Embrace sustainable AI: Dynamic data subset selection for image classification
Pattern Recognition(2024)
摘要
Data selection is commonly used to reduce costs and energy usage by training on a subset of available data. However, determining the appropriate subset size requires extensive dataset knowledge and experimentation, limiting transferability. Varying the validation set also produces unstable results and wastes computational resources. In this paper, we propose a data selection method for dynamically determining subset ratios based on model performance using only a training set. The data search space is narrowed through weighted sampling, leveraging statistical selection patterns. Parallel analysis of class distributions identifies the most representative samples with high selection potential. Extensive experiments validate our approach and demonstrate improved training efficiency. Our method speeds up various subset ratios by up to 2.2x on CIFAR-10, 1.9x on CIFAR-100, 2.0x on TinyImageNet, and 2.1x on ImageNet with negligible accuracy drops.
更多查看译文
关键词
Data selection,Dynamic subset selection,Weighted sampling,Class distribution,Training efficiency
AI 理解论文
溯源树
样例
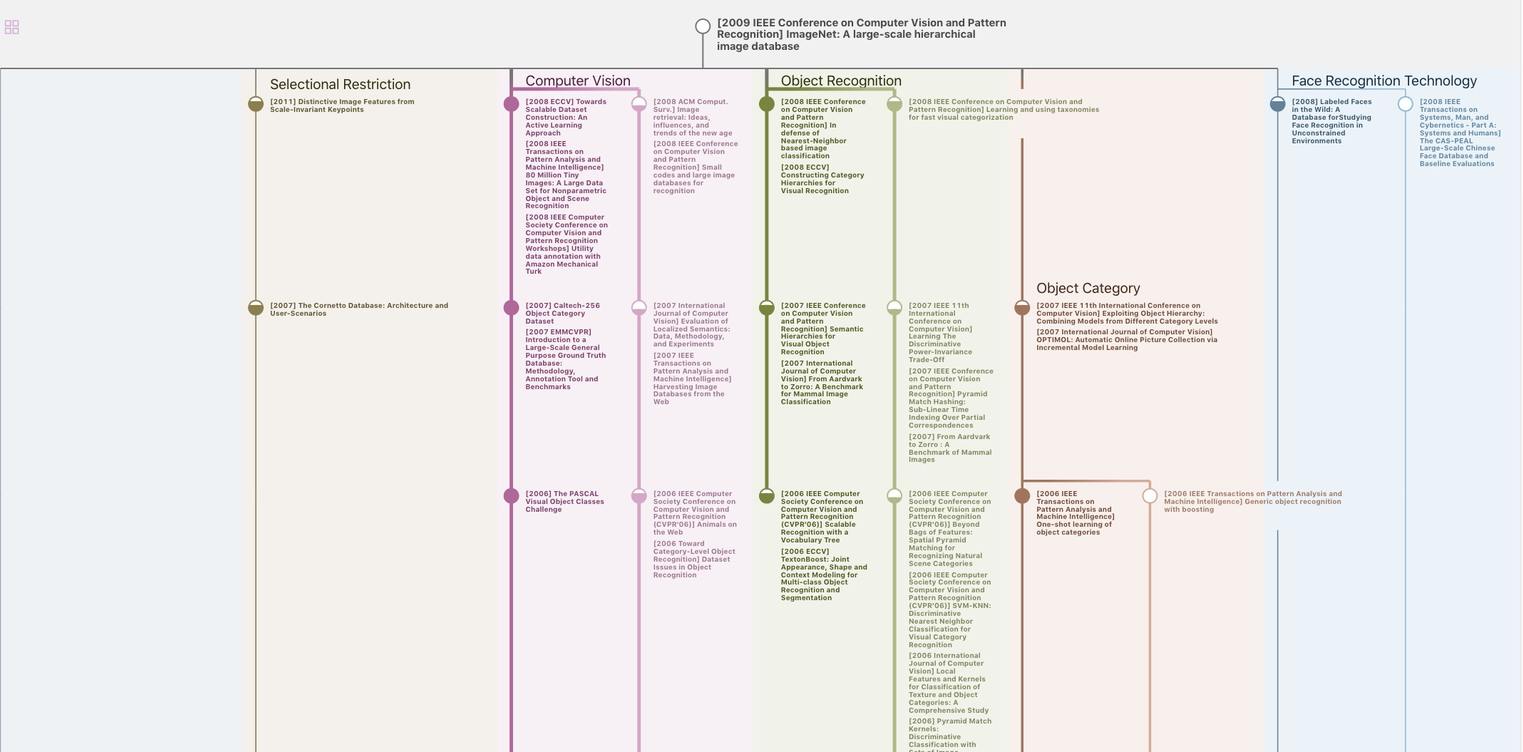
生成溯源树,研究论文发展脉络
Chat Paper
正在生成论文摘要