Automated entry of paper-based patient-reported outcomes: Applying deep learning to the Japanese orthopaedic association back pain evaluation questionnaire
COMPUTERS IN BIOLOGY AND MEDICINE(2024)
摘要
Background: Health -related patient -reported outcomes (HR -PROs) are crucial for assessing the quality of life among individuals experiencing low back pain. However, manual data entry from paper forms, while convenient for patients, imposes a considerable tallying burden on collectors. In this study, we developed a deep learning (DL) model capable of automatically reading these paper forms. Methods: We employed the Japanese Orthopaedic Association Back Pain Evaluation Questionnaire, a globally recognized assessment tool for low back pain. The questionnaire comprised 25 low back pain -related multiplechoice questions and three pain -related visual analog scales (VASs). We collected 1305 forms from an academic medical center as the training set, and 483 forms from a community medical center as the test set. The performance of our DL model for multiple-choice questions was evaluated using accuracy as a categorical classification task. The performance for VASs was evaluated using the correlation coefficient and absolute error as regression tasks. Result: In external validation, the mean accuracy of the categorical questions was 0.997. When outputs for categorical questions with low probability (threshold: 0.9996) were excluded, the accuracy reached 1.000 for the remaining 65 % of questions. Regarding the VASs, the average of the correlation coefficients was 0.989, with the mean absolute error being 0.25. Conclusion: Our DL model demonstrated remarkable accuracy and correlation coefficients when automatic reading paper -based HR -PROs during external validation.
更多查看译文
关键词
Back pain,JOABPEQ,Deep learning,Questionnaire,Convolutional neural network,Artificial intelligence
AI 理解论文
溯源树
样例
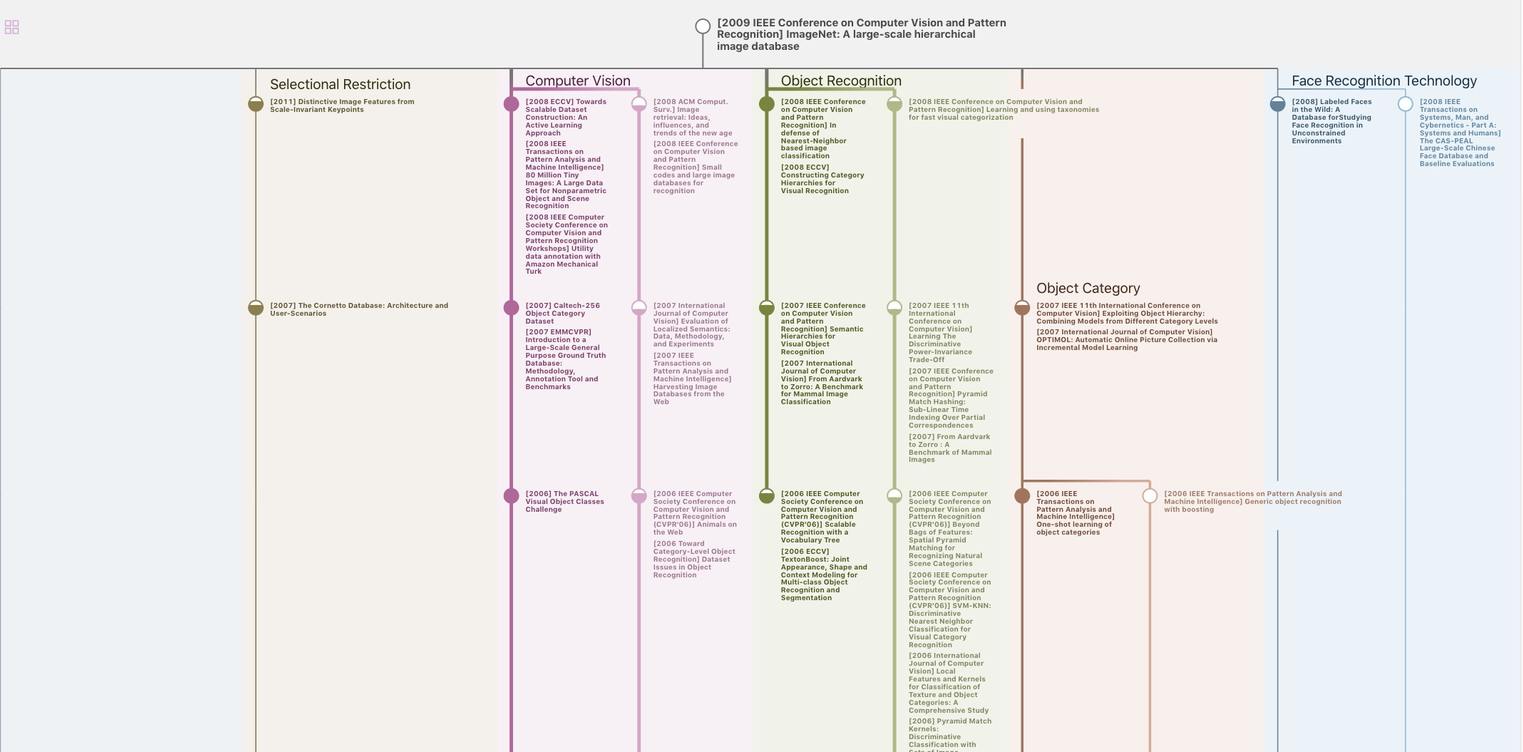
生成溯源树,研究论文发展脉络
Chat Paper
正在生成论文摘要