Event-triggered H∞ PI state estimation for delayed switched neural networks
Journal of Automation and Intelligence(2024)
摘要
On state estimation problems of switched neural networks, most existing results with an event-triggered scheme (ETS) not only ignore the estimator information, but also just employ a fixed triggering threshold, and the estimation error cannot be guaranteed to converge to zero. In addition, the state estimator of non-switched neural networks with integral and exponentially convergent terms cannot be used to improve the estimation performance of switched neural networks due to the difficulties caused by the nonsmoothness of the considered Lyapunov function at the switching instants. In this paper, we aim at overcoming such difficulties and filling in the gaps, by proposing a novel adaptive ETS (AETS) to design an event-based H∞ switched proportional–integral (PI) state estimator. A triggering-dependent exponential convergence term and an integral term are introduced into the switched PI state estimator. The relationship among the average dwell time, the AETS and the PI state estimator are established by the triggering-dependent exponential convergence term such that estimation error asymptotically converges to zero with H∞ performance level. It is shown that the convergence rate of the resultant error system can be adaptively adjusted according to triggering signals. Finally, the validity of the proposed theoretical results is verified through two illustrative examples.
更多查看译文
关键词
Switched neural networks,H∞ performance,PI state estimation,Event-triggered scheme
AI 理解论文
溯源树
样例
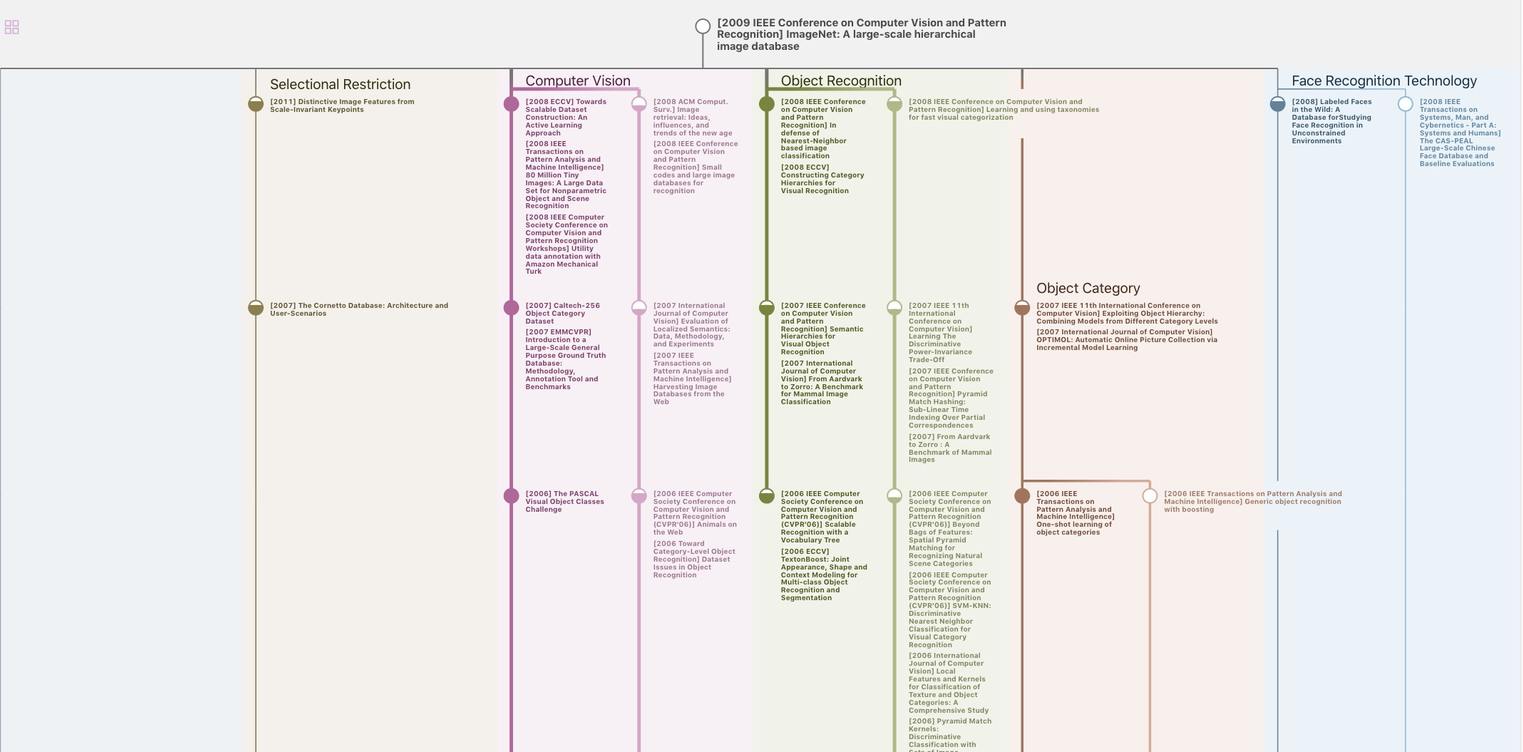
生成溯源树,研究论文发展脉络
Chat Paper
正在生成论文摘要