GLIMS: A Two-stage Gradual-learning Method for Cancer Genes Prediction Using Multi-omics Data and Co-splicing Network
iScience(2024)
摘要
Identifying cancer genes is vital for cancer diagnosis and treatment. However, as the complexity of cancer occurrence and limited cancer genes knowledge, it is hard to identify cancer genes in accurate using only a few omics data and the overall performance of existing methods is being called for further improvement. Here, we introduce a two-stage gradual-learning strategy GLIMS to predict cancer genes using integrative features from multi-omics data. Firstly, it uses a semi-supervised hierarchical graph neural network to predict the initial candidate cancer genes by integrating multi-omics data and PPI network. Then, it uses an unsupervised approach to further optimize the initial prediction by integrating the co-splicing network in post-transcriptional regulation, which plays an important role in cancer development. Systematic experiments on multi-omics cancer data demonstrated that GLIMS outperforms the state-of-the-art methods for the identification of cancer genes and it could be a useful tool to help advance cancer analysis.
更多查看译文
关键词
Cancer genes,Multi-omics data,Alternative splicing,Data integration,Graph convolutional network
AI 理解论文
溯源树
样例
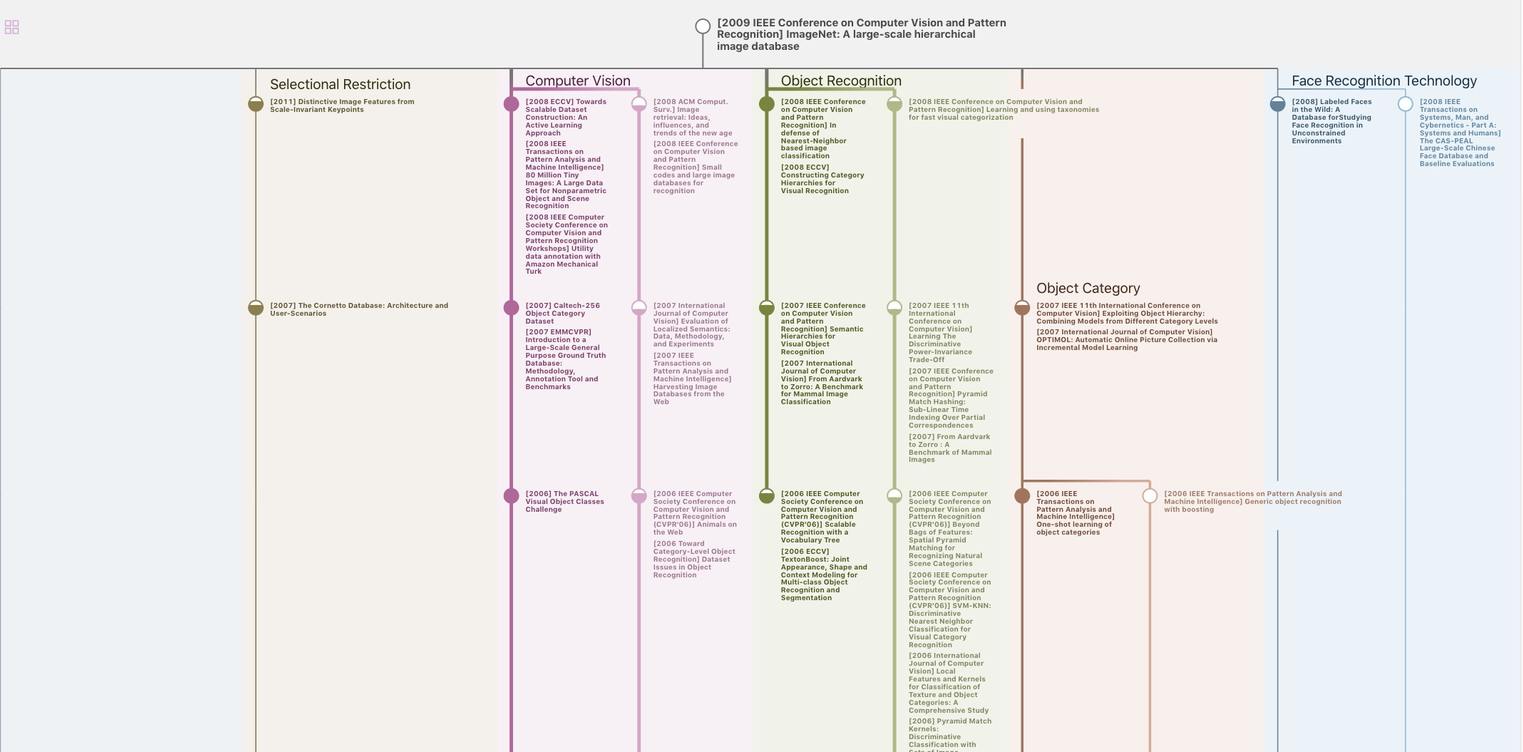
生成溯源树,研究论文发展脉络
Chat Paper
正在生成论文摘要