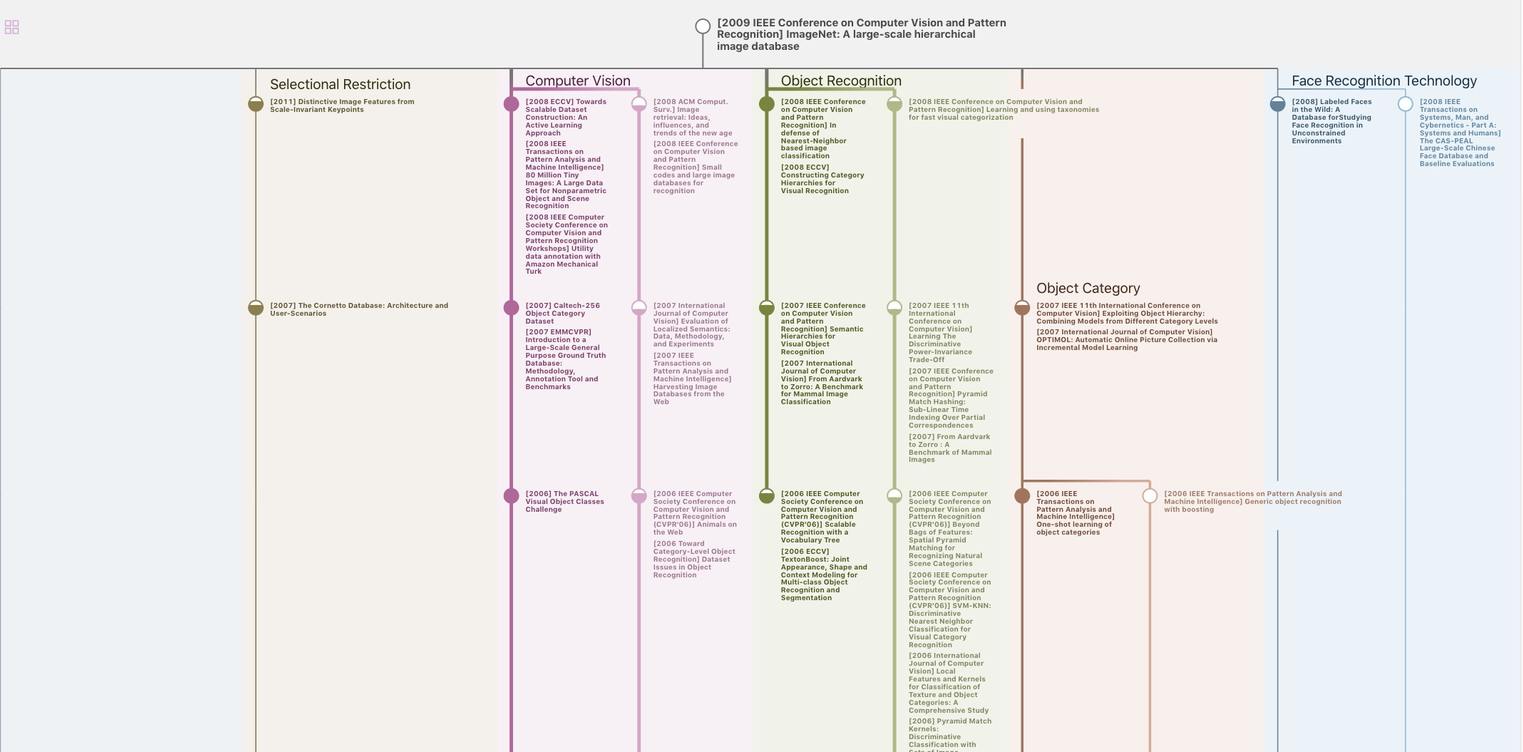
生成溯源树,研究论文发展脉络
Chat Paper
正在生成论文摘要