Data privacy protection health status assessment for rotating machinery with dual-task feature fusion framework
Neurocomputing(2024)
摘要
Accurately assessing health status of rotating machinery is essential in reliable operation and predictive maintenance. Recently, health status assessment (HSA) of rotating machinery has acquired significant progress, particularly with the increasing popularity of deep learning. However, most methods regard HSA as a combination of isolated tasks, ignoring the hierarchical relationships between tasks in predictive maintenance. Also, they fail to learn multi-source features adequately. Besides, due to data silos and conflict of interests, the collaborative model training poses a strong demand for privacy protection. Thus, this paper proposes a data privacy protection HSA framework with dual-task feature fusion learning. First, we consider HSA of machinery as an integral dual-task process and synchronously obtain assessment results. The proposed framework constructs hierarchical health labels to ensure assessment accuracy, and utilizes adaptive information exchange channels to maintain balance between the dual tasks. Next, we design a feature fusion network based on channel independence and patch mechanisms to enhance the utilization of correlation information in multi-source signals. Finally, by employing decentralized swarm learning, achieve privacy-preserving joint training to address data silos. Experimental results validate the effectiveness and superiority of the proposed framework.
更多查看译文
关键词
Rotating machinery,Health status assessment,Dual-task learning,Privacy protection,Feature fusion
AI 理解论文
溯源树
样例
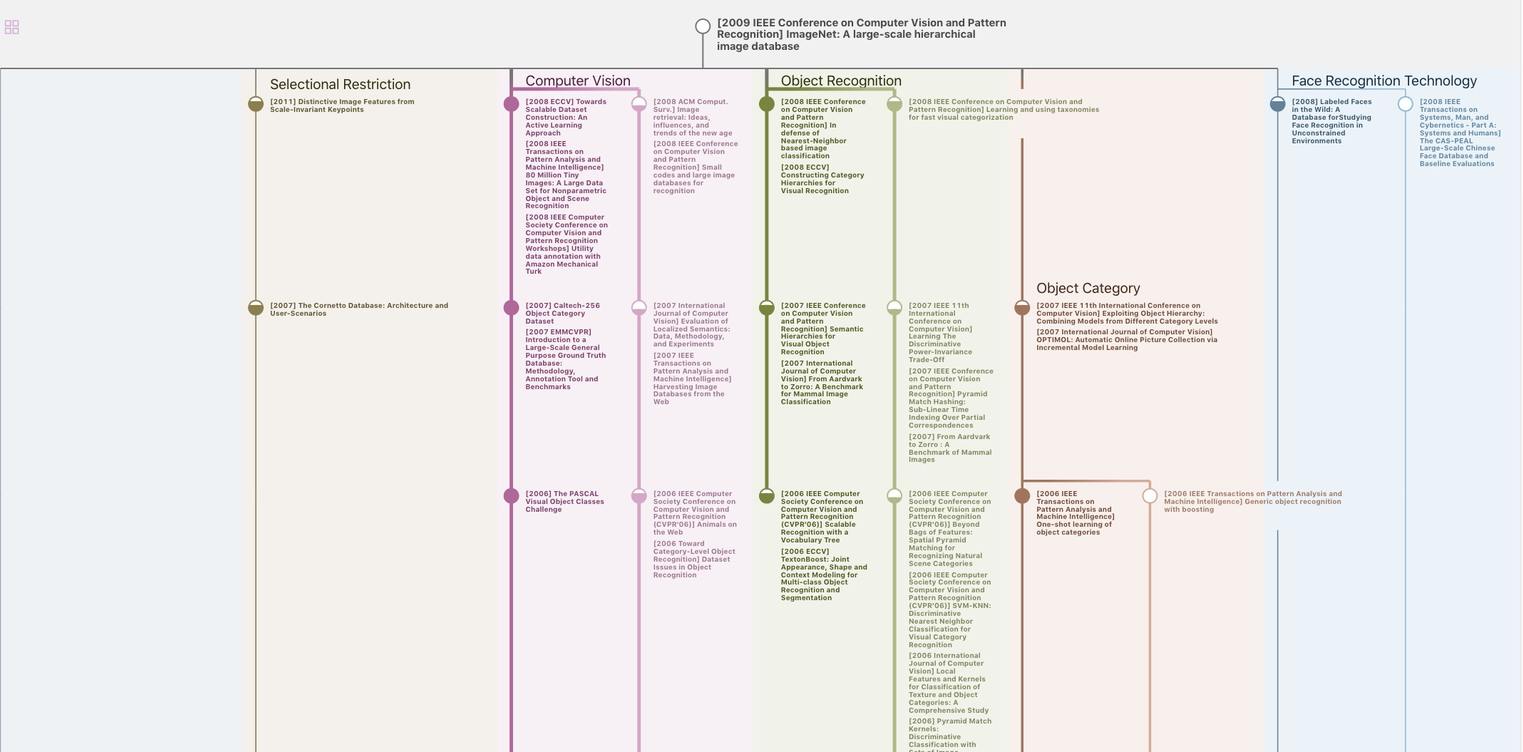
生成溯源树,研究论文发展脉络
Chat Paper
正在生成论文摘要