Pattern recognition frequency-based feature selection with multi-objective discrete evolution strategy for high dimensional medical datasets
Expert Systems with Applications(2024)
摘要
Feature selection has a prominent role in high-dimensional datasets to increase classification accuracy, decrease the learning algorithm computational time, and present the most informative features to decision-makers. This paper proposes a two-stage hybrid feature selection for high dimensional medical datasets: Maximum Pattern Recognition - Multi-objective Discrete Evolution Strategy (MPR-MDES). MPR is a rapid filter ranker that significantly outperforms existing frequency-based rankers in recognizing non-linear patterns, effectively eliminating a majority of non-informative features. Then, the wrapper Multi-objective Discrete Evolution Strategy(MDES) uses the remaining features and obtains sets of solutions which are automatically presented to decision-makers.The experiments conducted on large medical datasets demonstrate that MPR-MDES achieves considerable improvements compared to state-of-the-art methods, in terms of both classification accuracy and dimensionality reduction. In this sense, the proposal successfully performs when presenting informative feature sets to decision-makers. The implementation is available on https://github.com/KhaosResearch/MPR-MDES.
更多查看译文
关键词
Feature selection,High dimensional datasets,Filter,Wrapper,Multi-objective optimization
AI 理解论文
溯源树
样例
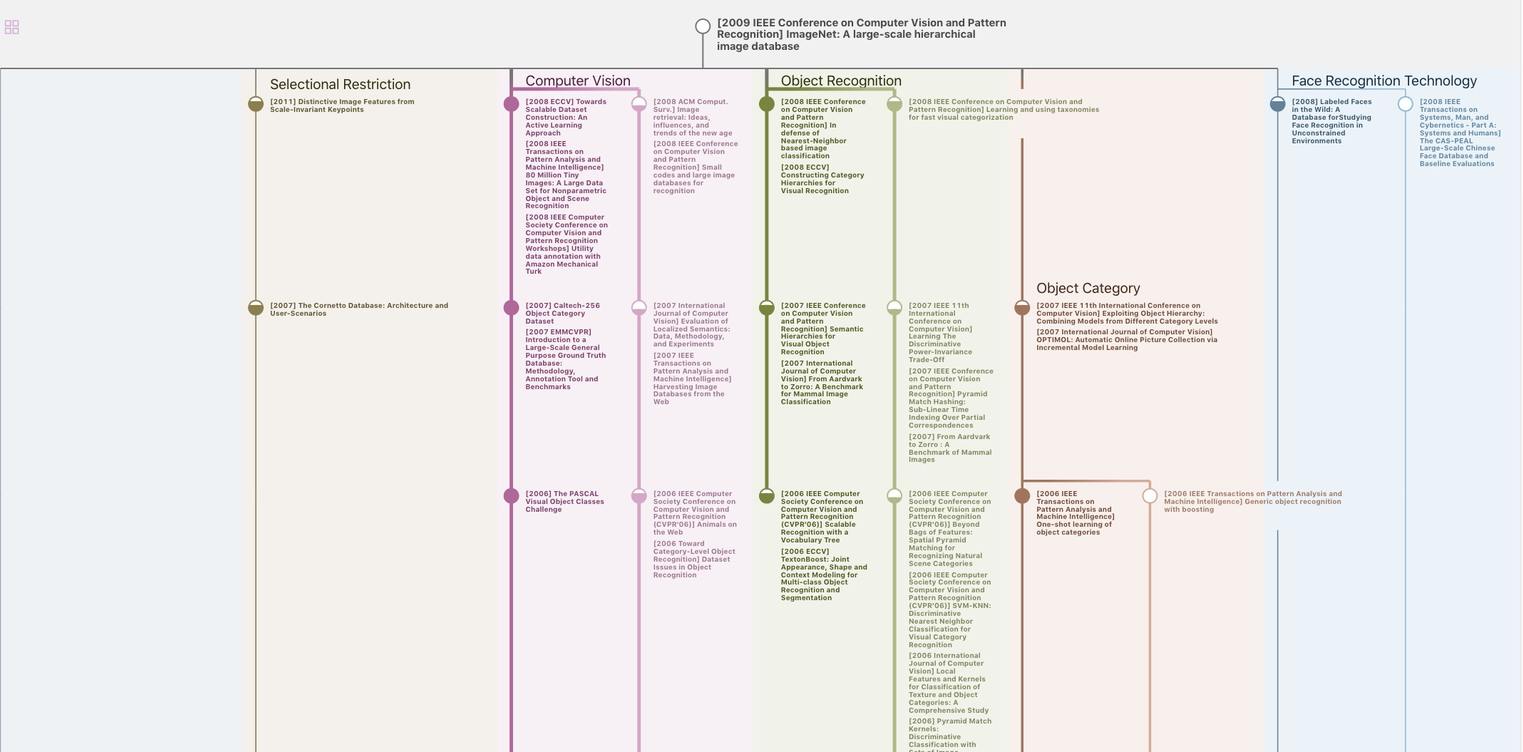
生成溯源树,研究论文发展脉络
Chat Paper
正在生成论文摘要