Semi-Bayesian active learning quadrature for estimating extremely low failure probabilities
RELIABILITY ENGINEERING & SYSTEM SAFETY(2024)
摘要
The Bayesian failure probability inference (BFPI) framework provides a sound basis for developing new Bayesian active learning reliability analysis methods. However, it is still computationally challenging to make use of the posterior variance of the failure probability. This study presents a novel method called 'semiBayesian active learning quadrature' (SBALQ) for estimating extremely low failure probabilities, which builds upon the BFPI framework. The key idea lies in only leveraging the posterior mean of the failure probability to design two crucial components for active learning - the stopping criterion and learning function. In this context, a new stopping criterion is introduced through exploring the structure of the posterior mean. Besides, we also develop a numerical integration technique named 'hyper -shell simulation' to estimate the analytically intractable integrals inherent in the stopping criterion. Furthermore, a new learning function is derived from the stopping criterion and by maximizing it a single point can be identified in each iteration of the active learning phase. To enable multi -point selection and facilitate parallel computing, the proposed learning function is modified by incorporating an influence function. Through five numerical examples, it is demonstrated that the proposed method can assess extremely small failure probabilities with desired efficiency and accuracy.
更多查看译文
关键词
Structural reliability analysis,Bayesian active learning,Stopping criterion,Learning function,Parallel computing
AI 理解论文
溯源树
样例
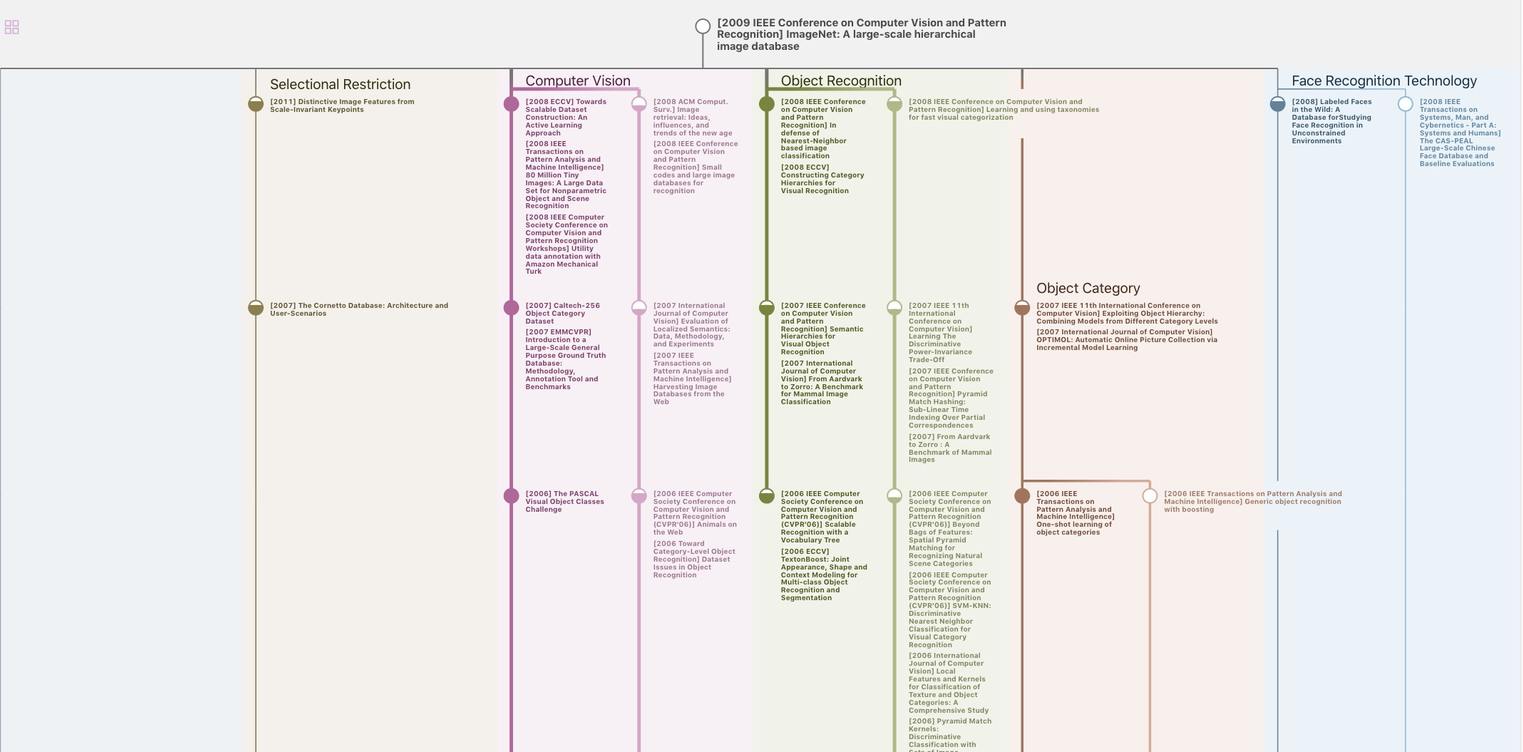
生成溯源树,研究论文发展脉络
Chat Paper
正在生成论文摘要