PECI-Net: Bolus segmentation from video fluoroscopic swallowing study images using preprocessing ensemble and cascaded inference
Computers in Biology and Medicine(2024)
摘要
Bolus segmentation is crucial for the automated detection of swallowing disorders in videofluoroscopic swallowing studies (VFSS). However, it is difficult for the model to accurately segment a bolus region in a VFSS image because VFSS images are translucent, have low contrast and unclear region boundaries, and lack color information. To overcome these challenges, we propose PECI-Net, a network architecture for VFSS image analysis that combines two novel techniques: the preprocessing ensemble network (PEN) and the cascaded inference network (CIN). PEN enhances the sharpness and contrast of the VFSS image by combining multiple preprocessing algorithms in a learnable way. CIN reduces ambiguity in bolus segmentation by using context from other regions through cascaded inference. Moreover, CIN prevents undesirable side effects from unreliably segmented regions by referring to the context in an asymmetric way. In experiments, PECI-Net exhibited higher performance than four recently developed baseline models, outperforming TernausNet, the best among the baseline models, by 4.54% and the widely used UNet by 10.83%. The results of the ablation studies confirm that CIN and PEN are effective in improving bolus segmentation performance.
更多查看译文
关键词
Medical image analysis,Video fluoroscopic swallowing study,Bolus segmentation,Cascaded inference,Preprocessing ensemble
AI 理解论文
溯源树
样例
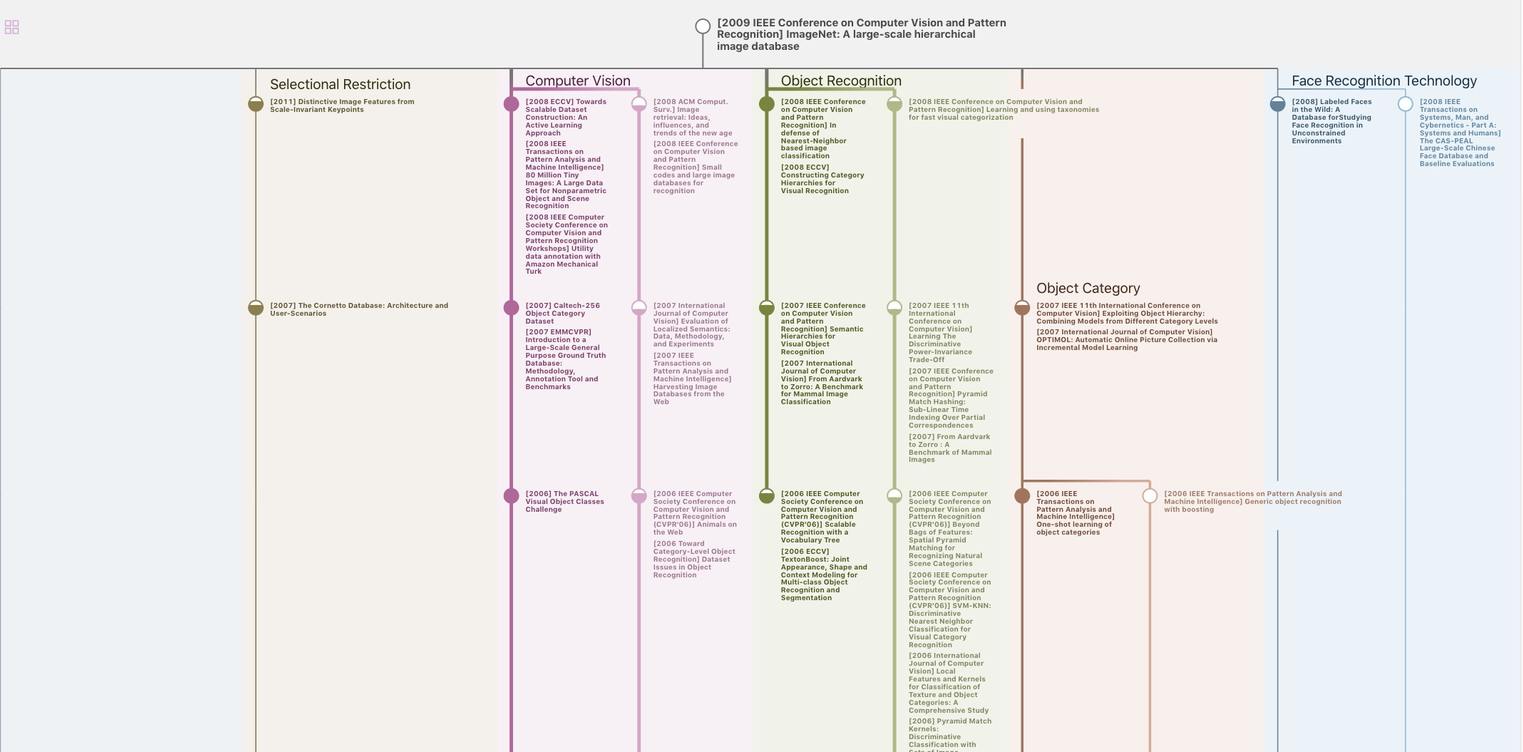
生成溯源树,研究论文发展脉络
Chat Paper
正在生成论文摘要