Channel based approach via faster dual prediction network for video anomaly detection
Multimedia Tools and Applications(2024)
摘要
Due to the fuzziness of anomaly definition and the complexity of scenes in real video data, video anomaly detection is still a challenging task. In this work, we explored a novel lightweight dual branch convolution neural architecture that can separate appearance-motion representations to capture spatial and temporal information, respectively, since abnormal events are usually different from normal cases in appearance or motion behavior. Considering the channel redundancy problem in the traditional neural network, and the feature information processed by different branches is different, the corresponding channel attenuation is carried out, which greatly improves the speed of anomaly detection while maintaining the performance of the model. In order to improve the utilization of key features, we exploited a Channel Squeeze and Excitation module and insert it into the encoder part of the network to focus on the channel correlation and adaptively recalibrate the characteristic response of the channel. The importance of each feature channel is automatically acquired through learning, and then according to the importance, the useful channels are promoted and the channels that are not useful for the current task are suppressed. In addition, in order to increase the reconstruction error of the motion encoder and consider the diversity of the normal patterns, we propose to use a memory module to augment the motion U-Net, where the items in the memory record the prototype mode of the normal data. The experiments on three benchmark datasets, UCSD Ped2, CHUK Avenue, and ShanghaiTech, demonstrate that our method achieves AUC scores of 96.3%, 87.4%, and 73.5%, respectively. The experimental speed reaches 55fps, showing a competitive performance relative to the current state of research.
更多查看译文
关键词
Video anomaly detection,Faster dual convolution network,Channel decrease,Channel squeeze-and-excitation module
AI 理解论文
溯源树
样例
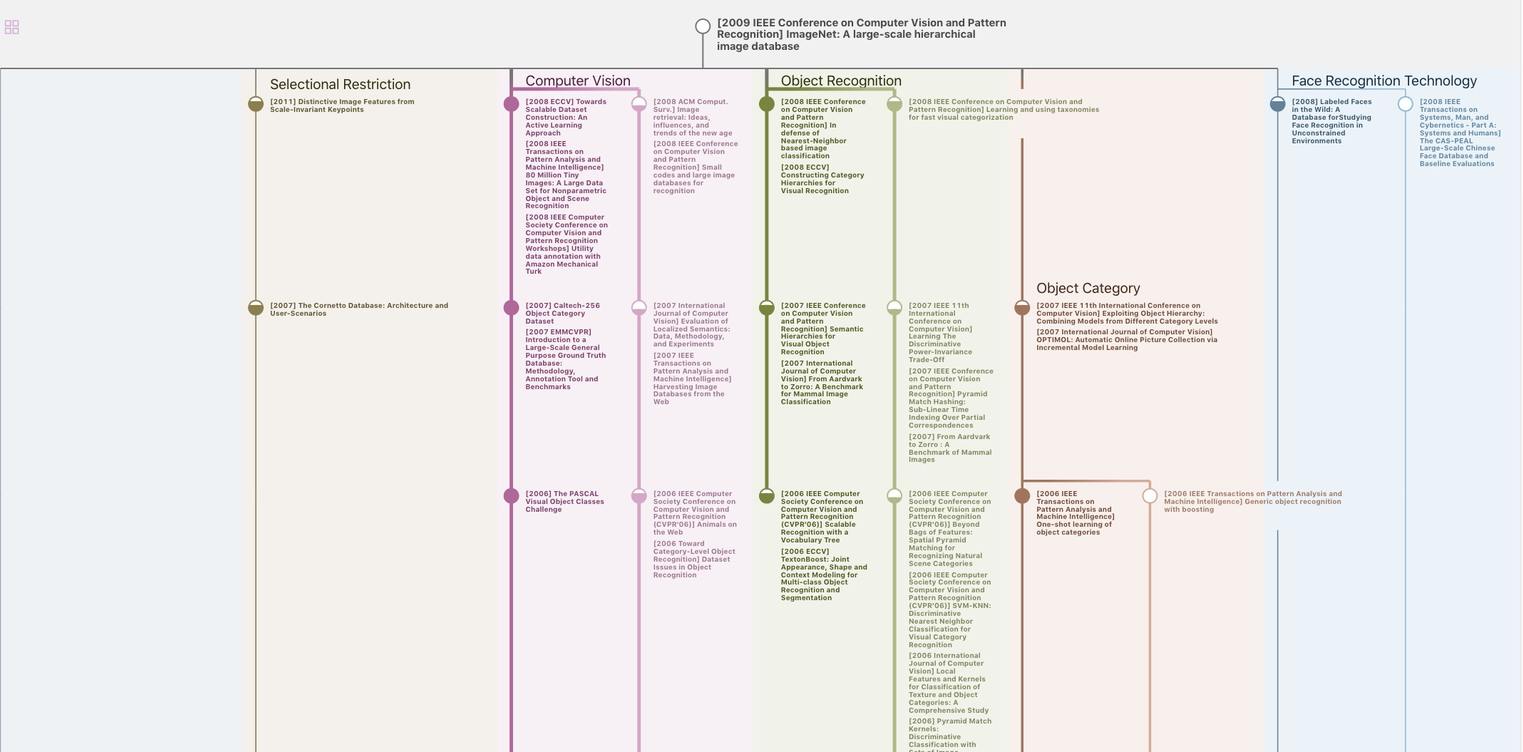
生成溯源树,研究论文发展脉络
Chat Paper
正在生成论文摘要