Bio-multisensory-inspired gate-attention coordination model for forecasting short-term significant wave height
ENERGY(2024)
摘要
Accurate forecasting of significant wave heights (SWH) plays an important role in the generation and conversion of ocean wave energy, being a promising clean energy source, while a challenging task since the SWH is produced by a complex natural process. Additionally, some deep forecasting models integrate parallel and recursive structures via unexplained ways to present intelligent fitting, leading to insufficient inheritance of hybrid advantages. Since biological multisensory systems automatically cope with complex time series, this paper explores a bio-multisensory-inspired gate-attention coordination network (BGC-Net) for forecasting SWH. Specifically, inspired by the multisensory cognitive process, the BGC-Net includes sensory capture, brain memory, and forecasting components, and their collaboration offers a clear multi-level handling process. In internal implementations, through mimicking that multiple senses independently capture external stimuli, the first component adopts several activation functions for suppressing data fluctuations and detecting the complex nonlinear and linear features. Through mimicking that the left and right hemispheres interact and collaborate electrical impulses, the second component integrates both gate-driven recursive and self-attention-driven parallel structures to extract multivariate and temporal dependencies at the feature level. Through mimicking that a higher cerebral cortex fuses extracted information for the final cognition, the third component proposes three feature fusion ways of concatenate, attention, and gated fusion, and takes the best one to generate SWH forecasts. In summary, the BGC-Net not only has interpretability at the biological level but also effectively integrates the advantages of parallel and recursive structures. Five experiments and six analyses all indicate that the BGC-Net exceeds 17 baselines, has root mean squared error (RMSE) average improvements with 22.30% and 19.22% for two datasets, and provides references to support SWH forecasting.
更多查看译文
关键词
Attention mechanism,Deep learning,Gated mechanism,Intelligent feature extraction,Significant wave height forecasting
AI 理解论文
溯源树
样例
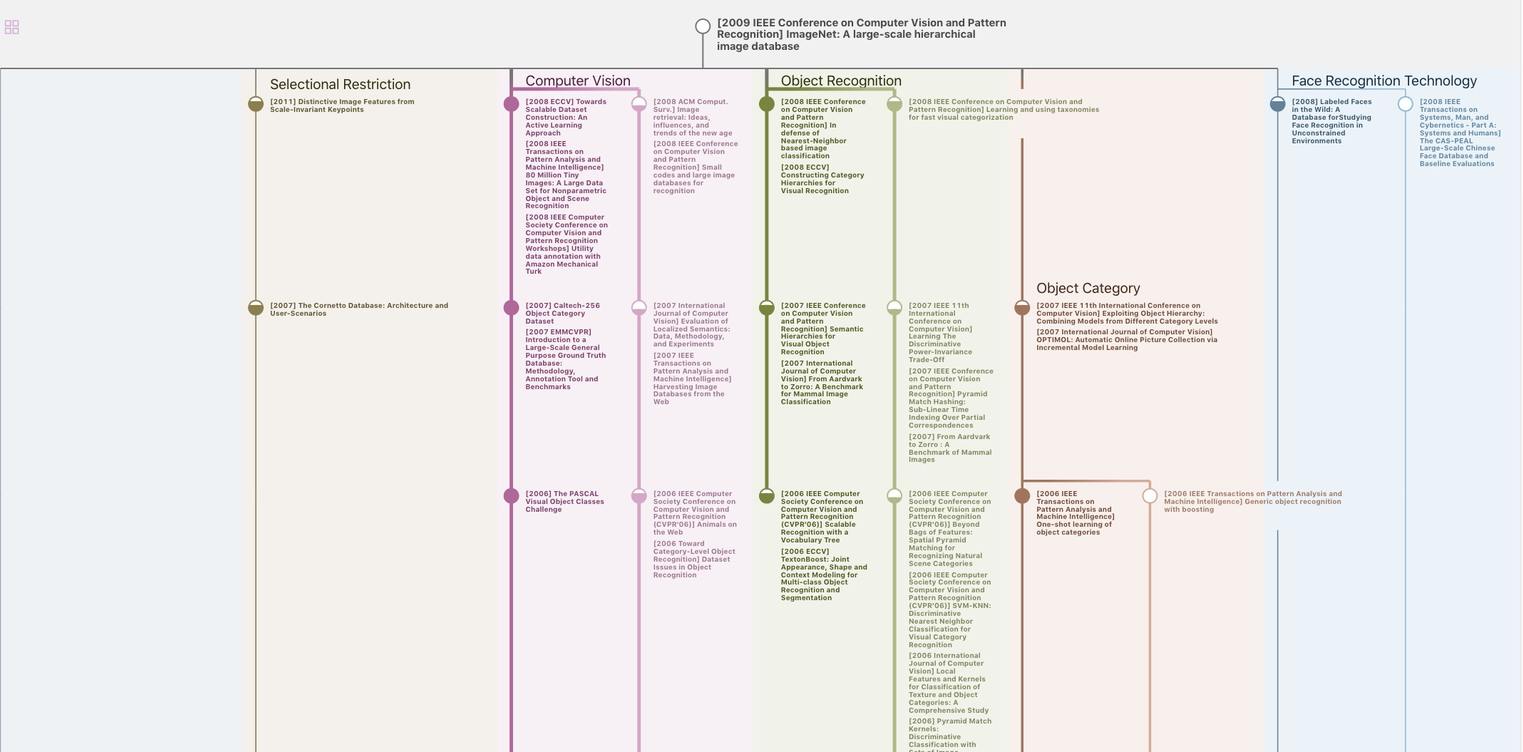
生成溯源树,研究论文发展脉络
Chat Paper
正在生成论文摘要