Multispectral detection of dietary fiber content in Chinese cabbage leaves across different growth periods
Food Chemistry(2024)
摘要
Multispectral imaging, combined with stoichiometric values, was used to construct a prediction model to measure changes in dietary fiber (DF) content in Chinese cabbage leaves across different growth periods. Based on all the spectral bands (365–970 nm) and characteristic spectral bands (430, 880, 590, 490, 690 nm), eight quantitative prediction models were established using four machine learning algorithms, namely random forest (RF), backpropagation neural network, radial basis function, and multiple linear regression. Finally, a quantitative prediction model of RF learning algorithm is constructed based on all spectral bands, which has good prediction accuracy and model robustness, prediction performance with R2 of 0.9023, root mean square error (RMSE) of 2.7182 g/100 g, residual predictive deviation (RPD) of 3.1220 > 3.0. In summary, this model efficiently detects changes in DF content across different growth periods of Chinese cabbage, which offers technical support for vegetable sorting and grading in the field.
更多查看译文
关键词
Chinese cabbage,Dietary fiber,Multispectral imaging,Predictive model,Random forest
AI 理解论文
溯源树
样例
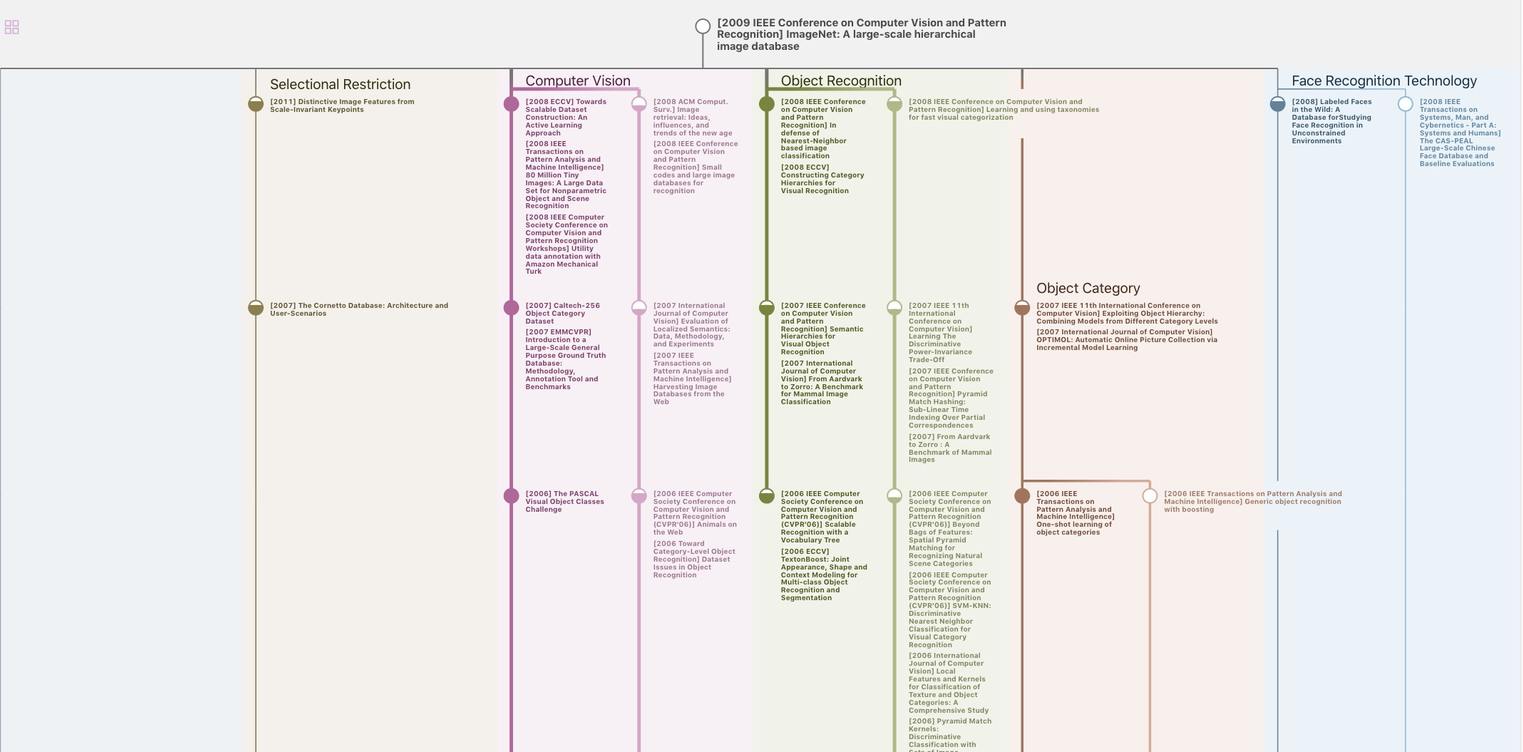
生成溯源树,研究论文发展脉络
Chat Paper
正在生成论文摘要